Computer vision
Image Classification Explained [+V7 Tutorial]
20 min read
—
Jul 6, 2021
What is image classification and why is it important? Check out this beginner's guide to image recognition and build your own image classifier today.
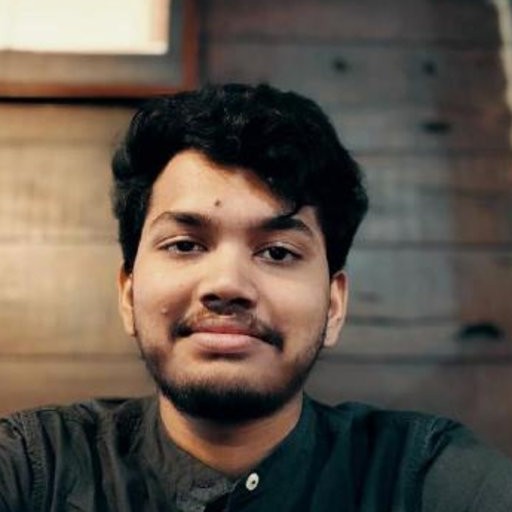
Guest Author
Image Classification is one of the most fundamental tasks in computer vision.
And for a reason—
It has revolutionized and propelled technological advancements in the most prominent fields, including the automobile industry, healthcare, manufacturing, and more.
So much more.
But—
How does Image Classification work, and what are its benefits and limitations?
Here’s what we’ll cover:
What is Image Classification?
How does Image Classification work?
Image Classification metrics
How to train an image classifier on V7?
And in case you want to skip the theory and roll up your sleeves to get some hands-on experience in training your own ML Models, check out:
What is Image Classification?
Image Classification (often referred to as Image Recognition) is the task of associating one (single-label classification) or more (multi-label classification) labels to a given image.
Here's how it looks like in practice when classifying different birds— images are tagged using V7.
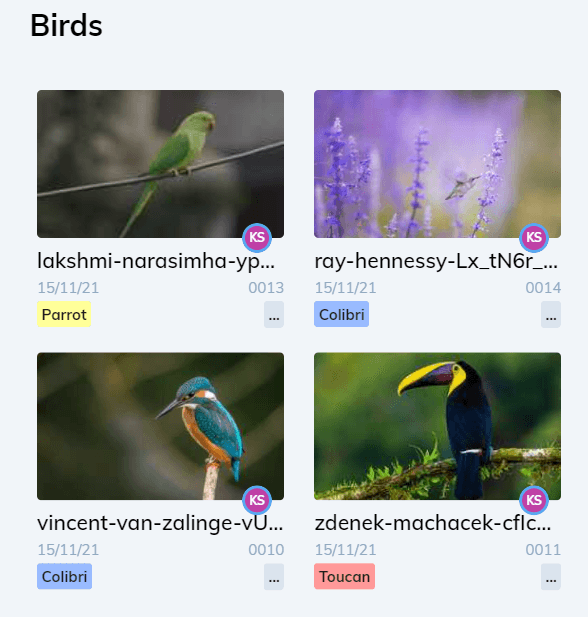
Image Classification using V7
Image Classification is a solid task to benchmark modern architectures and methodologies in the domain of computer vision.
Pro tip: Check out 27+ Most Popular Computer Vision Applications and Use Cases.
Now let's briefly discuss two types of Image Classification, depending on the complexity of the classification task at hand.
Single-label Classification
Single-label classification is the most common classification task in supervised Image Classification.
As the name suggests, a single label or annotation is present for each image in single-label classification. Therefore, the model outputs a single value or prediction for each image that it sees.
The output from the model is a vector with a length equal to the number of classes and value denoting the score that the image belongs to this class.
A Softmax activation function is employed to make sure the score sums up to one and the maximum of the scores is taken to form the model’s output.
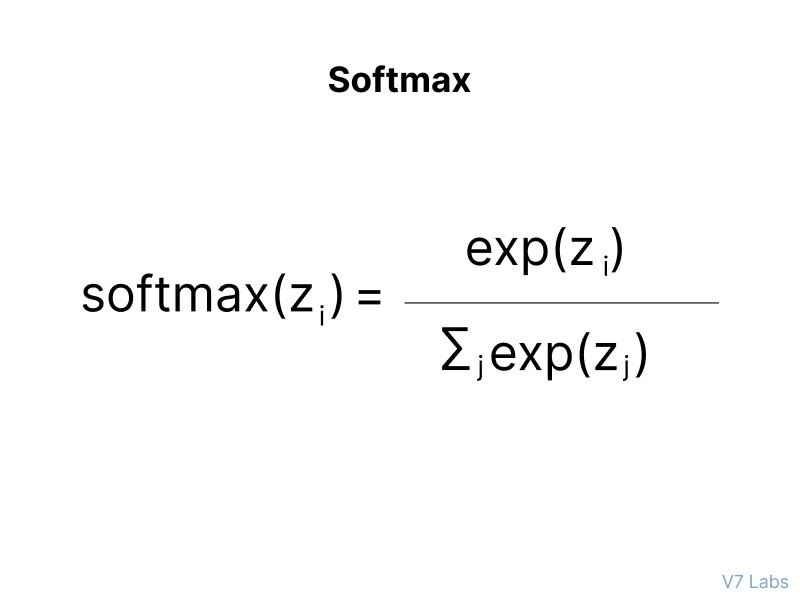
Softmax function mathematically
While the Softmax initially seems not to provide any value to the prediction as the maximum probable class does not change after applying it, it helps to bound the output between one and zero, helping gauge the model confidence from the Softmax score.
Pro tip: Learn more about activation functions by reading 12 Types of Neural Network Activation Functions: How to Choose?
Some examples of single-label classification datasets include MNIST, SVHN, ImageNet, and more.
Single-label classification can be of Multiclass classification type where there are more than two classes or binary classification, where the number of classes is restricted to only two.
Multi-label Classification
Multi-label classification is a classification task where each image can contain more than one label, and some images can contain all the labels simultaneously.
While this seems similar to single-label classification in some respect, the problem statement is more complex compared to single-label classification.
Multi-label classification tasks popularly exist in the medical imaging domain where a patient can have more than one disease to be diagnosed from visual data in the form of X-rays.
Furthermore, in natural surroundings, image labeling can also be framed as a multi-label classification problem, indicating objects present in the images.
Pro tip: Looking for quality medical data? Check out 21+ Best Healthcare Datasets for Computer Vision.
How does image classification work?
In this section, we'll discuss how image classification works and outline the steps you need to take to train your own image classifiers in V7.
Here's what we'll cover:
Data collection
Models
Training
Metrics
V7 image classifier tutorial
Ready? Let's get started!
Data collection
Due to the simplicity of the task, creating a high-quality dataset for image classification is quicker than other tasks, e.g., image segmentation.
Usually, a dataset is composed of images and a set of labels, and each image can have one or more labels. The most challenging part is to ensure the dataset is bias-free and balanced.
Pro tip: Have a look at our list of 65+ Best Free Datasets for Machine Learning.
Let me tell you a story that highlights the concept.
The story begins with the US Army trying to use Neural Networks to detect camouflaged enemy tanks.
Researchers trained a supervised network on 50 photos of camouflaged tanks and 50 photos of trees without tanks. They then validated their network on 200 more images they had captured for testing, only to find that the network successfully detected camouflaged tanks.
The researchers handed over the work to the Pentagon, which handed it back right after, complaining that the network did not work at all in their tests.
It turned out that the researchers were using a biased dataset, where the discriminant feature between data points was the weather, not the tank.
They had taken pictures of camouflaged tanks on cloudy days and pictures of trees on sunny days, leading to the network ignoring the tanks and discerning only between cloudy and sunny days.
The most used dataset is ImageNet with more than one million images annotated in 1000 categories.
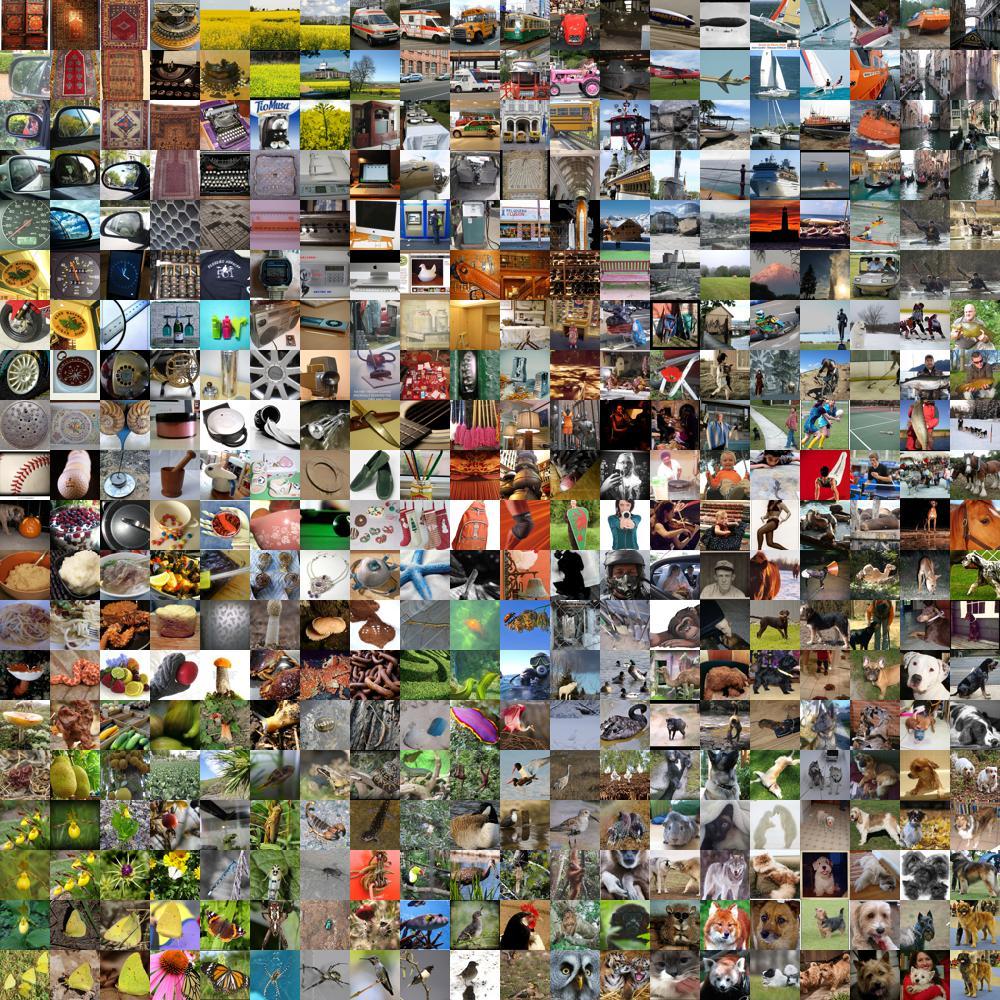
Source: some of ImageNet images
ImageNet is the de facto benchmark used to evaluate any modern computer vision architecture. In other words, we evaluate the quality of a model by looking at its error in this dataset.
There are tons of free and available datasets you can play with, have a look at 20+ open source computer vision datasets.
Models
In modern computer vision, the gold-standard model architecture to use is a convolutional neural network.
The convolution operation slides a matrix, called kernel, on input while performing pair-wise matrix multiplication and summing up the result. The following gif shows this process.
A note on naming: Filter and kernel are the same thing.
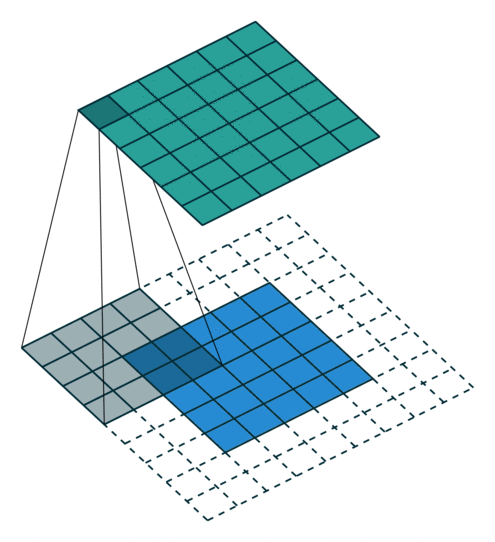
Source: The convolution operation
Let's see an example with numbers.
Assuming we have the following 3x3 kernel.
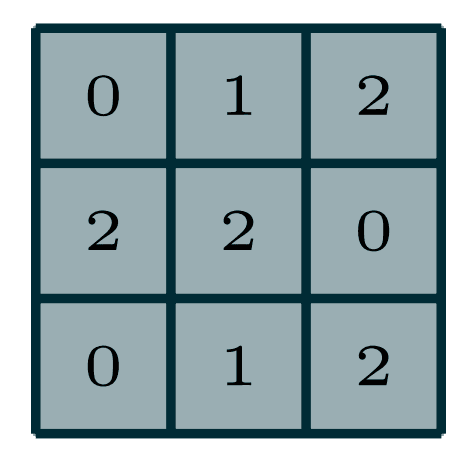
Source: 3x3 kernel
To apply the filter we have to imagine hovering it on the input matrix starting from top-right, multiplying each value with the kernel's corresponding value, and summing them up. Then we slide the kernel by a defined amount, called the stride, in this case, one, and we repeat the process.
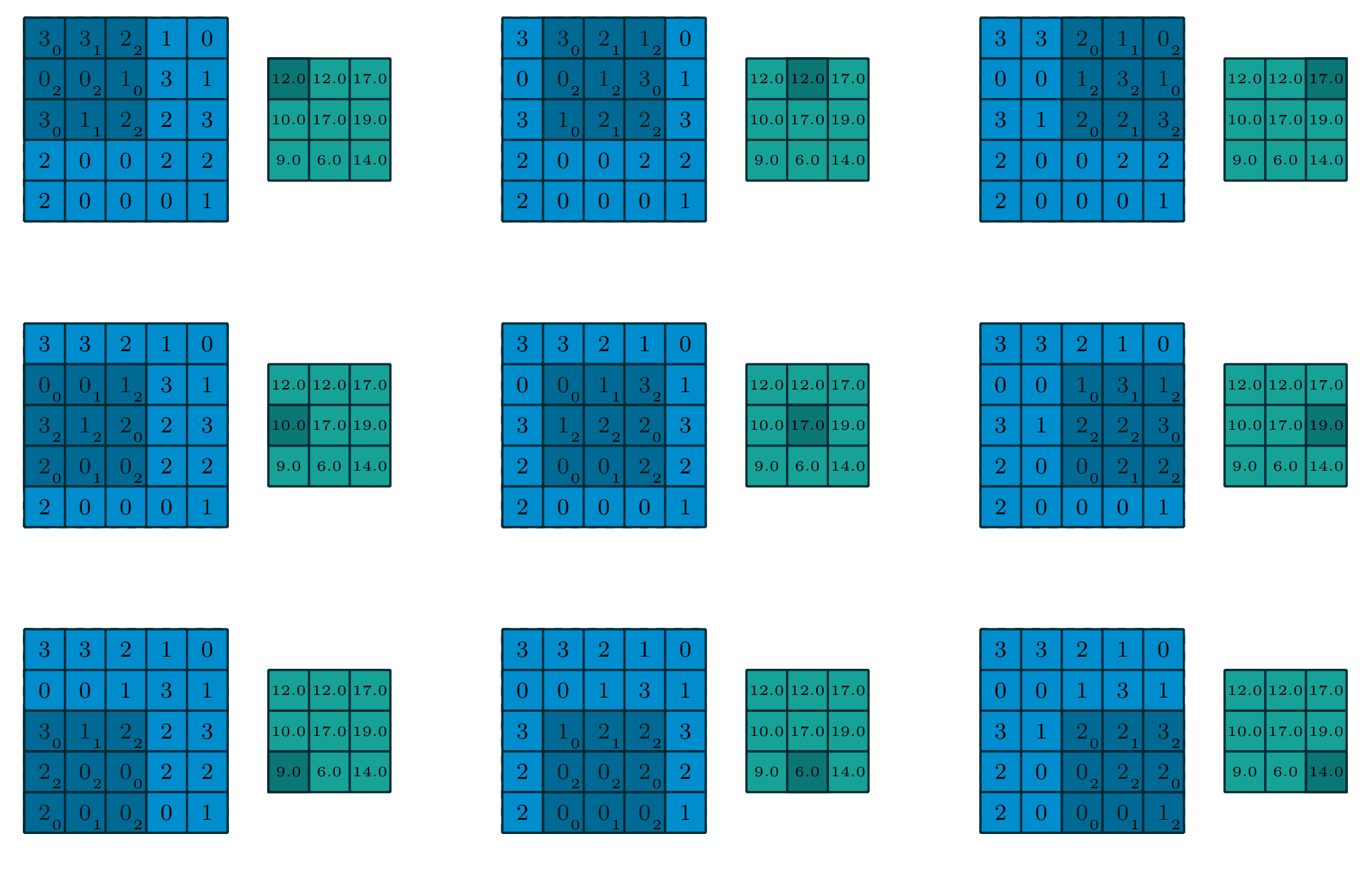
For example, the first step is to multiply and sump up:
3*0 + 3*1 + 2*2 + 0*2 + 0*1 + 3 *0 + 1*1 + 2*2
The number of kernels applied at the same time is referred to as features. Imagine we apply four 3x3 kernels to an image; the resulting matrix will have four features.
In practice, we randomly initialize the kernel's numbers, stack more of them together and update them during training using gradient descent.
One key aspect of the convolution operation is weights sharing; you may have noticed that the same kernel, the grey matrix, is reused in each step covering the whole image. This drastically reduces the number of weights needed, lowering computational cost.
Moreover, this introduces a positional bias since we teach the network to look at the input matrix by aggregating pixels close to each other. This helps because neighbor pixels are usually semantically connected.
Usually, in neural networks, multiple blocks of convolutional operation are stacked together to form a layer. Multiple layers are then composed together, like LEGOs, to form the final model. The number of layers is called depth; the more layers, the deeper the network.
To further reduce computation overhead, different kinds of poolings are applied between layers. A pool operation is a very simple thing. It takes a matrix and reduces it by aggregating its value. The most common one is max pooling where you define a window size and take the max inside it.

Source: Max Pooling
The final vector resulting by feeding a batch of images in this series of stacked convolution layers is then passed to a fully connected layer that outputs a vector with the classes.
Pro tip: Ready to train your models? Have a look at Mean Average Precision (mAP) Explained: Everything You Need to Know.
AlexNet
AlexNet was the first real convolutional neural network to achieve superhuman abilities. In 2012 it won the ImageNet large Scale Visual Recognition Channalnge by a 10% margin.
It is composed of stacked convolutional layers separated by max pooling
VGG
Very Deep Convolutional Networks **(**VGG) was the winner of 2014 ImageNet challenge. It is deeper than AlexNet pushing the depth up to 19 layers. It utilizes a smaller 3x3 kernel size filter, the standard today.
Inception V1-V3
Inception V1 was a family of networks developed by google. They have parallel connections in which the input flows in different convolutional blocks on different kernel sizes independently and then its output is aggregated. Inception V3 won the ImageNet competition in 2015
ResNet
ResNet introduced residual connection allowing the network to scale up to 150 layers. ResNet-50 is the most widely used backbone for computer vision tasks in the industry, due to its good accuracy and medium parameter count.
Multiple variants of ResNet have been proposed in the following years, some of the most interesting: Se-ResNet, ResNetXt, ResNeST and RegNet
Vision Transformers
Transformers are models widely used in Natural Language Processing (NLP). Recently, they have been adapter to image classification as well. While it is unclear if there is a real benefit over CNNs, the research is still undergoing.
Training
So, we got our data and our model, but how do we train it to successfully classify images?
Well, first of all, you need to know that there are two main categories of learning.
Supervised Learning
When we provide our model with training errors signals, e.g. you classify this image as a cat but it was a dog, we perform supervised learning. This is the most common scenario in which we have labeled datasets with image and class pairs.
Neural Networks are trained by minimizing a function, called loss, using gradient descend.
For single-label classification, we rely on the, defined as follows:

Don't be scared by the notation, m is the number of samples, y_i is the label, hat y_i is the prediction and ln is the natural logarithm.
Softmax
The model outputs a vector with a length equal to a number of classes, they are the classes' scores. Since they can be anything, we apply a special function called Softmax.
It makes these numbers sum up to one, so each individual item is bounded between 0 and 1. The more close to one the model thinks that class is the correct one.
The Softmax is defined as follows.

So we take the exponential of the output and we divide by the sum of the exponential of all the outputs. Let's take an example, imagine we have two classes and our output is:
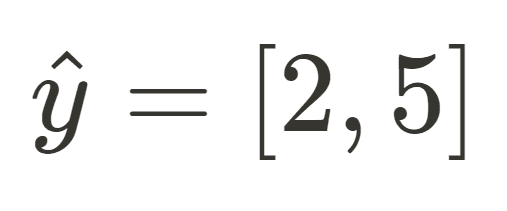
Clearly, the value for class 2, hat y_2, is the biggest one, but we really want them to be between 0 and 1 (you'll see it later). So let's apply the function, first let's find the denominator.

Then we take the exponential of each output and we divide by it.

The number sums up to one and they capture the correct score the model assigned previously. You may are wondering why we don't just normalize the output.
Here's the answer—
If we normalize the previous values we obtain [0.28, 0.71], while if with Softmax [0.05, 0.95]. Softmax pushes the values more away than vanilla normalization, effectively almost taking the maximum value, this is from where the name Softmax comes from.
Cross Entropy
Let's take an example if our target is $0$ but our model output $0.9$, so very close to $1$, the loss value would be.
Remember, in this case, the model outputs a vector with a length equal to a number of classes, they are the class's score. These numbers sum up to one, so each individual item is bounded between 0 and 1.
The more to close to one the model thinks that class is the correct one.

If you know about regression you may think that we can apply Mean Square Error and call if a day.
MSE doesn't penalize the model as much as BCE does. If we compute the gradient with respect to hat y_i.

While with MSE:

The gradient from BCE is 5 times more, thus the model will be penalized more.
Multi-Labels Classification
For multi-labels classification we want our outputs to be independent of each other since multi classes can exist at the same time. For example, if we have two classes at the same time our output could be [20, 21], we cannot apply Softmax since it will give more importance to one specific value, the biggest one.
In this case, we apply the Sigmoid function before BCE that maps between [-1,1], it is defined as follows.
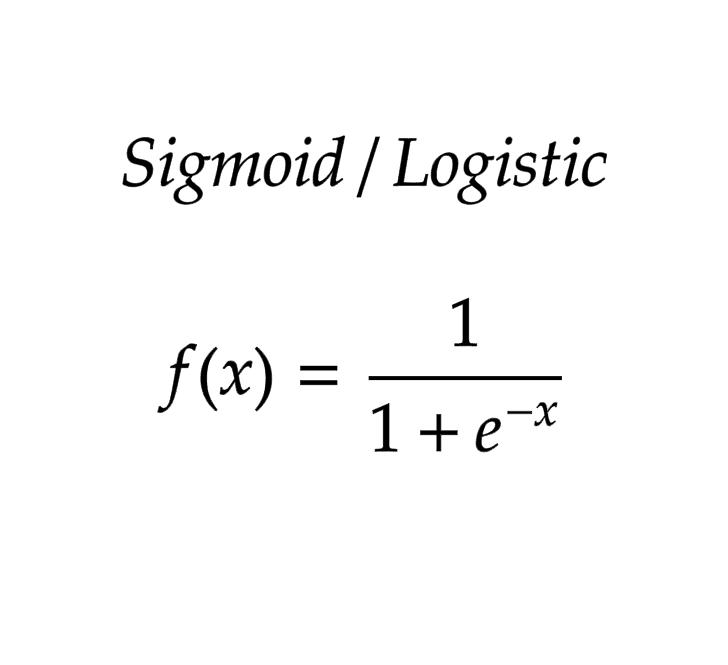
This will make sure that each individual value in our model outputs is bounded by the same range.
Unsupervised Learning
Here we don't have annotations, just a big collection of raw images and we let our model figure out what they are during training.
There are different approaches to solving this task—let's go through each one of them.
Contrastive learning
In contrastive learning, we take an input image, x, sample a “positive” image, x_pos, and a “negative” image, x_pos, in some way and try to train a scoring model such that score(x, x_pos) > score(x, x_neg)
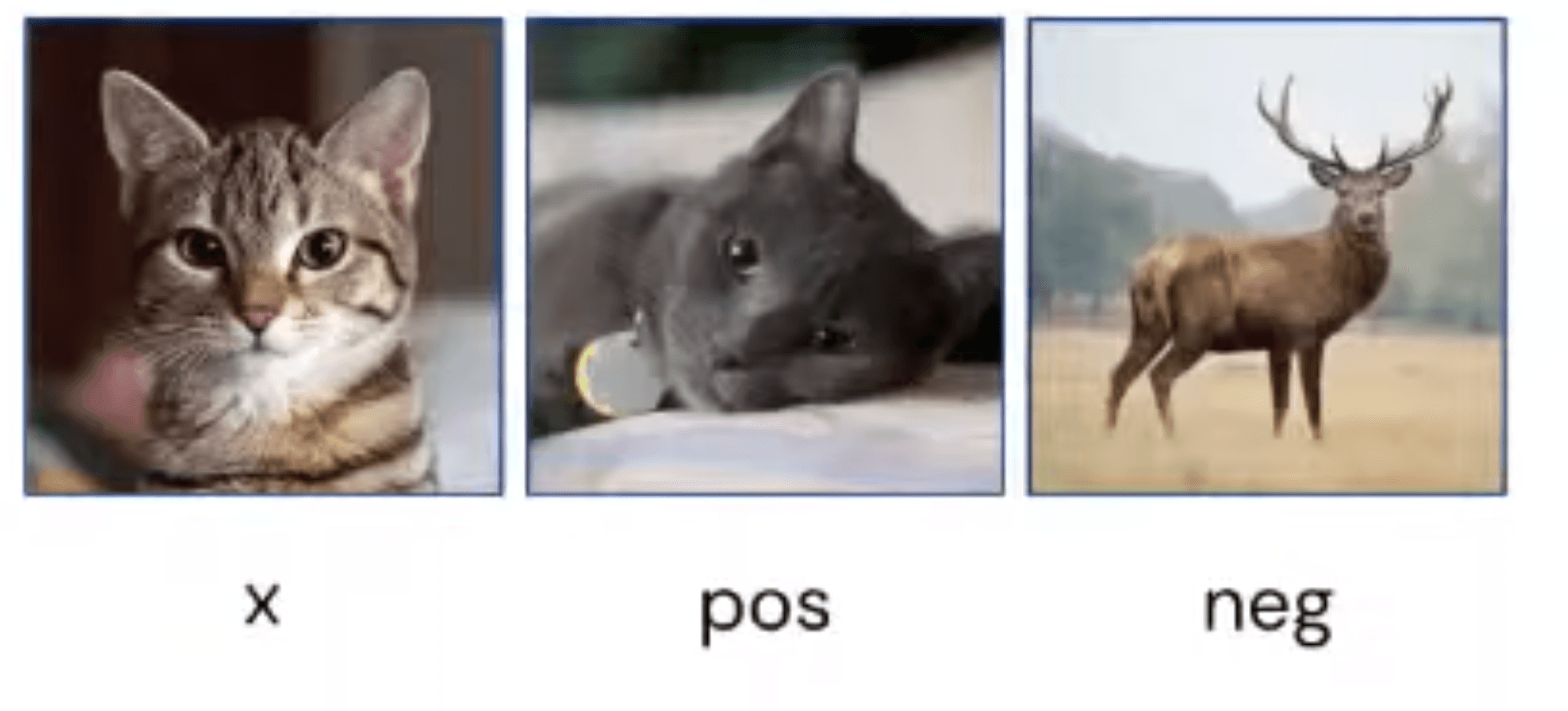
A sample with positive and negative images
We draw these samples randomly by enforcing that each time we have a different positive and negative example.
If we have labels, the negative sample must be from a different class, while the positive one is from the same one. When there are missing labels, one easy way to get the sample is to sample two parts of the same image for the positive and one part of another random image for the negative.
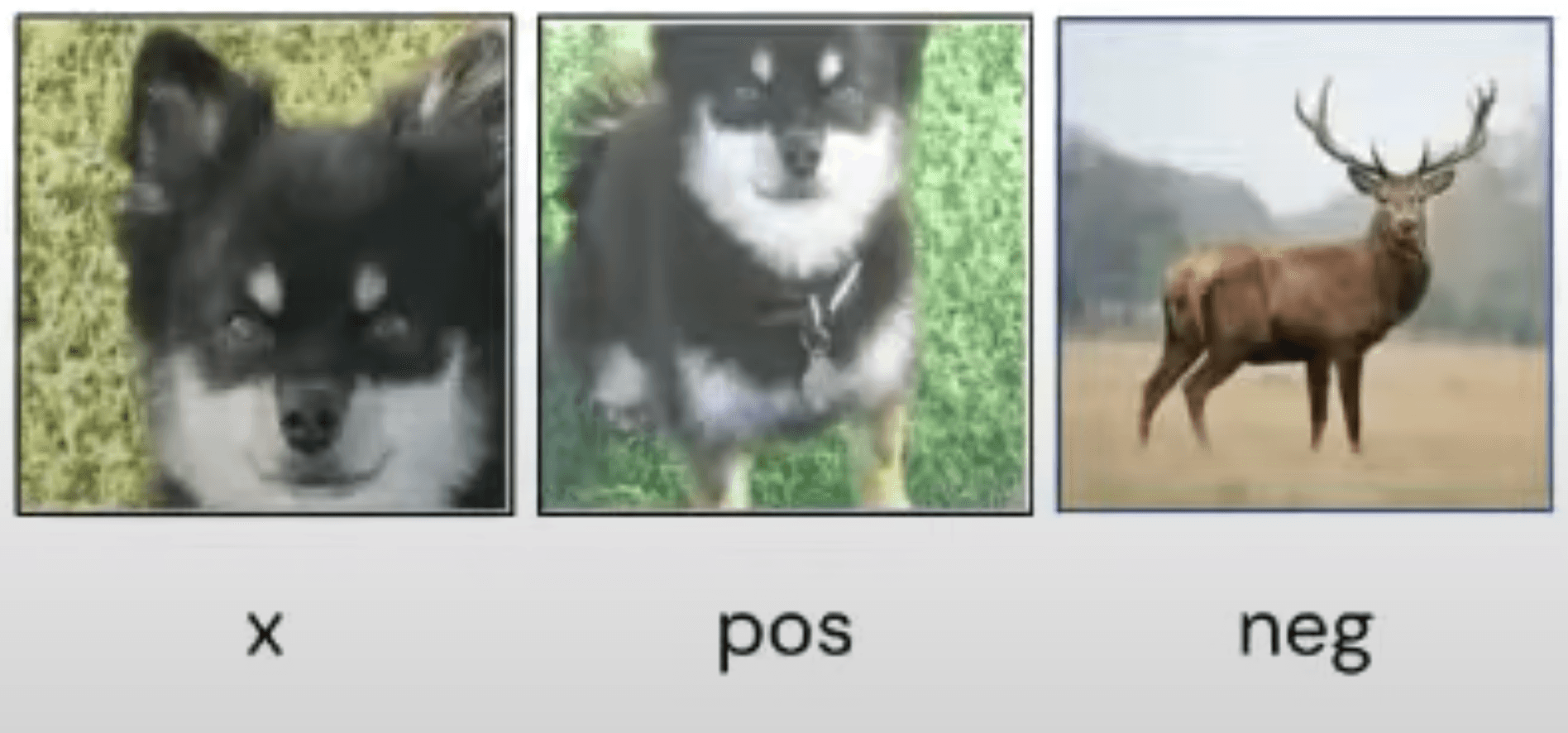
Sample of the same image negative and positive
Given a vector representation of an image, we try to push the two positive as close as possible and the negative them far away. One way is to learn a similarity metric, D.
D is the distance between these representations.
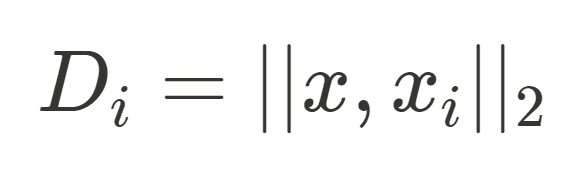
If positive, we want our loss L to push them close.

So we want x and x_pos to have a big dot product. On the other hand, if we have a negative sample we do the opposite.

The epsilon acts like a margin value.
Depending on how we decide to sample our example, we can have a pairwise loss, so with (x, x_pos) and (x, x_neg) or a triplet loss with (x, x_pos, x_neg) all together.

With Tripet losses, we first compute the score between x,_pos and x and x_neg, and then we use them in the loss.

Our variable m acts like a margin.
Another very common loss is InfoNCE in which here we can have multiple negative examples.
InfoNCE is the log-likelihood to predict the correct example from a given set of examples based on the score of the model.

The score function, s, is usually the inner product. The higher the more similar they are and vice versa.
One very successful method that uses contrastive learning is SimCLR in which you have a network f, usually a ResNet-50, and a linear projection, g.
The way it works is simple—
We sample a minibatch from the dataset and for each data point, x, we apply data augmentation and pass them through the network, f, and the linear projection obtained two representations. This effectively creates two positive pairs since we augmented the same images, then we apply a contrastive loss on all the augmented data points in the batch keeping track of the positive pairs.
Generative Models
Another idea is to tackle the unsupervised problem by using generative networks.
The idea is to give the networks reconstruction images tasks.
For example, we can crop some part of the image and train the network to fill in the gap.
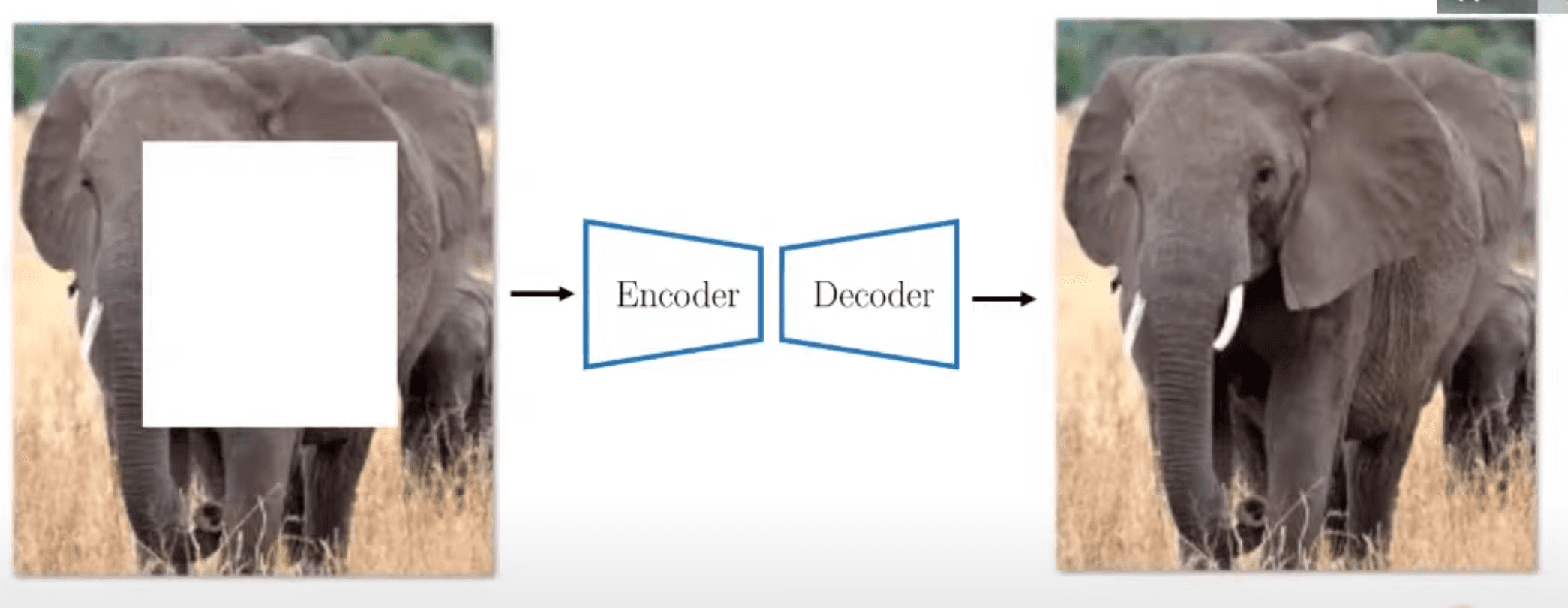
Generative model
This task requires the network to develop context-aware skills to reconstruct the missing input. Unfortunately, there are some limitations to this approach.
For example, there are way too many pixels details that the network has to learn that are not essentials, like the color.
Pro tip: To learn more, check out this Introduction to Autoencoders.
We can overcome these issues by using some ideas from contrastive learning, like applying an augmentation to create a pair of positive examples and training the network to reconstruct the permuted example.
However, there are some caveats here as well—
To make this work we also need a lot of negative examples, which is not ideal.
Teacher student models
One of the most efficient methodologies is the teacher-student approach.
The teacher and the student are two networks, usually the same. An input image, x, is first augmented in two different ways and then each of the new augmented images is passed to the teacher and student respectively.
They generate two features vector that we score with a reconstruction loss, like Mean Square Error or Cosine Similarity. Since they come from the same image, they are a positive pair and we want the result features from both models to be as similar as possible.
We don't need negative examples.
One approach that we want to highlight here is BYOL.
In BYOL, at each training step, after creating two augmented images from a data point and computing their score in the features space, the parameters of the student are updated using gradient descent while the teacher's ones are updated with the exponential moving average of the student's weights.
Even though it is a very straightforward procedure, it is highly effective.
Image Classification Metrics
Image Classification models have to be evaluated to determine how well they perform in comparison to other models.
Here are some well-known metrics used in Image Classification.
Precision
Precision is a metric that is defined for each class. Precision in a class tells us what proportion of data predicted by the ML model to belong to the class was actually part of the class in the validation data.
A simple formula can demonstrate this:

Where:
TP represents True Positives: The number of samples that were predicted to belong to a class and correctly belong to the class.
FP represents False Positives: The number of samples that were predicted to belong to a class while they do not belong to the class at all.
Recall
Recall similar to precision is defined for each class.
Recall tells us what proportion of the data from the validation set belonging to the class was identified correctly (as belonging to the class).
Recall can be represented as:

Where:
FN represents False Negatives: The number of samples that the model predicted as not belonging to a class while they actually belong to that particular class.
F1 Score
F1 Score helps us achieve a balance between precision and recall to get an average idea of how the model performs.
F1 score as a metric is calculated as follows.
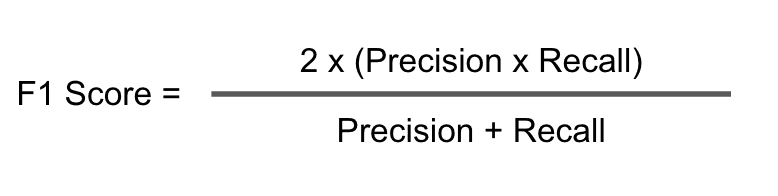
Precision and Recall scores largely depend on the problem the classification model is trying to address.
Recall is a critical metric, particularly in problems referring to the medical image analysis, like detection of pneumonia from chest X-rays, where false negatives cannot be present to prevent diagnosing a patient as healthy when they actually have the disease.
Precision is needed where the false positives have to be avoided, like email spam detection. If an important email is classified as spam, then a user would face significant issues.
Bonus 1: Video classification explained
Significantly differing from Image Classification, which only uses Image Processing algorithms and Convolutional Neural Networks to make a classification, Video Classification tasks make use of both image and temporal (relating to time) data.
Video Classification algorithms utilize the relation between the various frames in a continuous video to perform better than standard Image Classification algorithms on these tasks.
Neural Networks better suited to time series data like LSTMs (Long Short Term Memory) and RNNs (Recurrent Neural Networks) are used in conjunction with CNNs to perform video classification tasks to exploit the temporal relations between frames that simple CNN based methods would miss out on.
General Video classification datasets include sports datasets and datasets obtained from Youtube.
Bonus 2: 3D Classification explained
3D data classification is very similar to 2D image classification, with the primary difference being in the structure of the CNN and the nature of the movement of the sliding kernel.
Kernels in 3D data classification are also 3D and move along all three axes as compared to two axes linear motion in 2D CNNs.
CNNs are adept in capturing spatial data and hence adapt easily when the data is spaced out over three axes as compared to two.
Popular 3D classification datasets are found in the medical domain, with brain data obtained from MRI scans and structural data of macromolecules obtained from Cryo-Electron Microscopy.
How to train an image classifier on V7?
Finally, let us walk you through the process of training image classification models on V7. No matter your experience level, V7 makes it extremely easy to build reliable image classifiers within minutes.
To begin, you need to sign up for a 14-day free trial to get access to our tool. And once you are in, here's what comes next.
1. Upload the data
To start building an image classifier on V7, you need to upload image data that you want to work with. Go to the dataset tab on the left-hand side of the screen and click “New Dataset”.
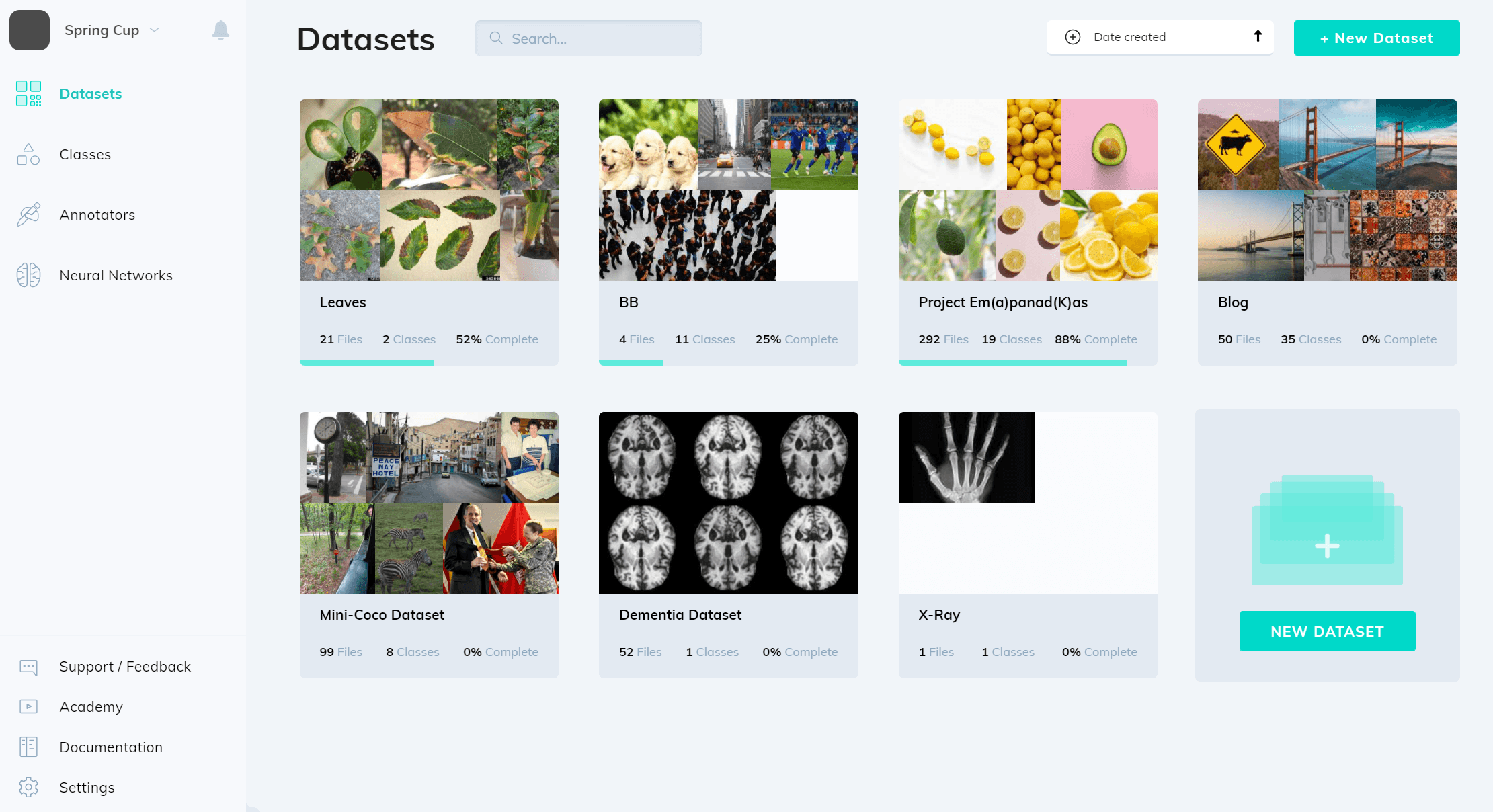
V7 also allows you to upload your data via API and CLI SDK.
Pro tip: For more information, make sure to check out V7 Darwin Documentation.
2. Create new annotation classes (tags)
You can do it both ways—either from the image level or going to the "Classes tab to create your classes in bulk.
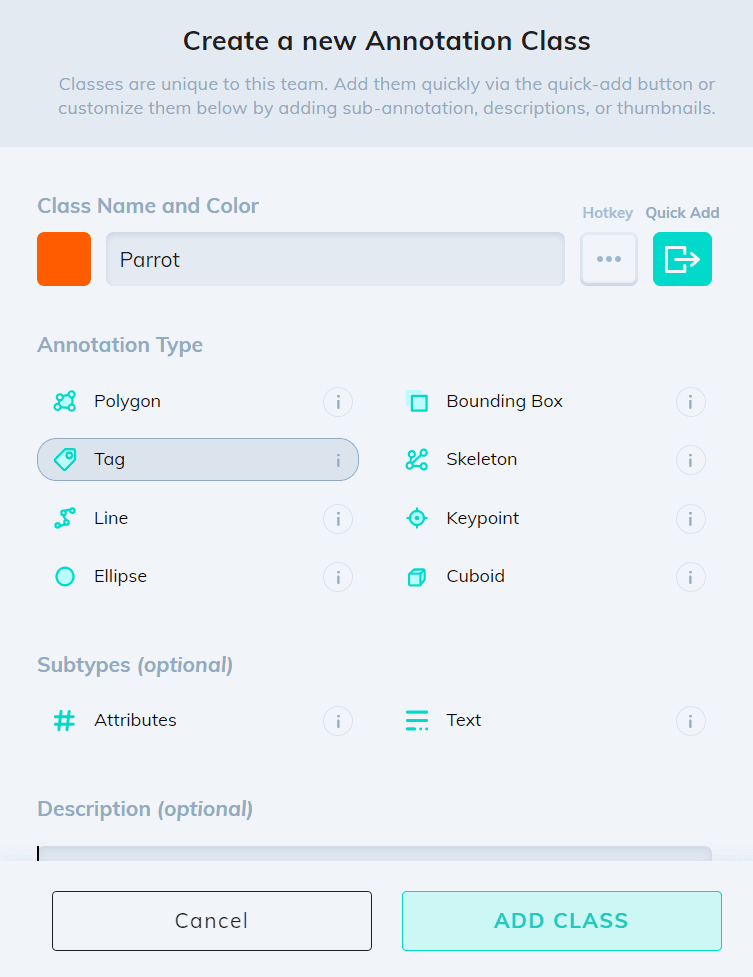
New annotation class creation on V7
Apart from choosing your annotation type—in this case a tag, you can also add subannotations, such as Attributes or Text.
3. Annotate your data (image tagging)
Once you've created your classes and organized your dataset, you can start labeling your data by adding tags to your images. As mentioned above, you can either add your tags to each image individually or tag them in bulk from the dataset level.
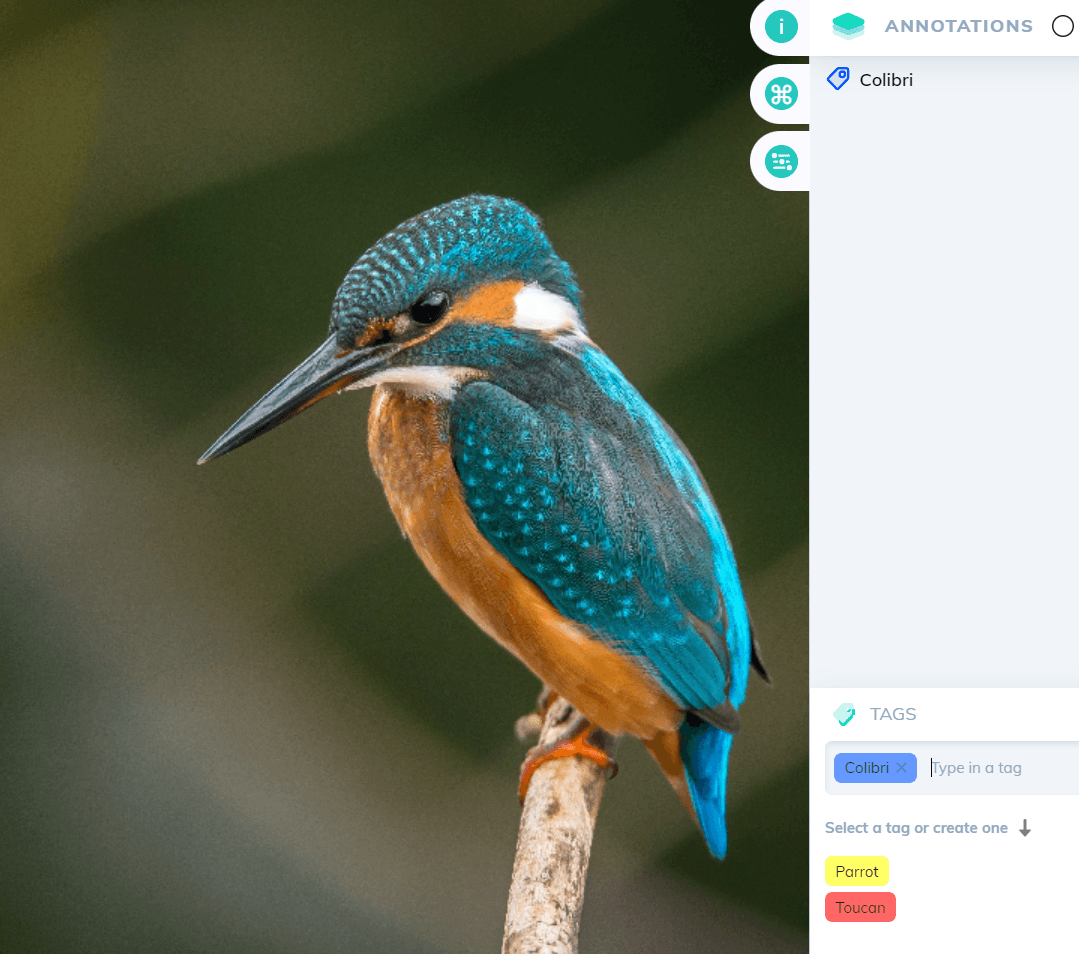
Tagging a colibri on V7
4. Train your Image Classification model
Once you've annotated enough instances, you are ready to train your first image classifier using V7!
Simply head over to the "Neural Networks" tab, add new model, choose "Image Classification", pick your dataset and start training.
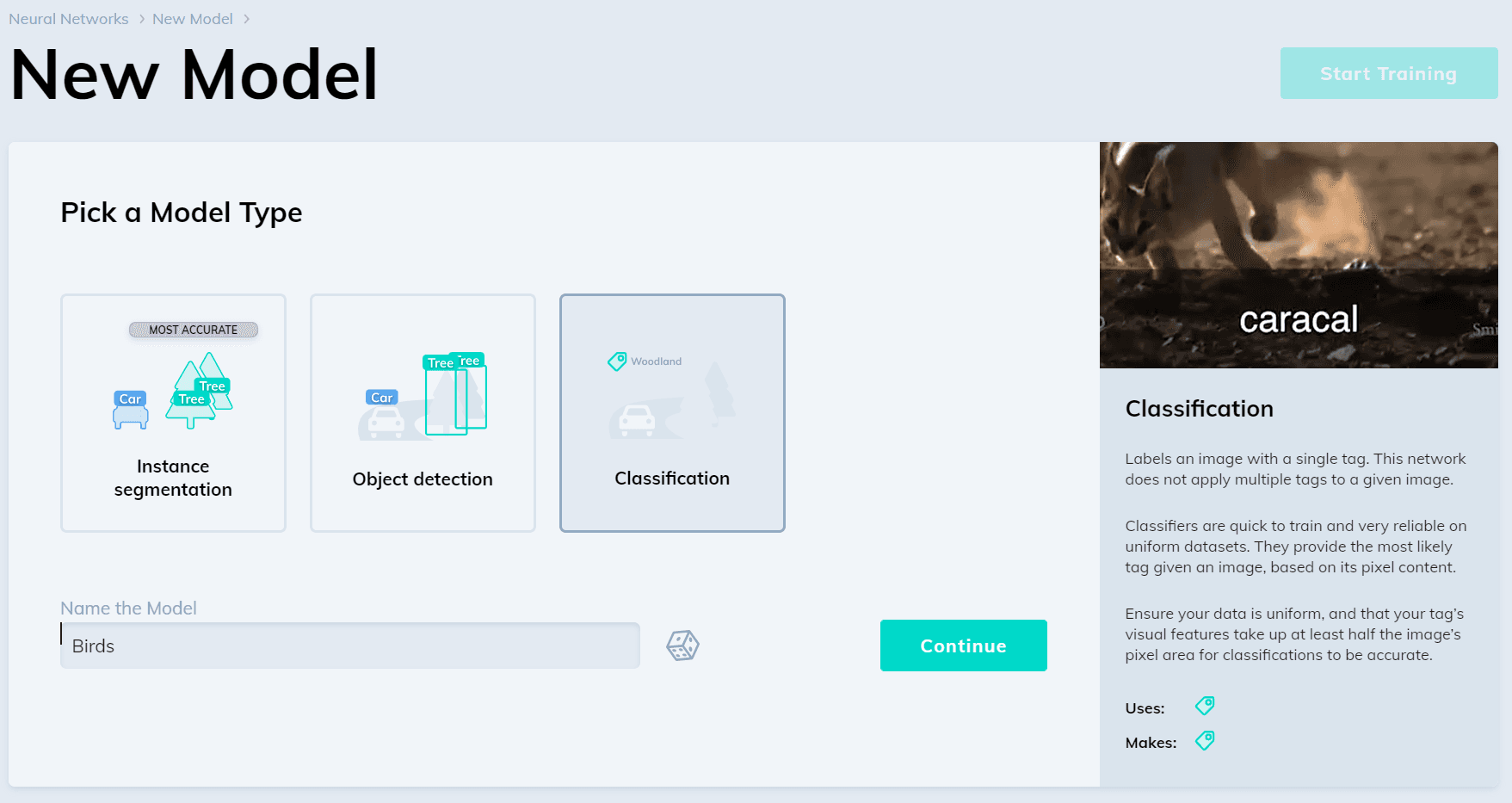
Training Image Classification model on V7
And... don'rt forget that V7 also allows you to train object detection, instance segmentation and OCR models ;-)
Now, go ahead, and start building your AImodels! We are excited to see your computer vision project ideas.
Image classification in a nutshell: Key takeaways
Finally, let's recap everything you've learned today about image classification:
Image classification is a subdomain of computer vision dealing with categorizing and labeling groups of pixels or vectors within an image using a collection of predefined tags or categories that an algorithm has been trained on.
We can distinguish between supervised and unsupervised classification.
In supervised classification, the classification algorithm is trained on a set of images along with their corresponding labels.
In unsupervised classification, the algorithm uses only raw data for training.
To build reliable image classifiers you need enough diverse datasets with accurately labeled data.
Image classification with CNN works by sliding a kernel or a filter across the input image to capture relevant details in the form of features.
The most important image classification metrics include Precision, Recall, and F1 Score.
Read more:
Annotating With Bounding Boxes: Quality Best Practices
YOLO: Real-Time Object Detection Explained
The Beginner’s Guide to Semantic Segmentation
A Comprehensive Guide to Human Pose Estimation
Hmrishav Bandyopadhyay studies Electronics and Telecommunication Engineering at Jadavpur University. He previously worked as a researcher at the University of California, Irvine, and Carnegie Mellon Univeristy. His deep learning research revolves around unsupervised image de-warping and segmentation.