Darwin fundamentals
Darwin advanced
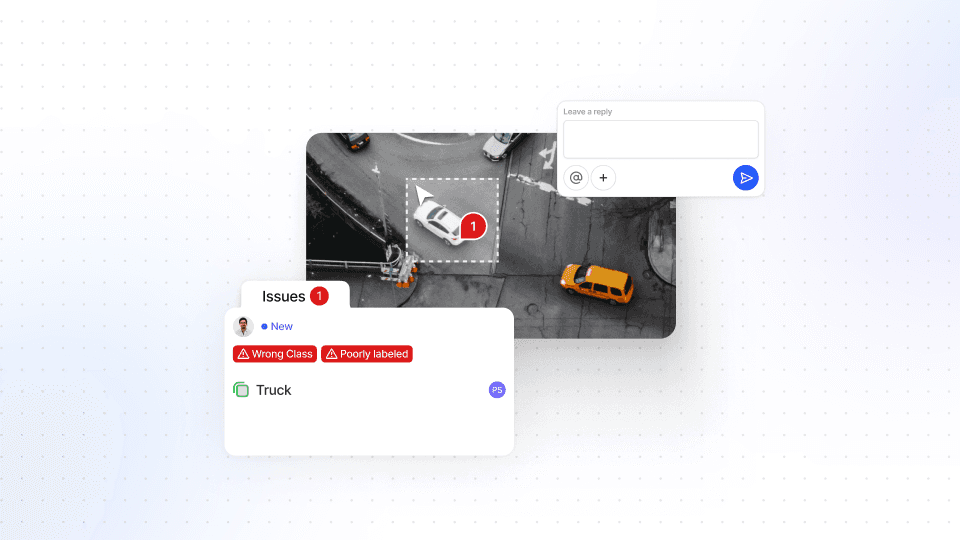
Play video
0:52
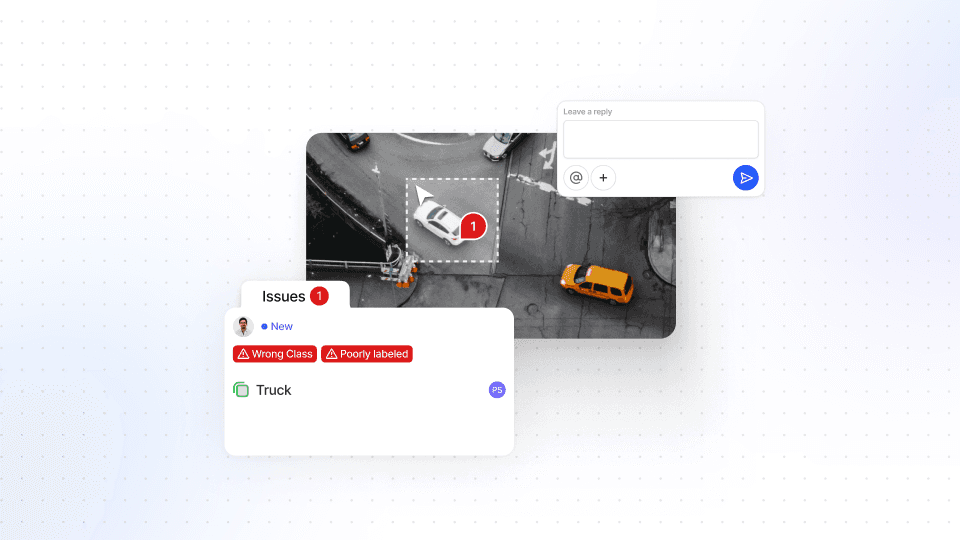
Play video
0:52
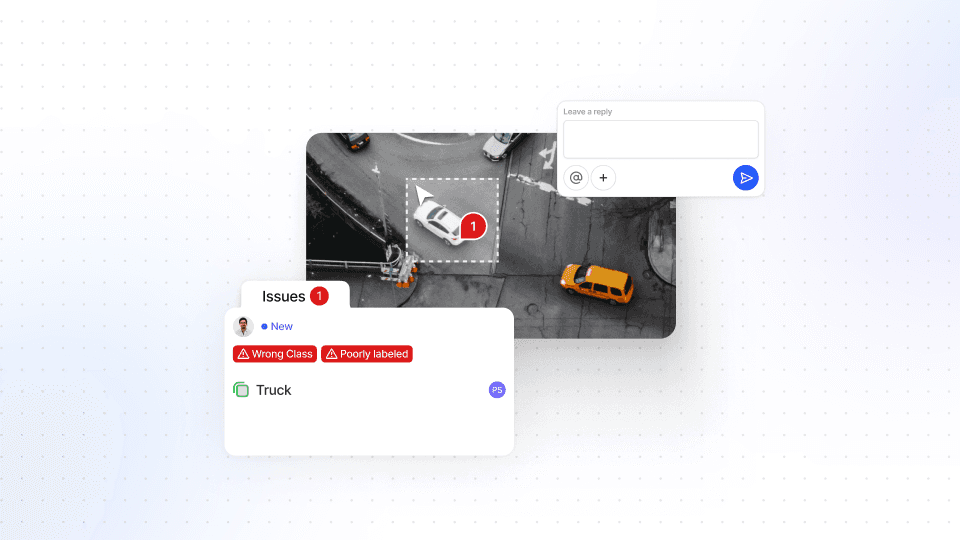
Play video
0:52
During this session, we will explore the process of adding comments in V7. When reviewing annotations, comments can be used to notify the author about any necessary corrections or the need to add additional labels. The author will receive a notification with a direct link to the image, allowing them to make the necessary adjustments.
Comments are particularly valuable when seeking expert input, such as in the case of radiological images or other scientific fields. You can engage professional team members, like doctors, to provide feedback on specific images, ensuring a more accurate and reliable annotation process.
The best part is that comments will remain visible until they are resolved, either by an admin or the author of the annotation. This ensures that no feedback goes unnoticed, and you can easily keep track of any pending actions.
By the end of this video, you will have a clear understanding of how to use comments in V7 Darwin to enhance communication and documentation within your datasets. Whether you are part of a team collaborating on image recognition projects or require expert input for specific images, comments can significantly improve your QA processes and enhance the accuracy of your machine learning workflows.
During this session, we will explore the process of adding comments in V7. When reviewing annotations, comments can be used to notify the author about any necessary corrections or the need to add additional labels. The author will receive a notification with a direct link to the image, allowing them to make the necessary adjustments.
Comments are particularly valuable when seeking expert input, such as in the case of radiological images or other scientific fields. You can engage professional team members, like doctors, to provide feedback on specific images, ensuring a more accurate and reliable annotation process.
The best part is that comments will remain visible until they are resolved, either by an admin or the author of the annotation. This ensures that no feedback goes unnoticed, and you can easily keep track of any pending actions.
By the end of this video, you will have a clear understanding of how to use comments in V7 Darwin to enhance communication and documentation within your datasets. Whether you are part of a team collaborating on image recognition projects or require expert input for specific images, comments can significantly improve your QA processes and enhance the accuracy of your machine learning workflows.
During this session, we will explore the process of adding comments in V7. When reviewing annotations, comments can be used to notify the author about any necessary corrections or the need to add additional labels. The author will receive a notification with a direct link to the image, allowing them to make the necessary adjustments.
Comments are particularly valuable when seeking expert input, such as in the case of radiological images or other scientific fields. You can engage professional team members, like doctors, to provide feedback on specific images, ensuring a more accurate and reliable annotation process.
The best part is that comments will remain visible until they are resolved, either by an admin or the author of the annotation. This ensures that no feedback goes unnoticed, and you can easily keep track of any pending actions.
By the end of this video, you will have a clear understanding of how to use comments in V7 Darwin to enhance communication and documentation within your datasets. Whether you are part of a team collaborating on image recognition projects or require expert input for specific images, comments can significantly improve your QA processes and enhance the accuracy of your machine learning workflows.
Up next
3:49
Watch video

Auto-Annotate Tool
How does the Auto-Annotate tool work? We tackle accurate polygon and pixel-wise annotation masks.
3:49
Watch video

Auto-Annotate Tool
How does the Auto-Annotate tool work? We tackle accurate polygon and pixel-wise annotation masks.
3:49
Watch video

Auto-Annotate Tool
How does the Auto-Annotate tool work? We tackle accurate polygon and pixel-wise annotation masks.
16:35
Watch video
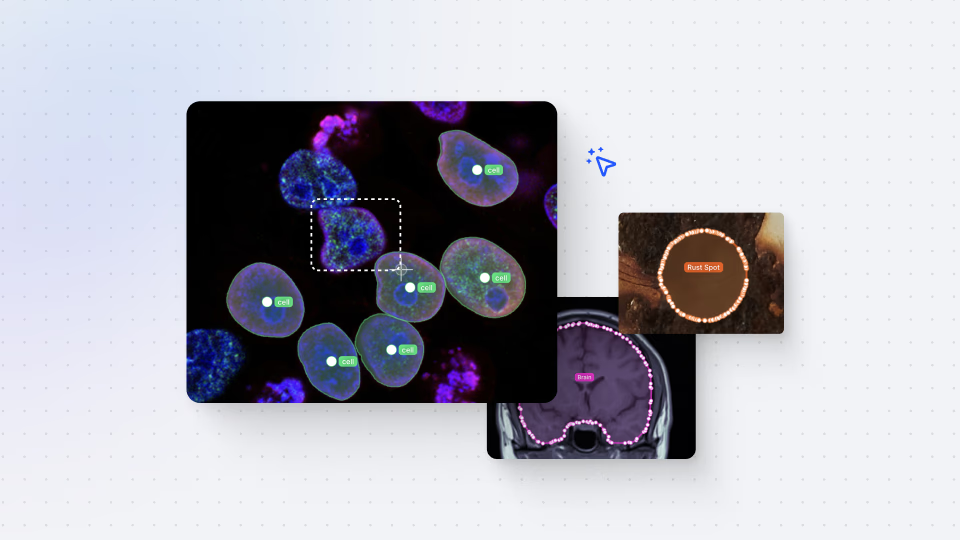
Annotations - Getting Started
We dive into image annotation and explain how you can get the most out of these impressive features.
16:35
Watch video
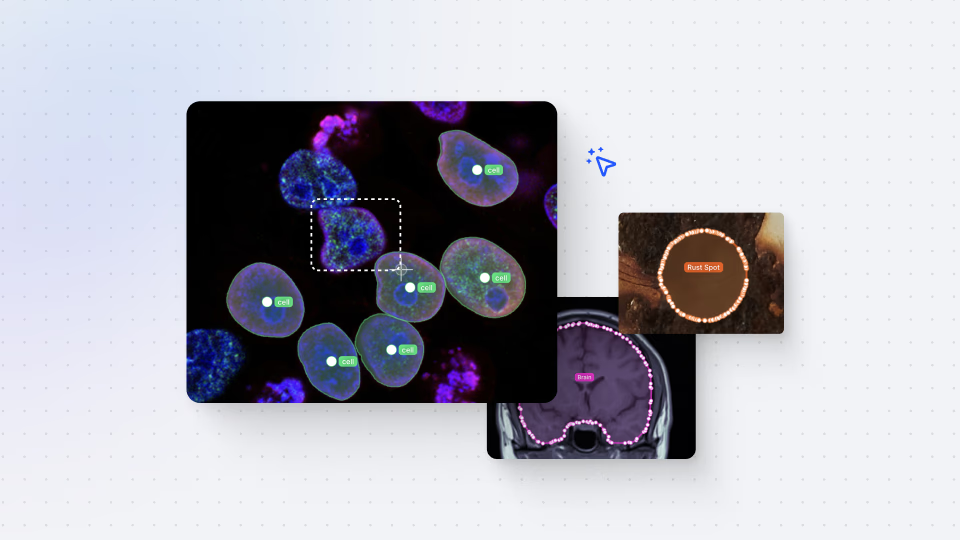
Annotations - Getting Started
We dive into image annotation and explain how you can get the most out of these impressive features.
16:35
Watch video
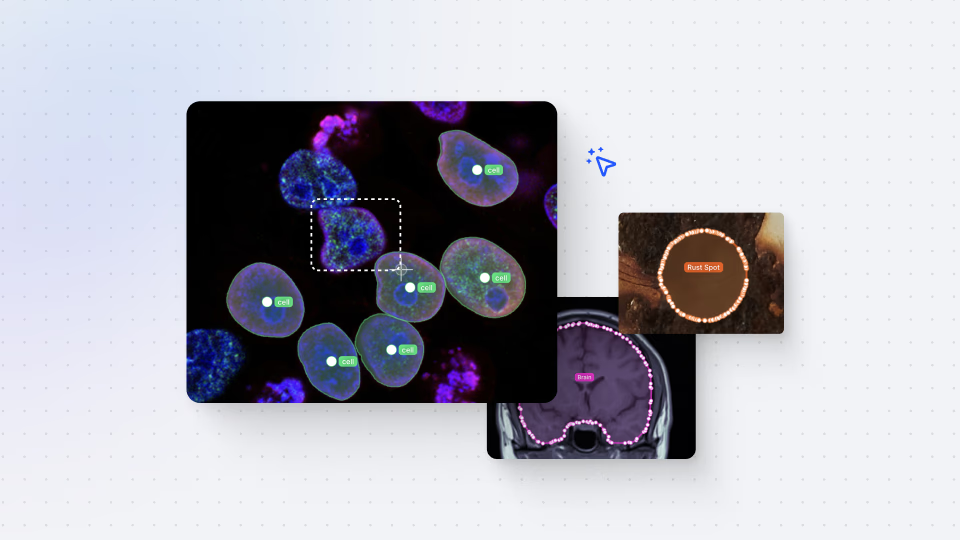
Annotations - Getting Started
We dive into image annotation and explain how you can get the most out of these impressive features.
5:56
Watch video

Auto-Annotate Tips & Tricks
Wield Auto-Annotate like the experts, with tips and tricks to accelerate your use of the tool.
5:56
Watch video

Auto-Annotate Tips & Tricks
Wield Auto-Annotate like the experts, with tips and tricks to accelerate your use of the tool.
5:56
Watch video

Auto-Annotate Tips & Tricks
Wield Auto-Annotate like the experts, with tips and tricks to accelerate your use of the tool.