Annotation suite
Annotation suite
Annotation suite
Data labeling.
Lightning-fast, pixel-perfect.
Data labeling.
Lightning-fast, pixel-perfect.
Customers
Customers
Build breakthrough AI models.
Join leading AI research labs and Fortune 100s.
Customers
Use cases
Use cases
Use cases
Create high-quality training data.
V7 supports all your labeling needs.
Images
Videos
DICOM & NIfTI
Microscopy
RLHF
Speed up complex annotation tasks with AI-assisted labeling and custom computer vision models. Generate semantic pixel masks and segment irregular shapes with a single click. Use keypoints, brushes, polylines, and more.
Images
Videos
DICOM & NIfTI
Microscopy
RLHF
Speed up complex annotation tasks with AI-assisted labeling and custom computer vision models. Generate semantic pixel masks and segment irregular shapes with a single click. Use keypoints, brushes, polylines, and more.
Annotation suite
Annotation suite
Annotation suite
Automate video annotations 10x faster without errors.
Create high-quality training data with AI-assisted labeling.
Automate video annotations 10x faster without errors.
Create high-quality training data with AI-assisted labeling.
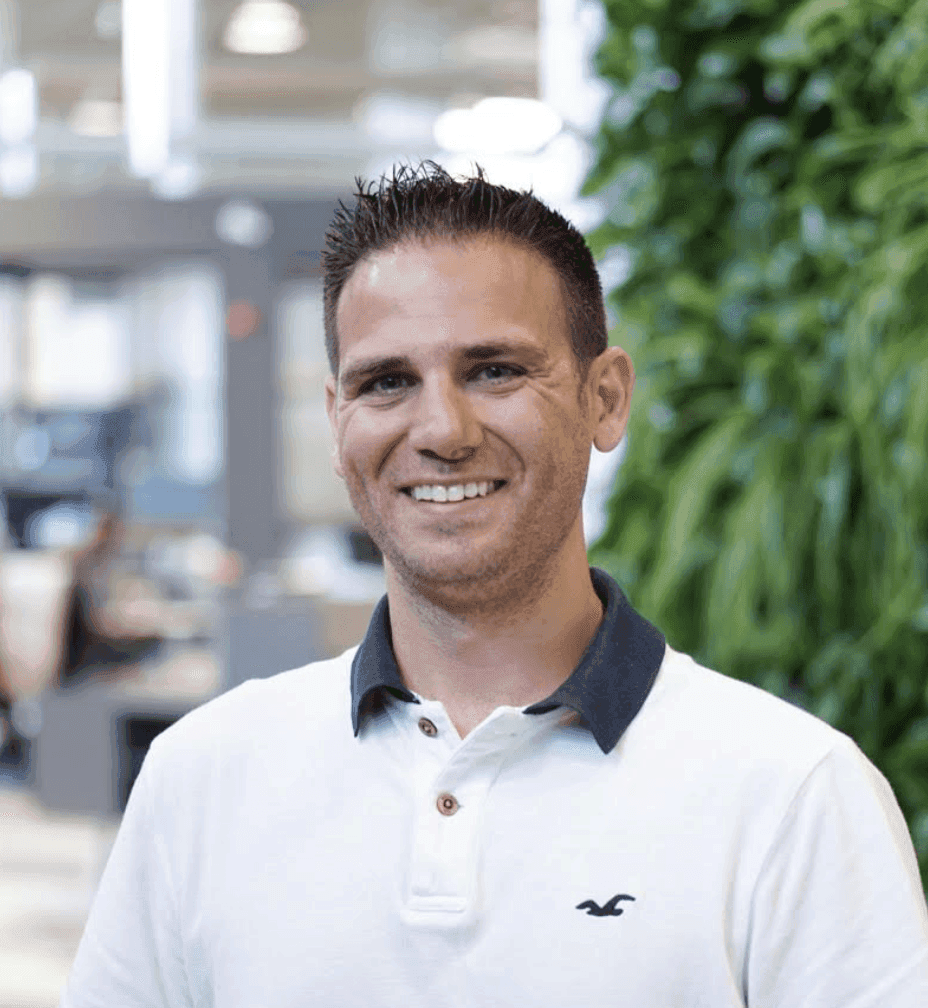
“Visibility on metrics in V7 is very helpful to us, and it's something we didn’t have in our internal solution.”
Andrew Achkar
Technical Director at Miovison
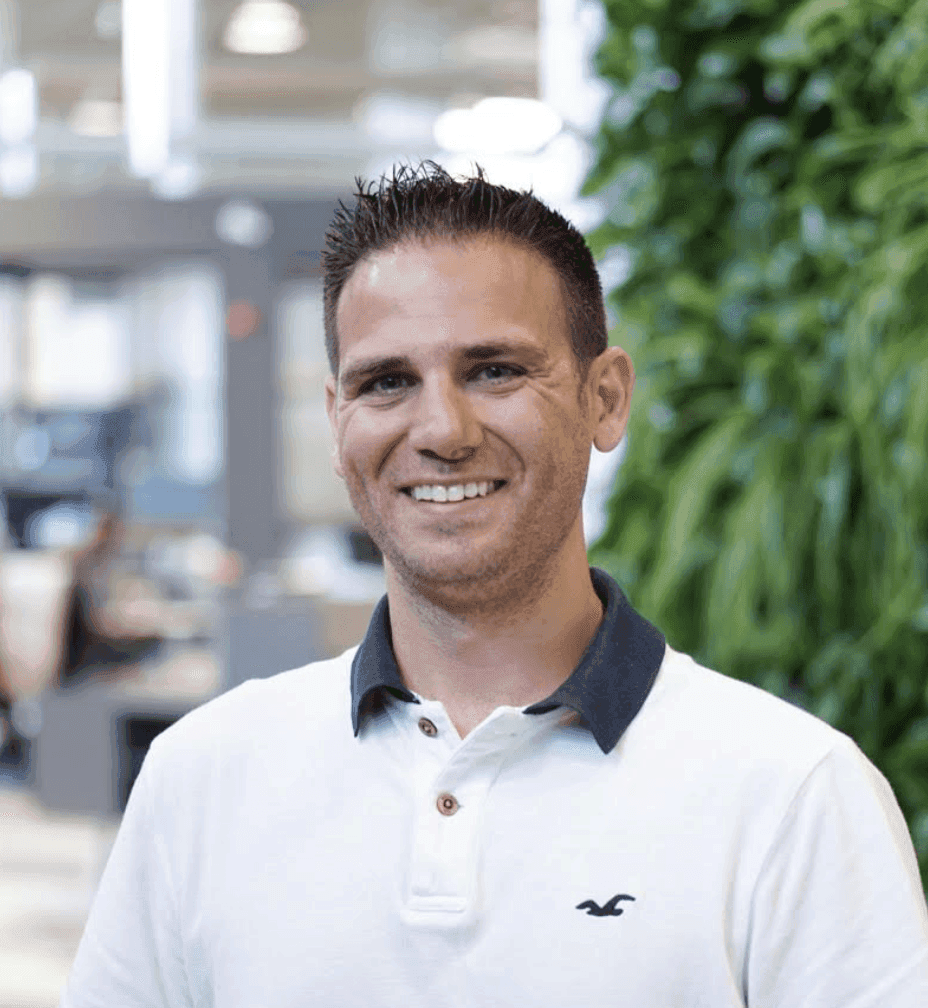
“Visibility on metrics in V7 is very helpful to us, and it's something we didn’t have in our internal solution.”
Andrew Achkar
Technical Director at Miovison
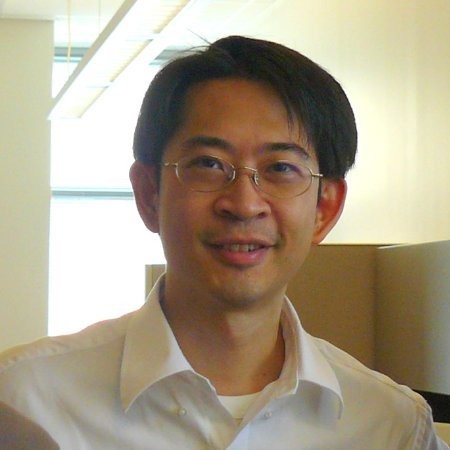
“V7 is great. The API is very straightforward to use, so we can easily get data into our system.”
David Soong
Director, Translational Data Science at Genmab
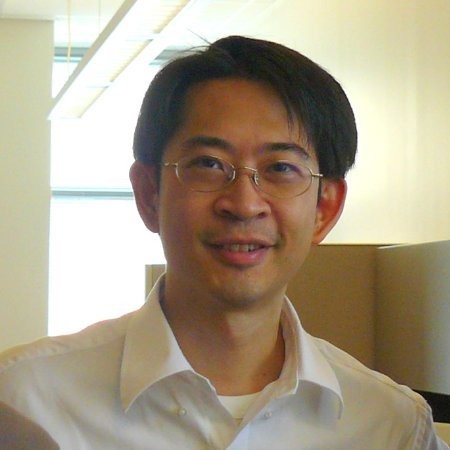
“V7 is great. The API is very straightforward to use, so we can easily get data into our system.”
David Soong
Director, Translational Data Science at Genmab
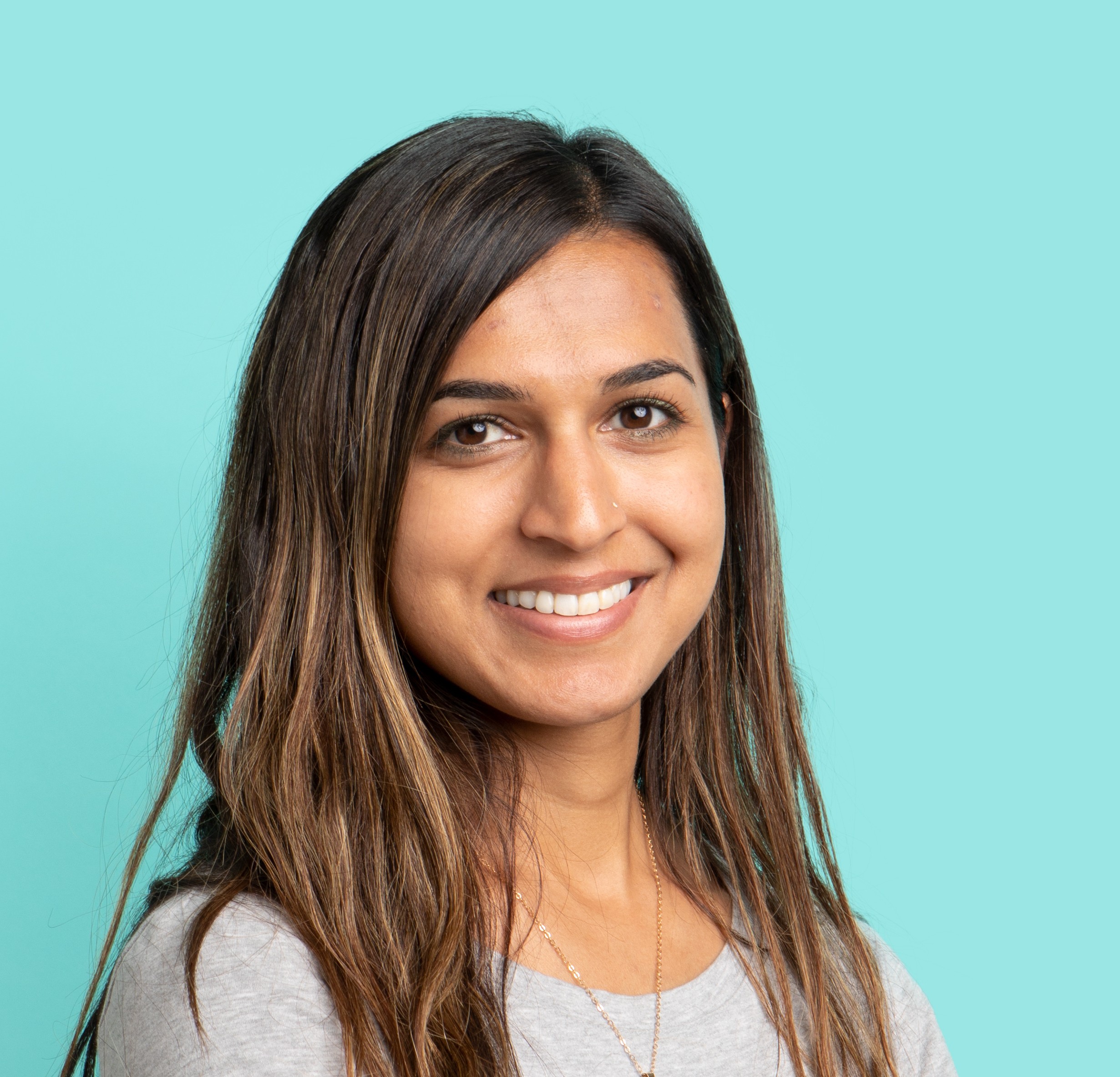
“We conducted extensive research of annotation tools and ultimately chose V7.”
Maleeha Nawaz
Manager of Quality and Data Curation at Imidex
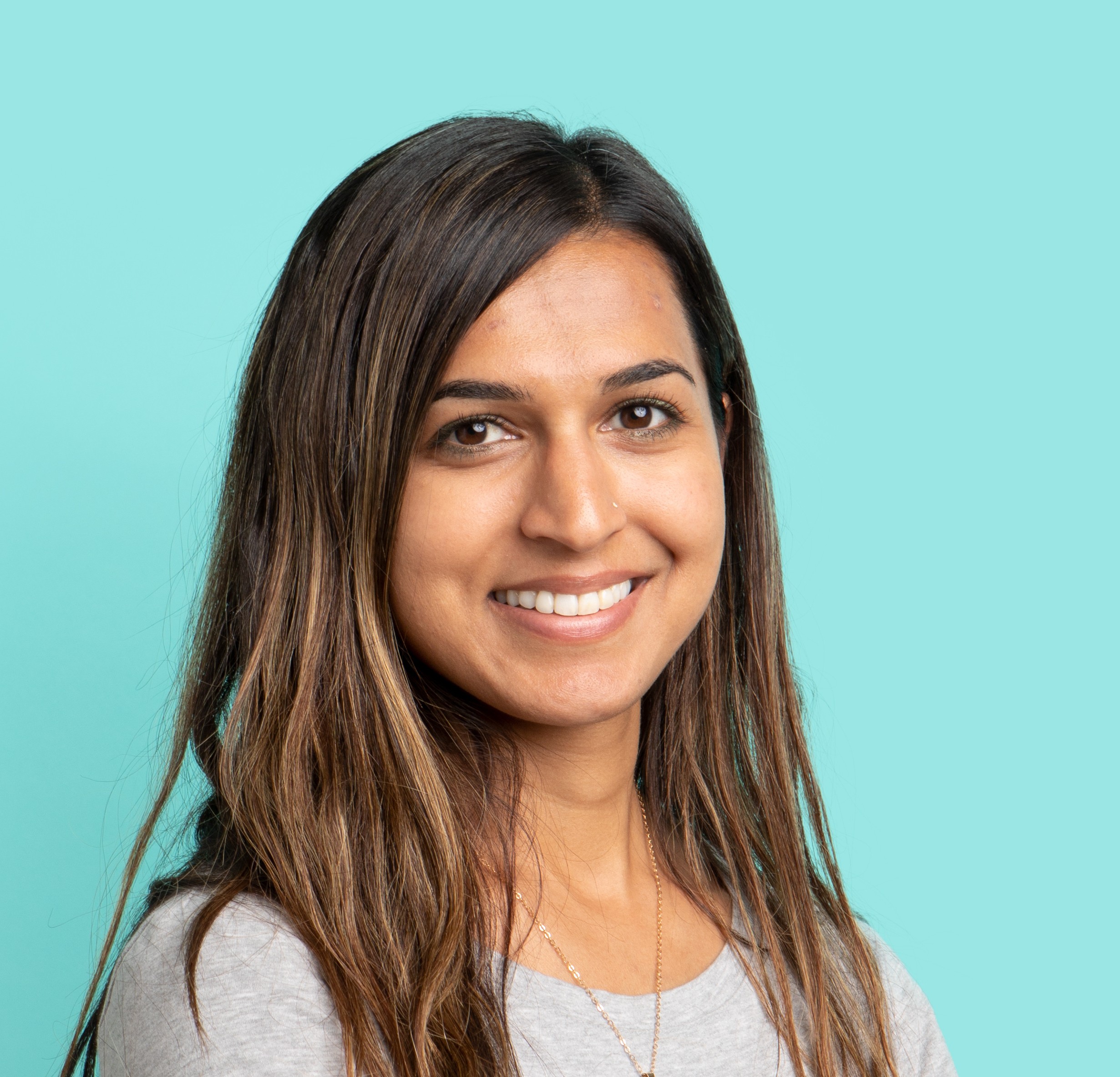
“We conducted extensive research of annotation tools and ultimately chose V7.”
Maleeha Nawaz
Manager of Quality and Data Curation at Imidex
Automated labeling
Automated labeling
Automated labeling
Annotate any dataset
with speed and accuracy
Annotate any dataset
with speed and accuracy
For many AI teams, creating high-quality training datasets is their biggest bottleneck. Annotation projects often stretch over months, consuming thousands of hours of meticulous work. V7 can speed up data annotation 10x, turning a months-long process into weeks.
Use intuitive interface and AI-assisted tools to turn complex labeling tasks into a few simple clicks and adjustments. Work with any size dataset and file type, from videos, PDFs, and architectural drawings to specialized medical formats like SVS or DICOM.
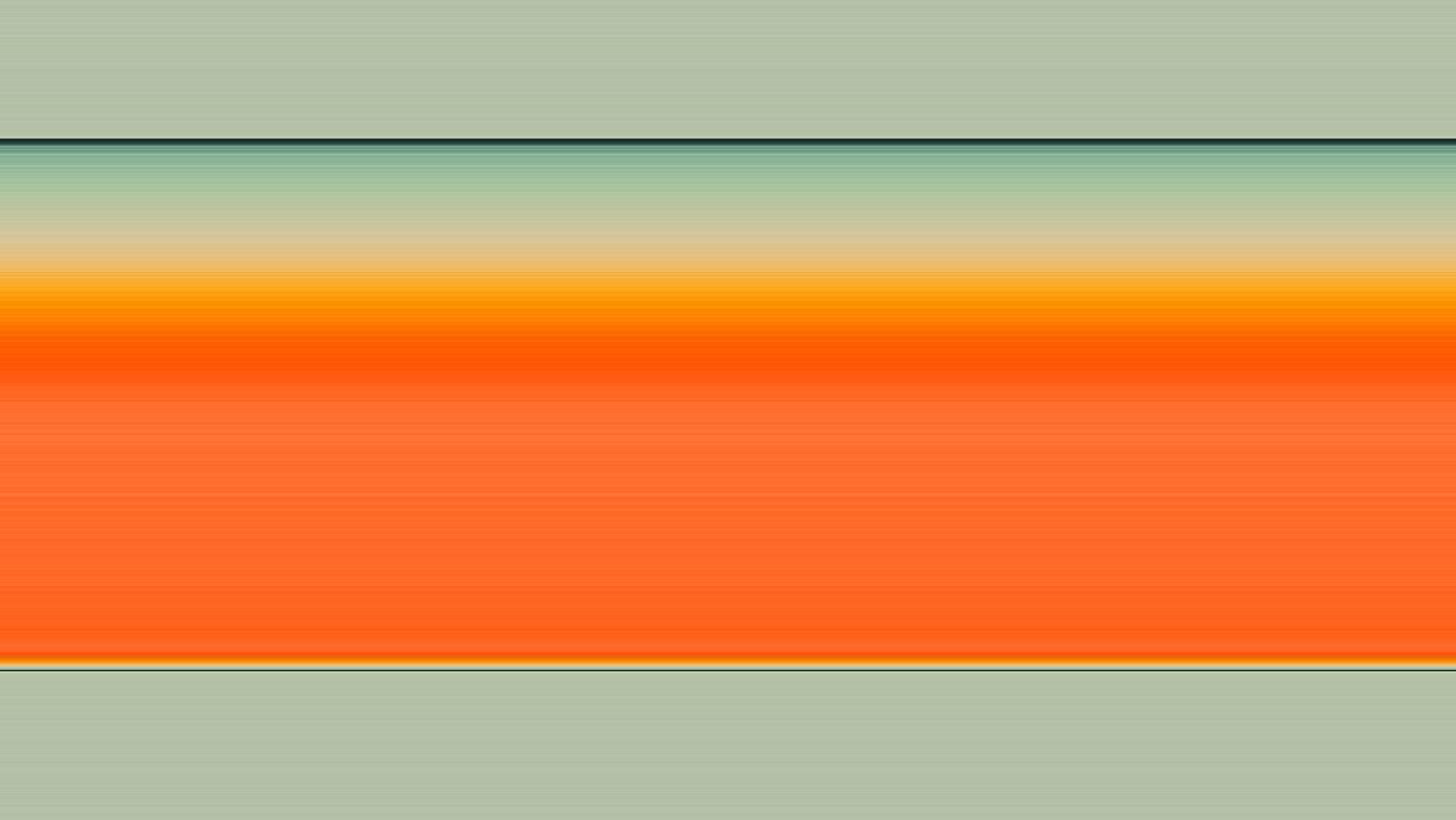
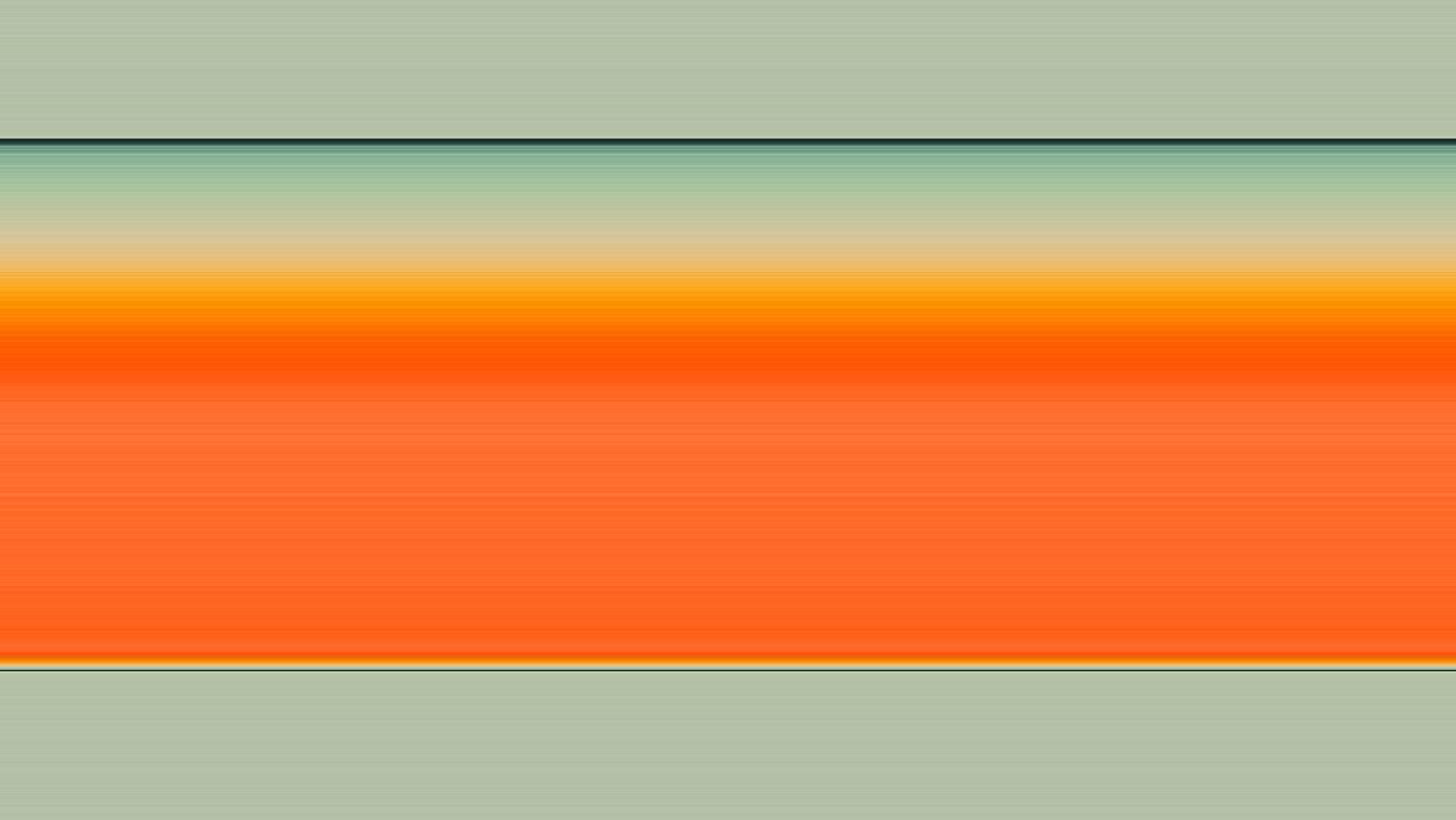
AI-assisted data labeling
Label data at lightning speed with V7 Auto-Annotate and SAM2. Segment complex objects like lesions in CT scans and items on assembly lines with high accuracy. Achieve expert level segmentation across diverse domains, regardless of industry.
AI-assisted data labeling
Label data at lightning speed with V7 Auto-Annotate and SAM2. Segment complex objects like lesions in CT scans and items on assembly lines with high accuracy. Achieve expert level segmentation across diverse domains, regardless of industry.
Auto-track for video
Track objects across selected time ranges in videos. Automatically follow instances and mark in-and-out of view situations. Streamline video annotation for tasks like AI-assisted surgeries, retail shrinkage prevention, or sports analytics.
Auto-track for video
Track objects across selected time ranges in videos. Automatically follow instances and mark in-and-out of view situations. Streamline video annotation for tasks like AI-assisted surgeries, retail shrinkage prevention, or sports analytics.
Label similar objects
Pick one object and find similar ones automatically. Speed up repetitive annotation problems like preparing training data for cell counting models or product identification on shelves. Reduce manual effort in large-scale labeling projects. Reduce human errors caused by fatigue and monotony.
Label similar objects
Pick one object and find similar ones automatically. Speed up repetitive annotation problems like preparing training data for cell counting models or product identification on shelves. Reduce manual effort in large-scale labeling projects. Reduce human errors caused by fatigue and monotony.
Model in the loop
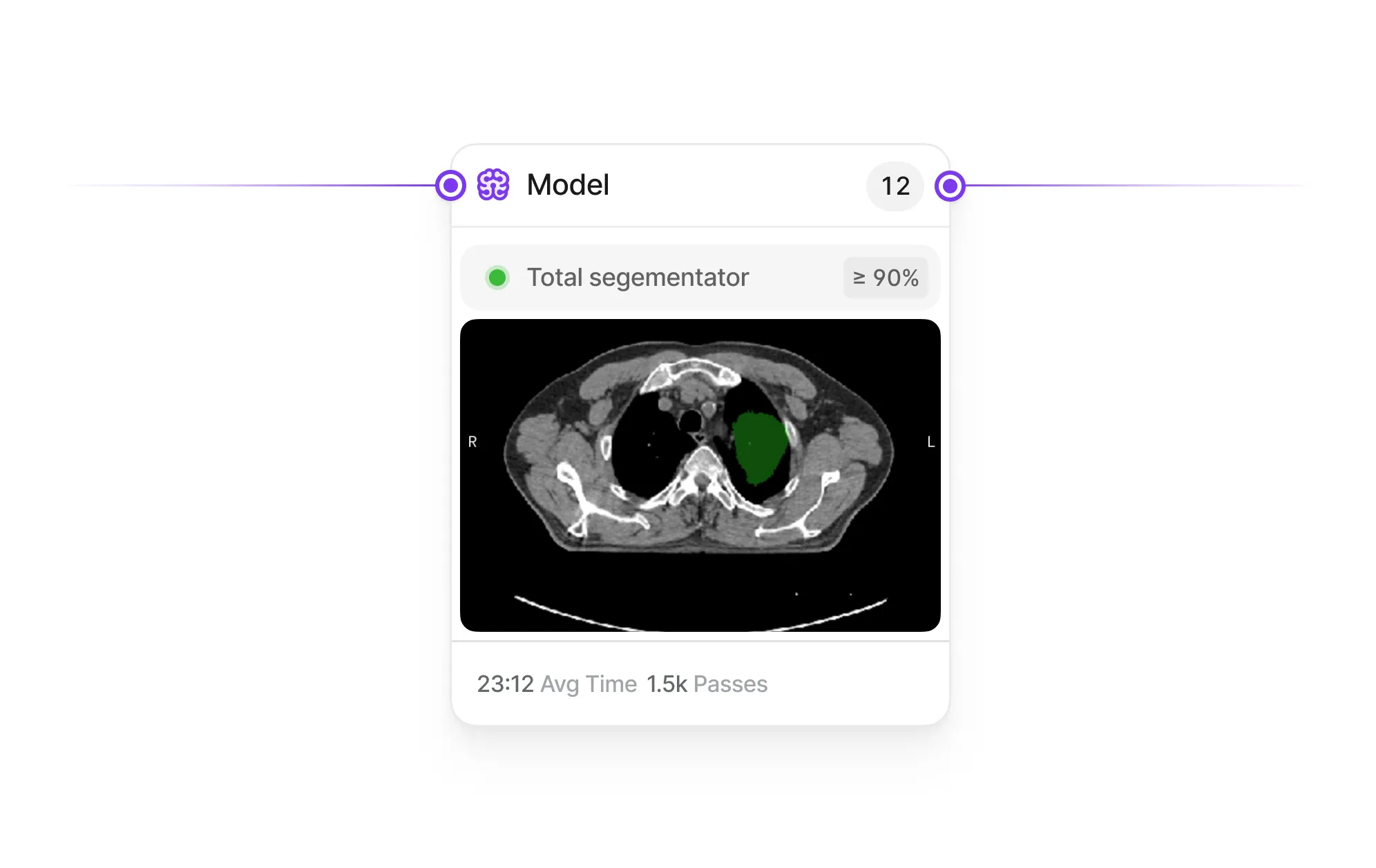
Integrate external or out-of-the-box models to pre-label your data or detect quality issues. Compare performance between human labelers and AI models in blind tests. Connect models to your ML pipeline and improve your annotation process and model accuracy.
Model in the loop
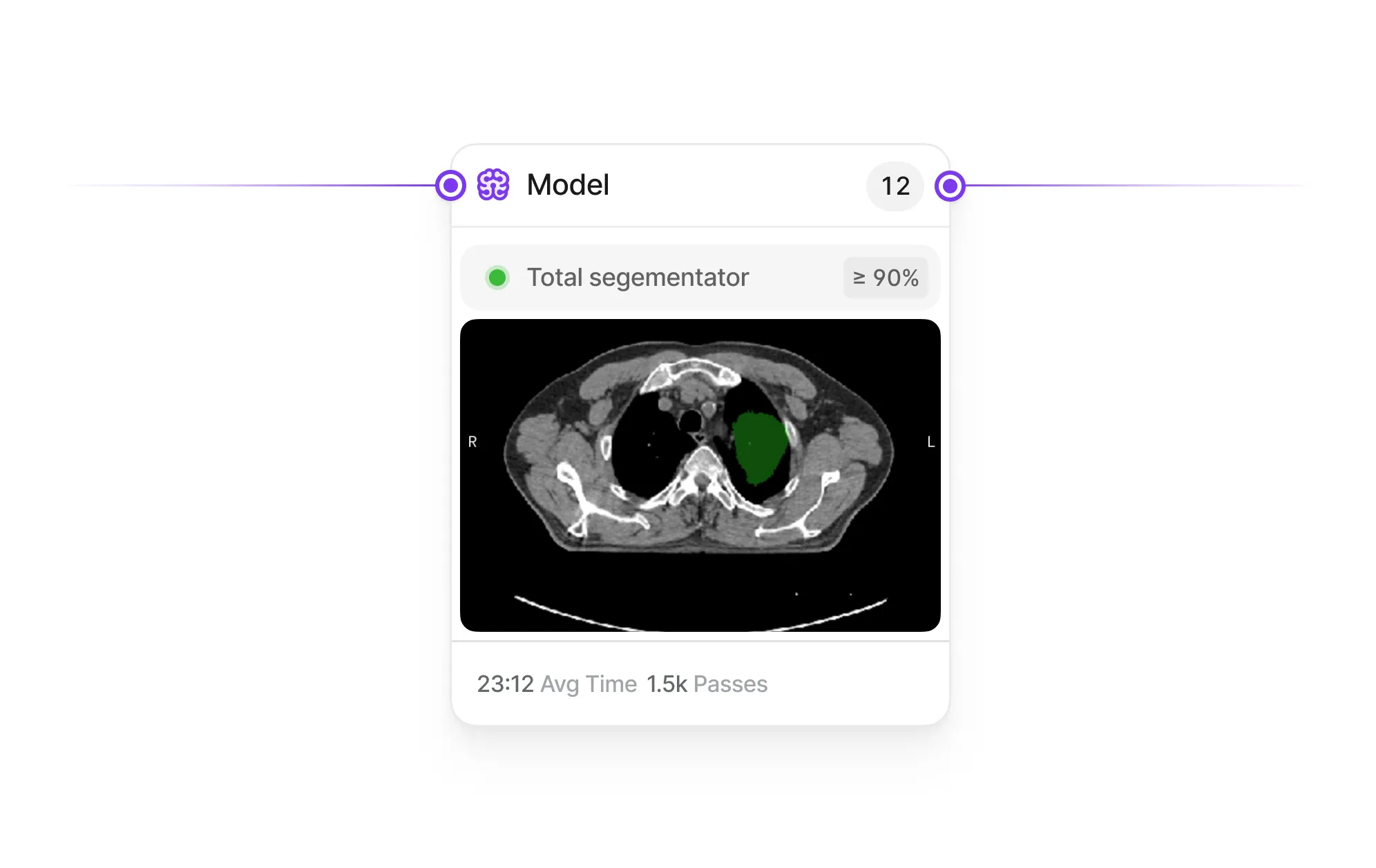
Integrate external or out-of-the-box models to pre-label your data or detect quality issues. Compare performance between human labelers and AI models in blind tests. Connect models to your ML pipeline and improve your annotation process and model accuracy.
Workflows
Workflows
Workflows
Leverage humans in the loop.
Build better ML pipelines.
Leverage humans in the loop. Build better ML pipelines.
As annotation projects scale, AI teams struggle to track progress, maintain QA standards, and ensure accountability. Inconsistencies can quickly compound, leading to compromised data quality—which impacts the entire AI development lifecycle.
V7 allows you to design multi-stage review workflows to orchestrate your labeling process. Assign roles, tasks, and manage project completion. Use conditional logic and automations to always route data to the right stages or team members.
Workflow
Consensus
Stages
Dataset
01
Consensus
02
Logic
03
Review
04
Annotate
05
Complete
06
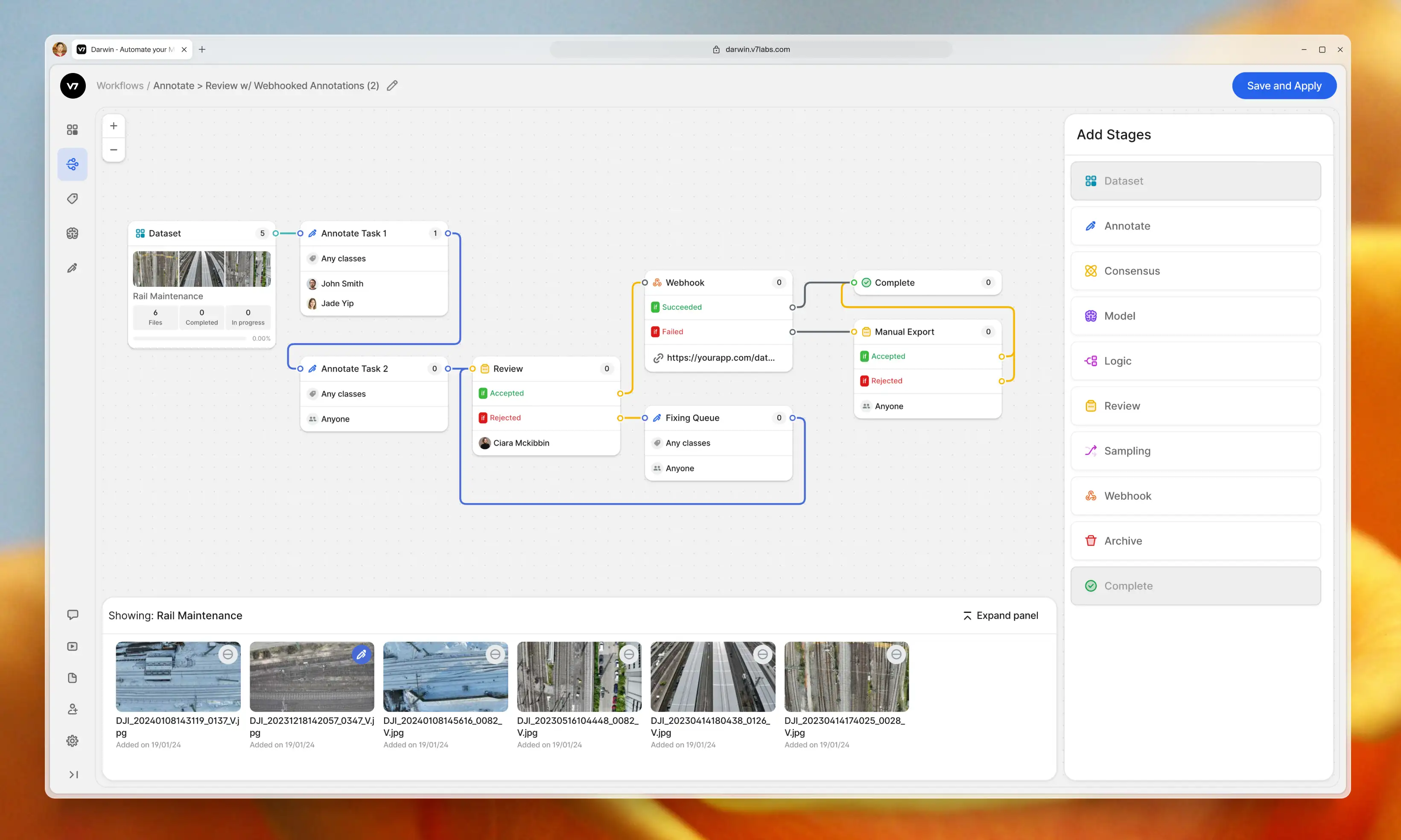
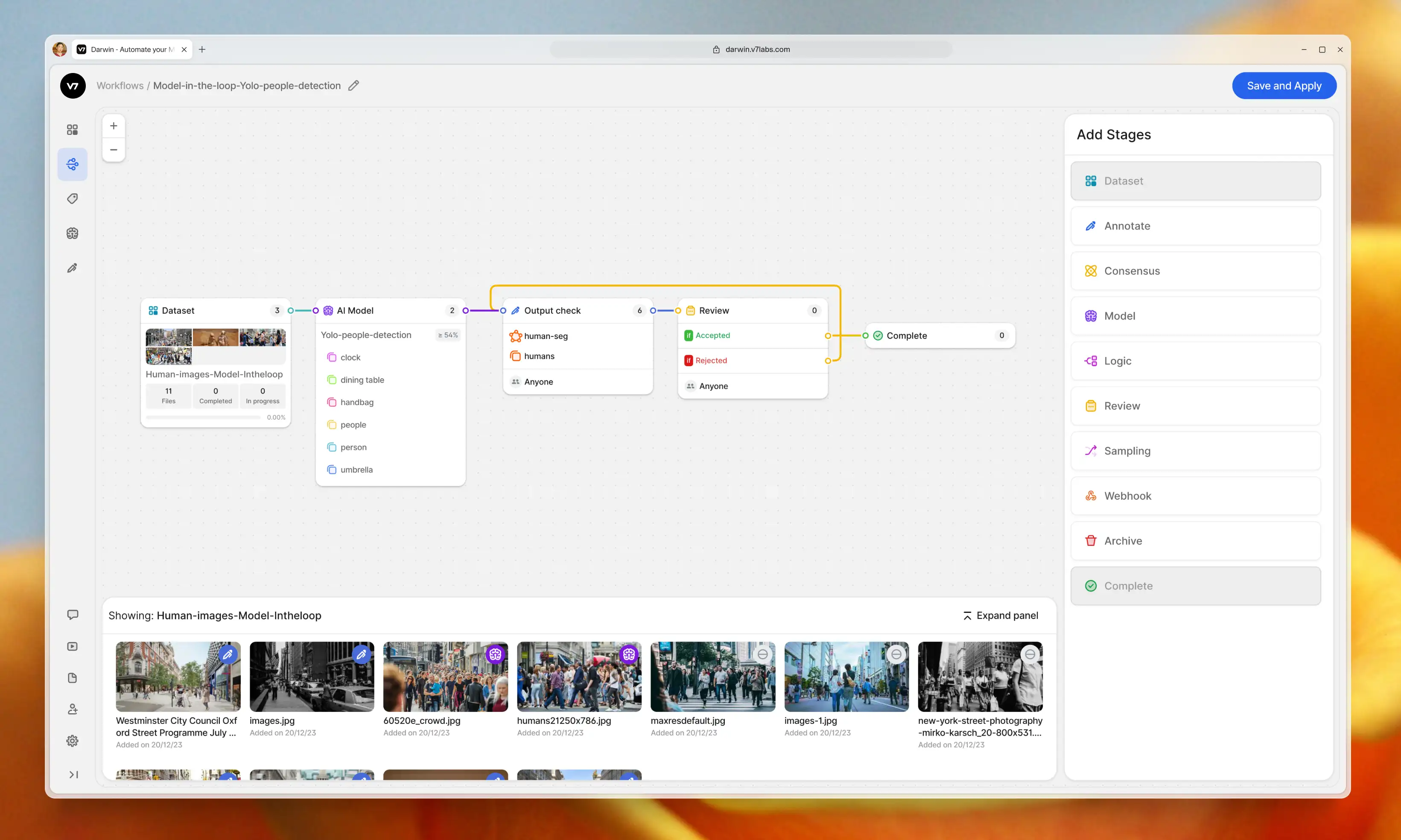
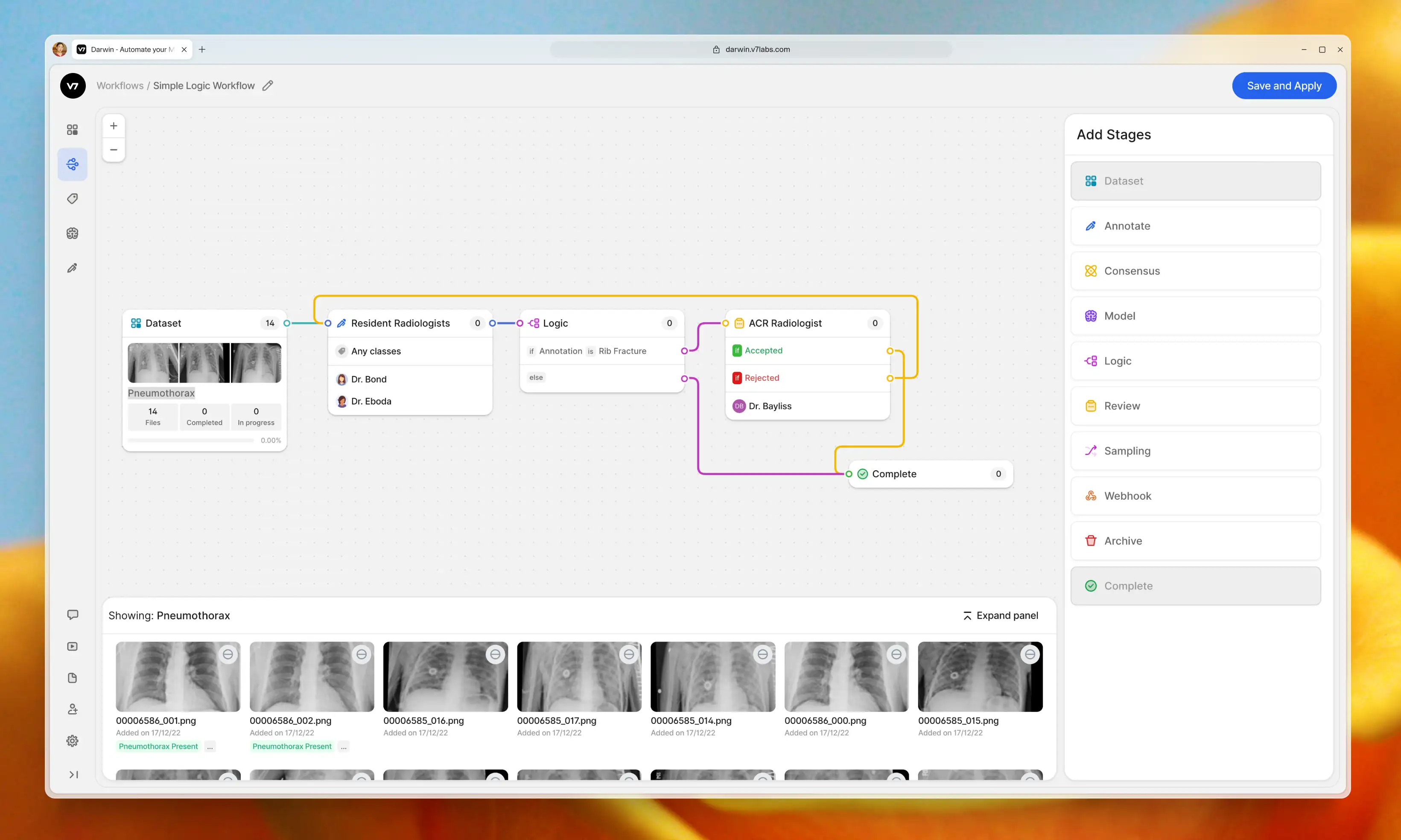
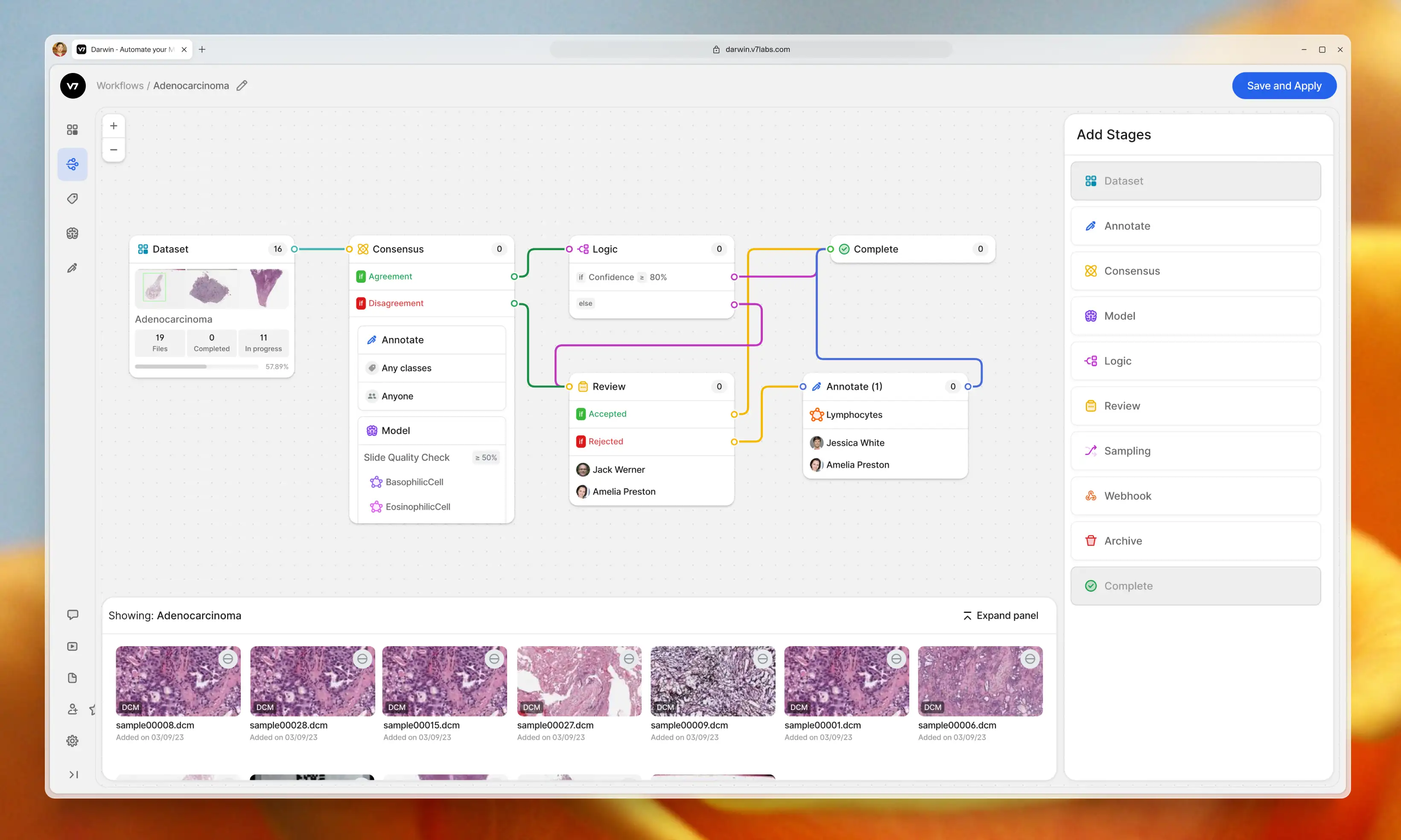
Workflow
Consensus
Stages
Dataset
01
Consensus
02
Logic
03
Review
04
Annotate
05
Complete
06
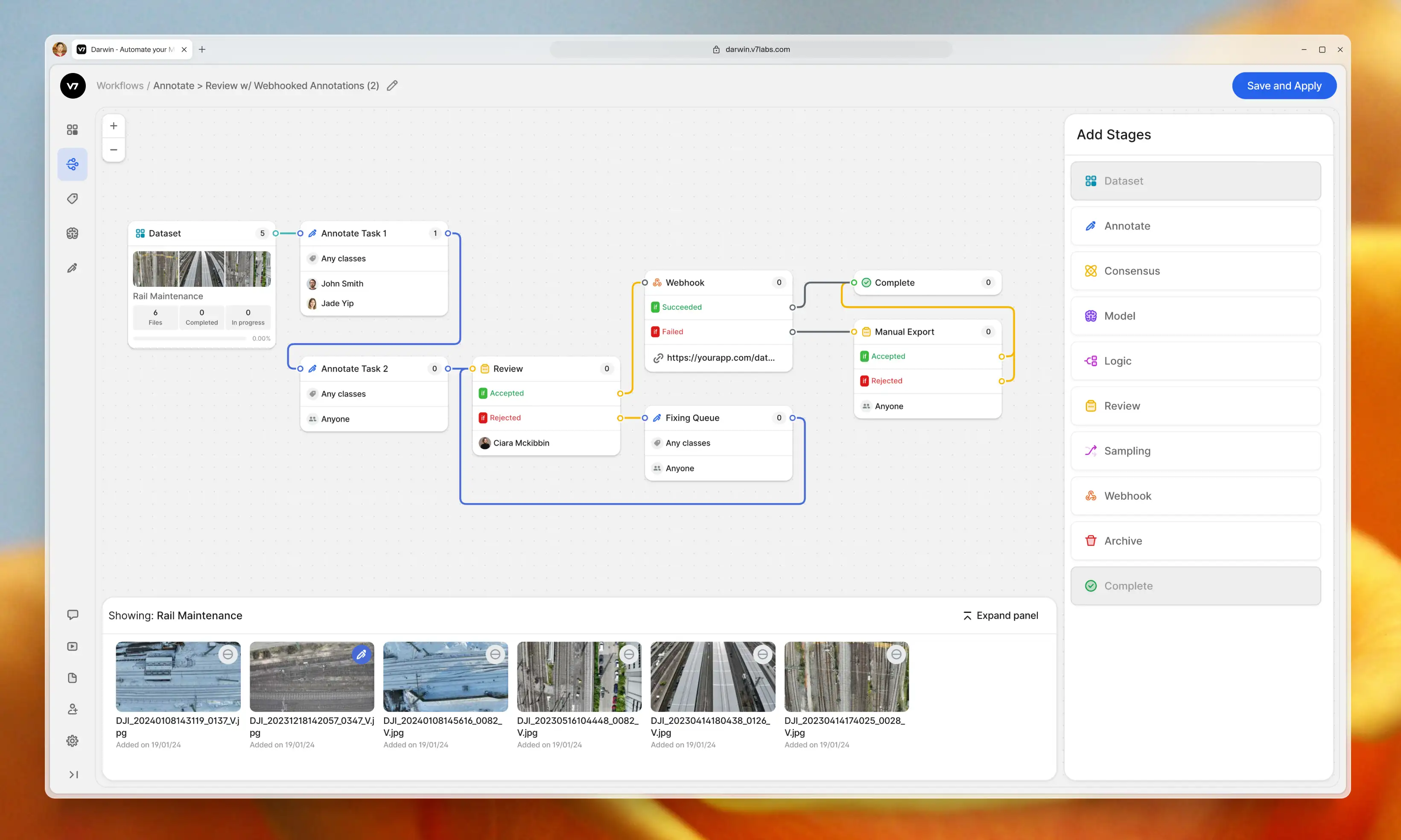
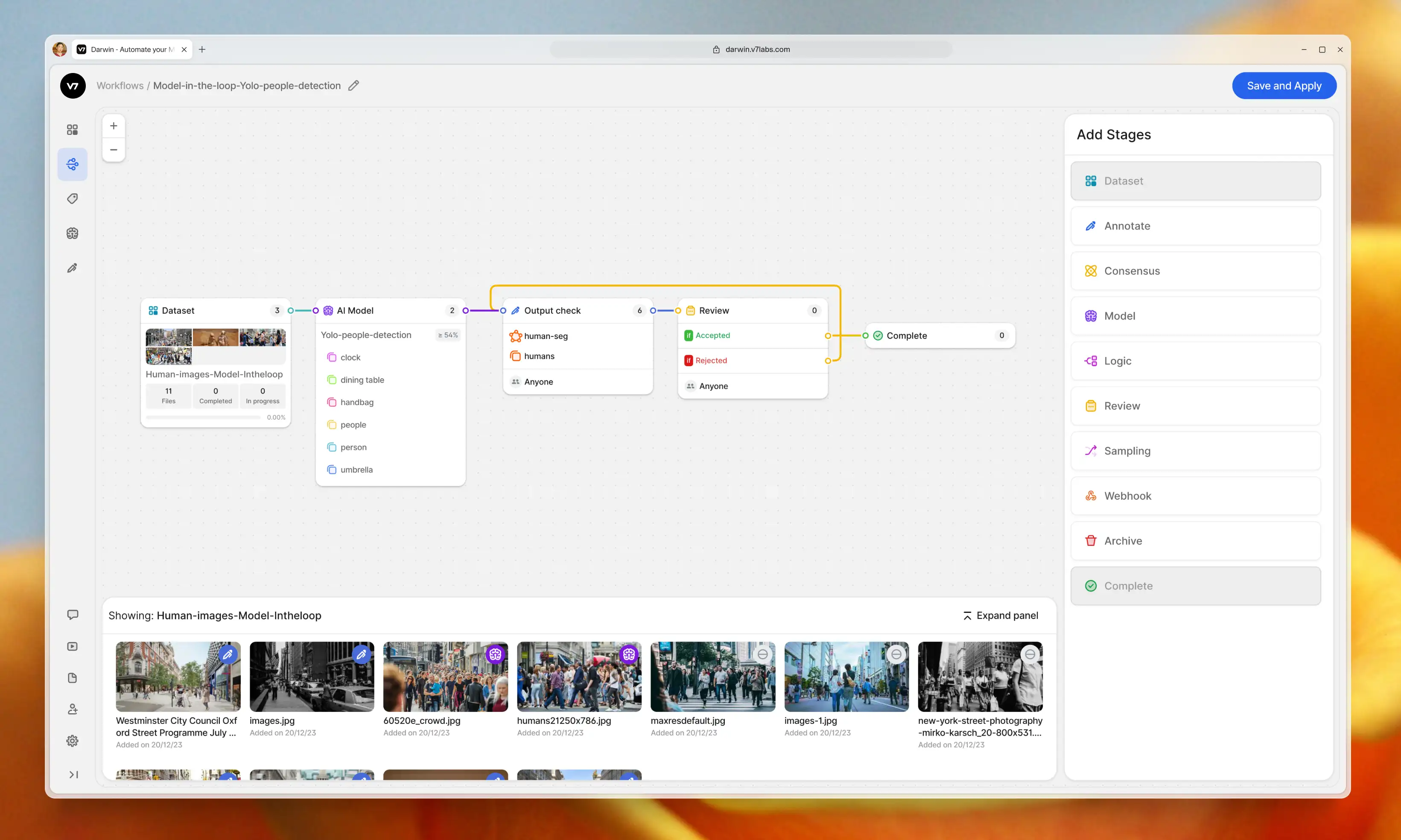
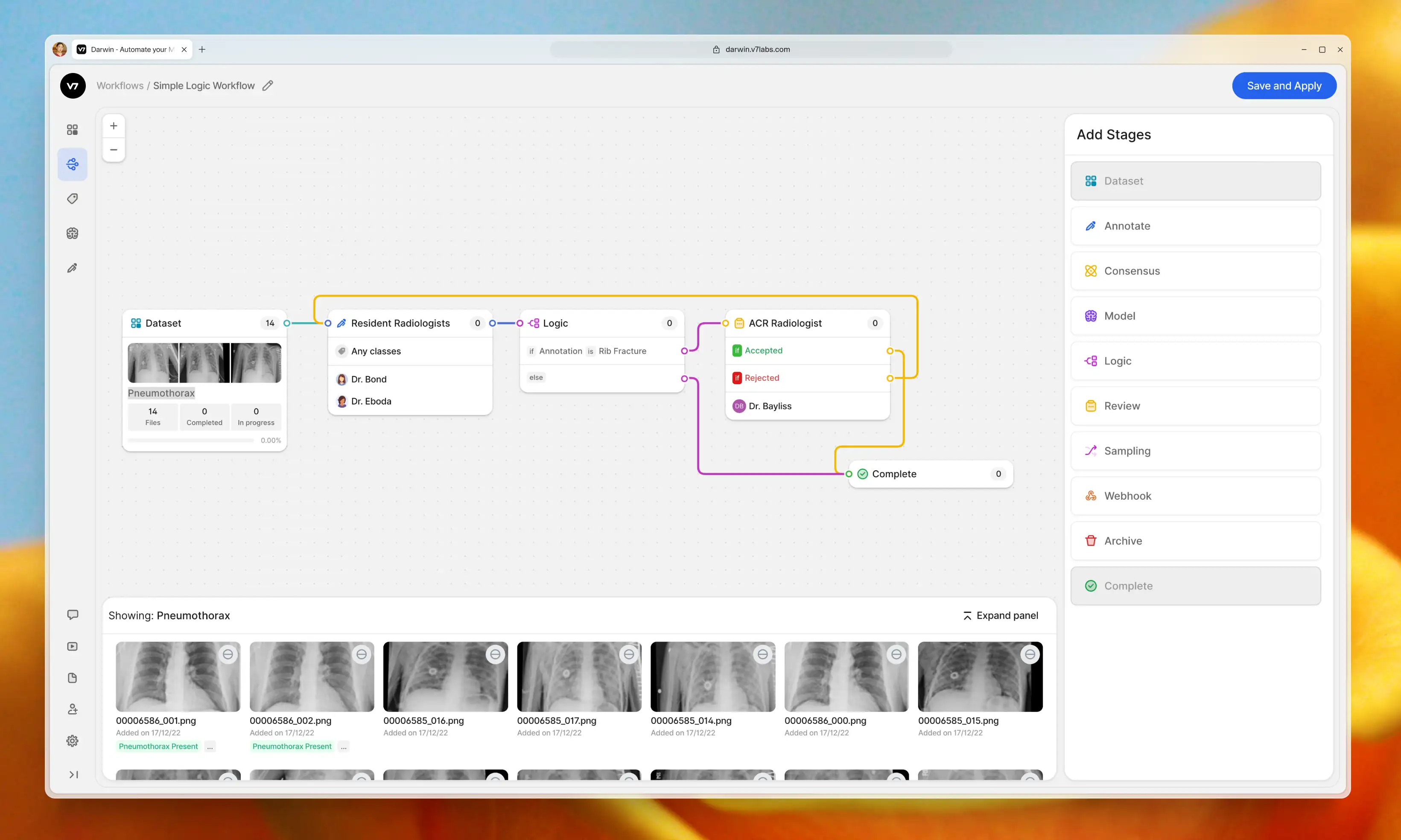
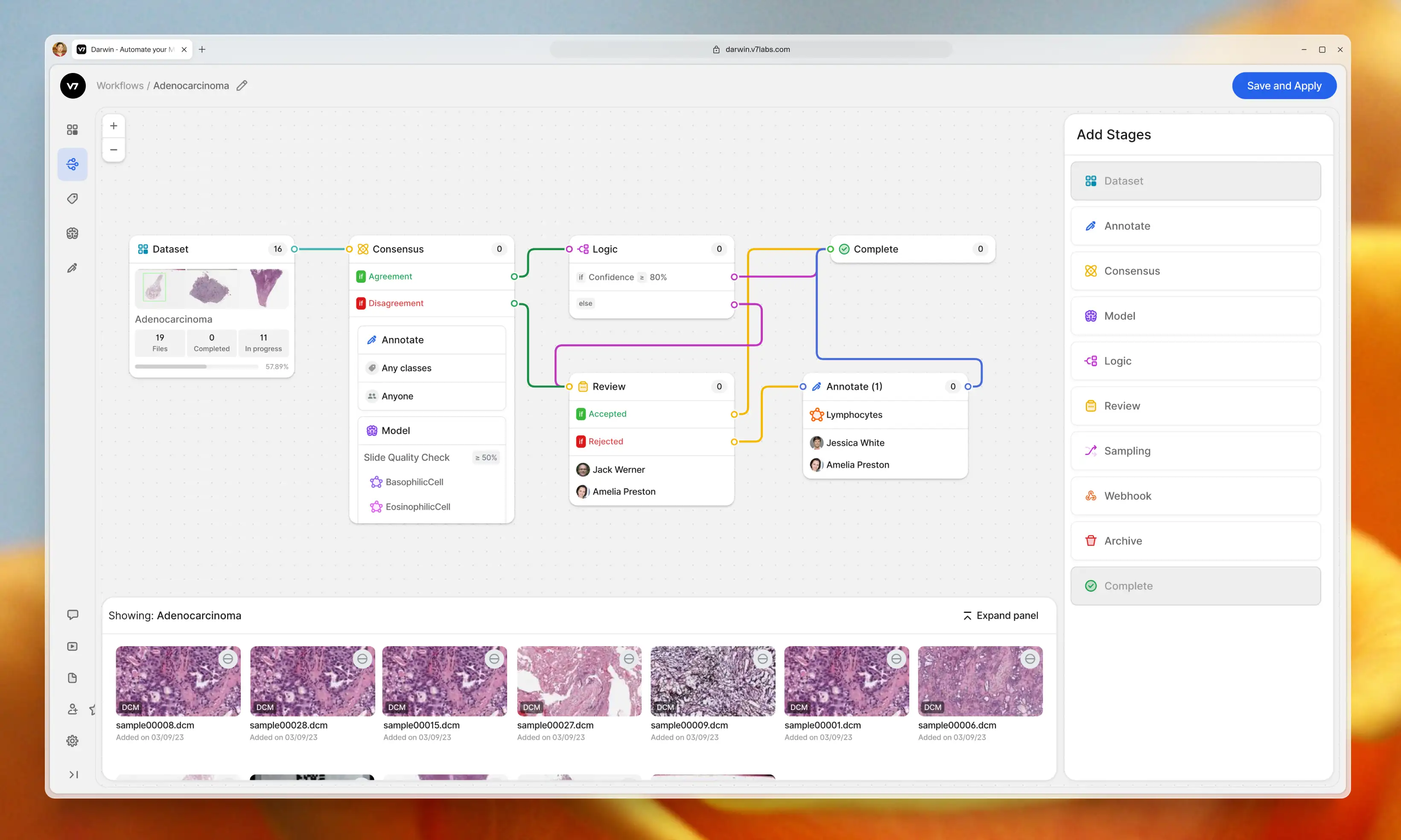
Collaborate
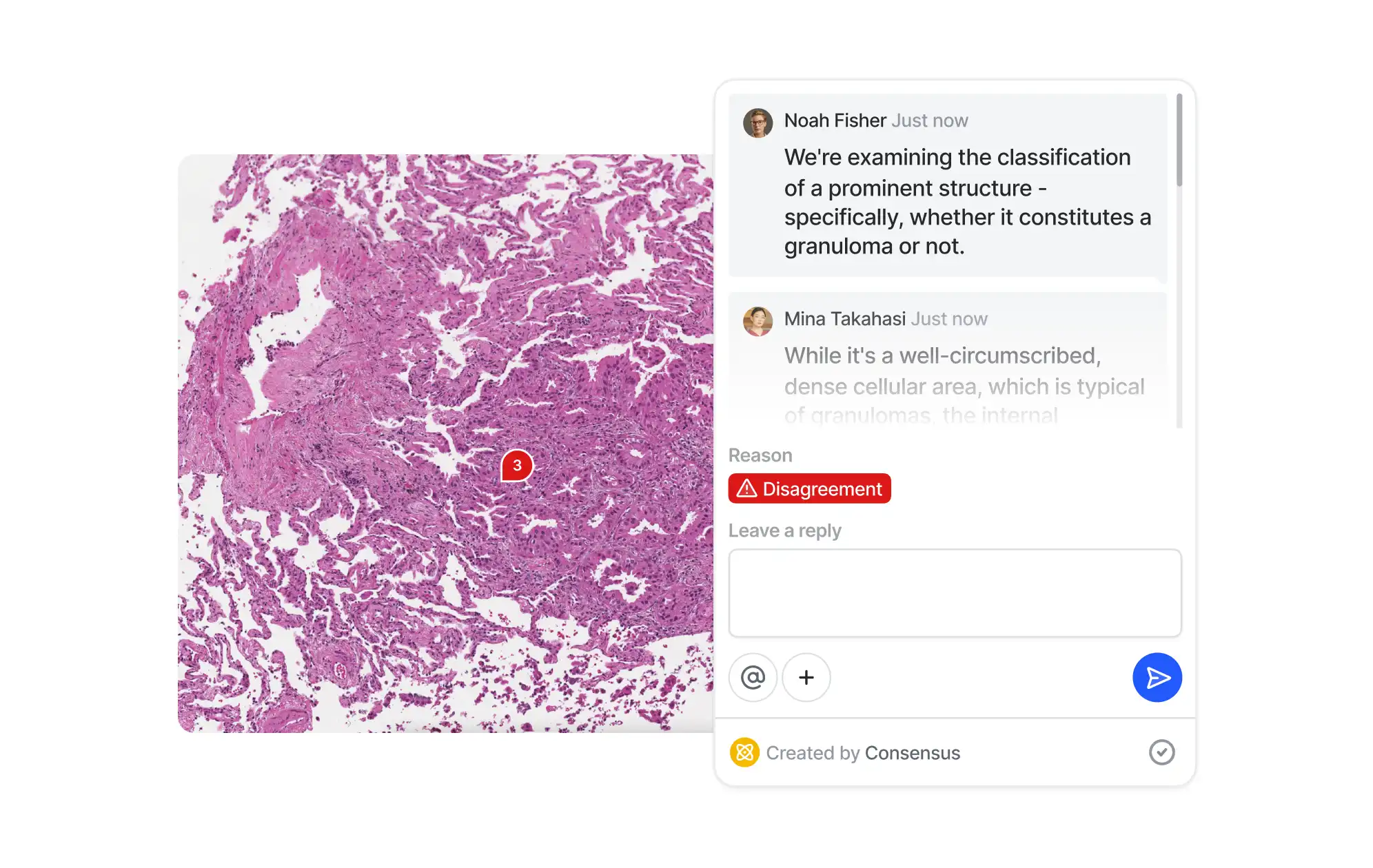
Improve teamwork with real-time collaboration features. Ensure smooth communication between annotators, reviewers, and ML engineers to speed up assignments. Delegate tasks and track progress of multiple independent annotation projects.
Collaborate
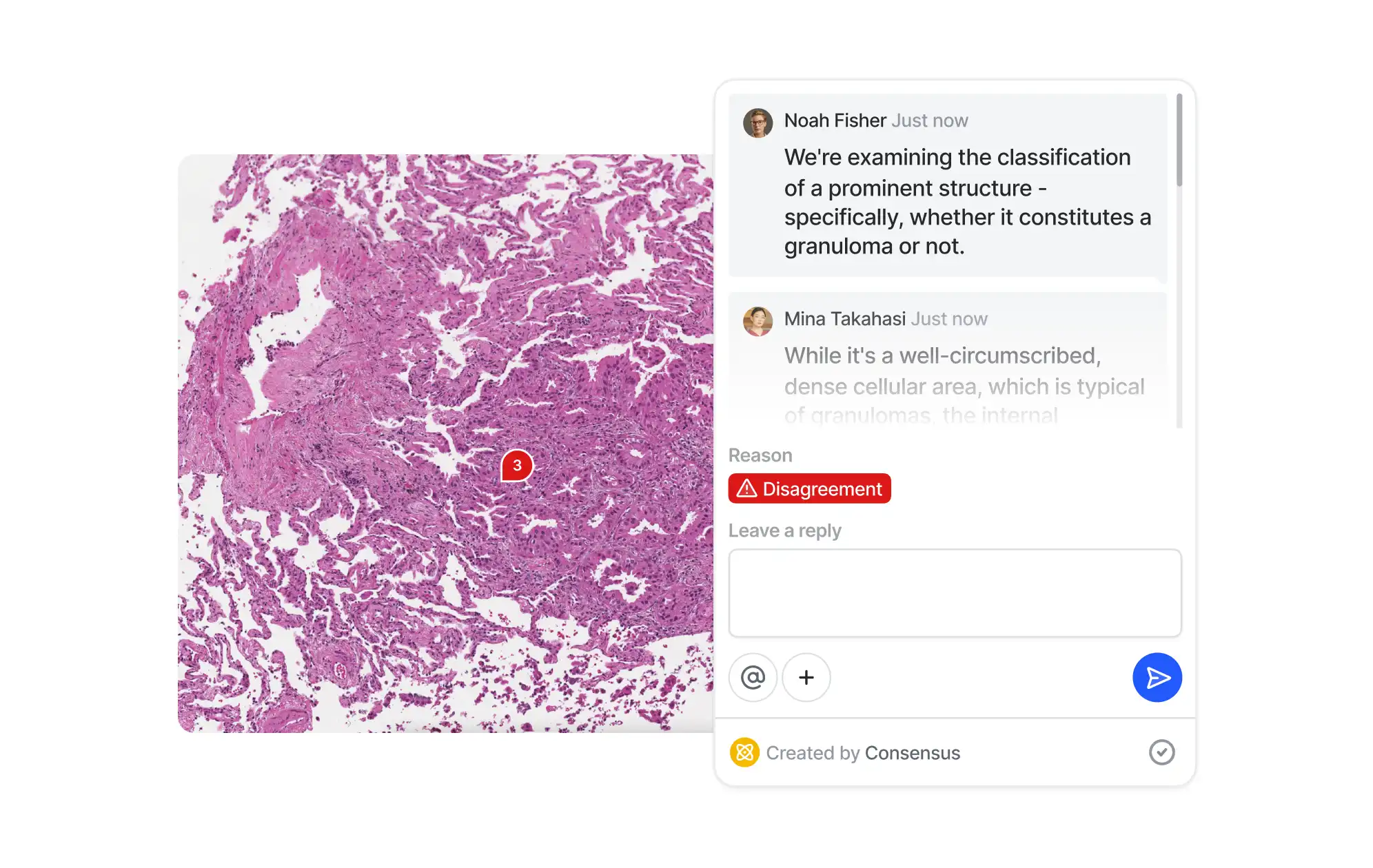
Improve teamwork with real-time collaboration features. Ensure smooth communication between annotators, reviewers, and ML engineers to speed up assignments. Delegate tasks and track progress of multiple independent annotation projects.
Consensus
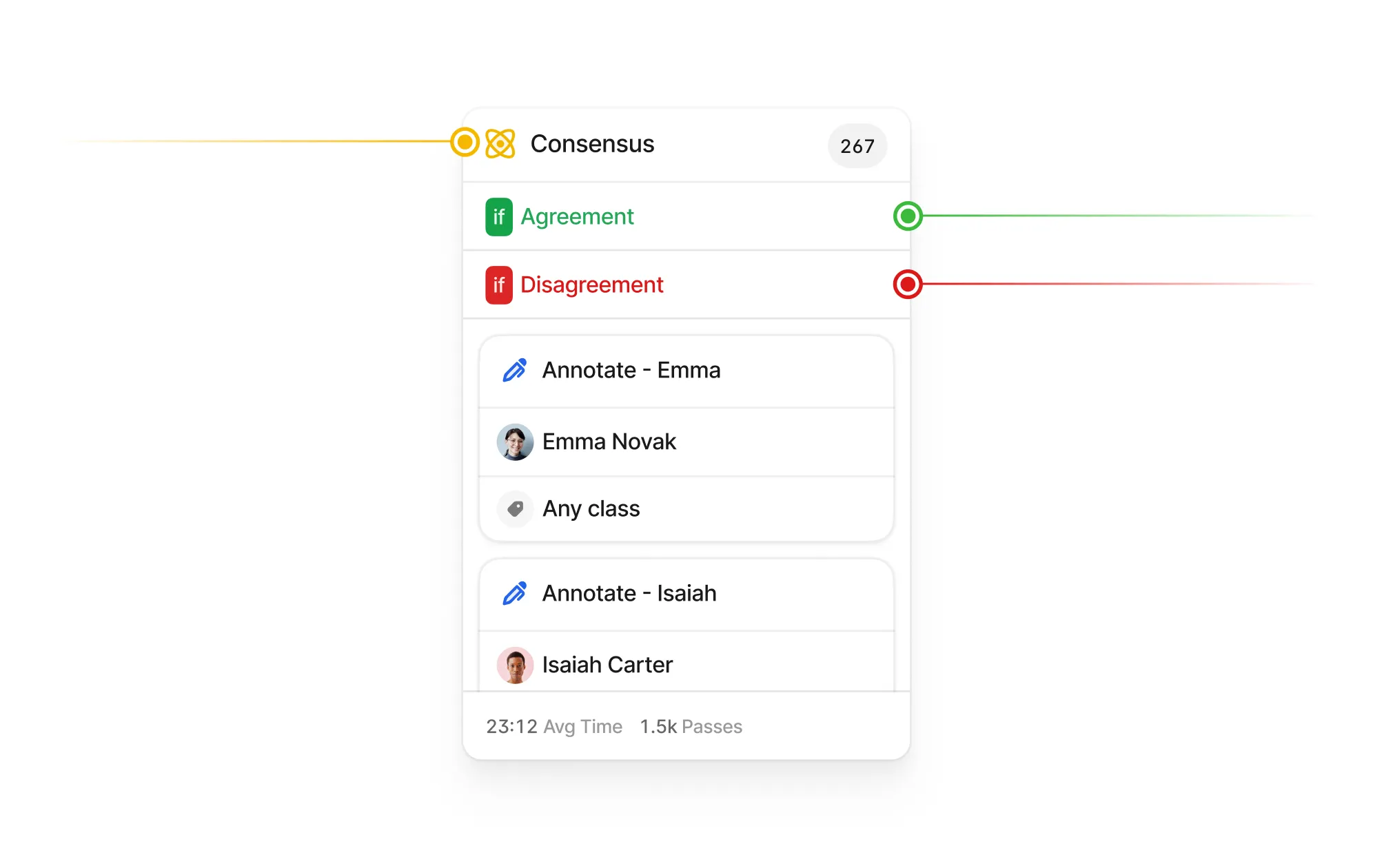
Ensure data integrity with blind tests. Measure agreement and overlap between multiple annotators or AI models. Use the Consensus Stage to surface discrepancies, resolve conflicts, and identify challenging data points.
Consensus
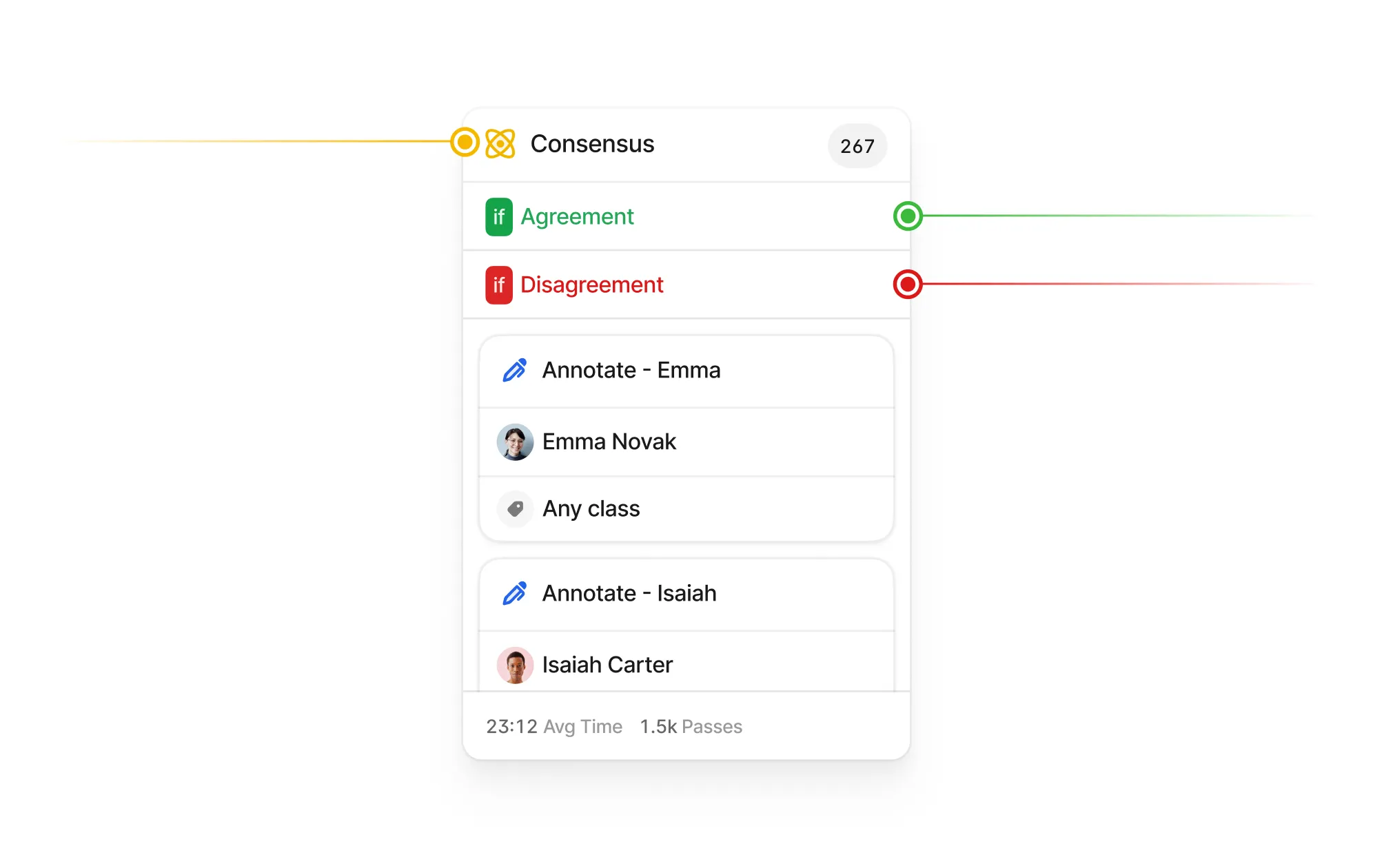
Ensure data integrity with blind tests. Measure agreement and overlap between multiple annotators or AI models. Use the Consensus Stage to surface discrepancies, resolve conflicts, and identify challenging data points.
Evaluate labelers
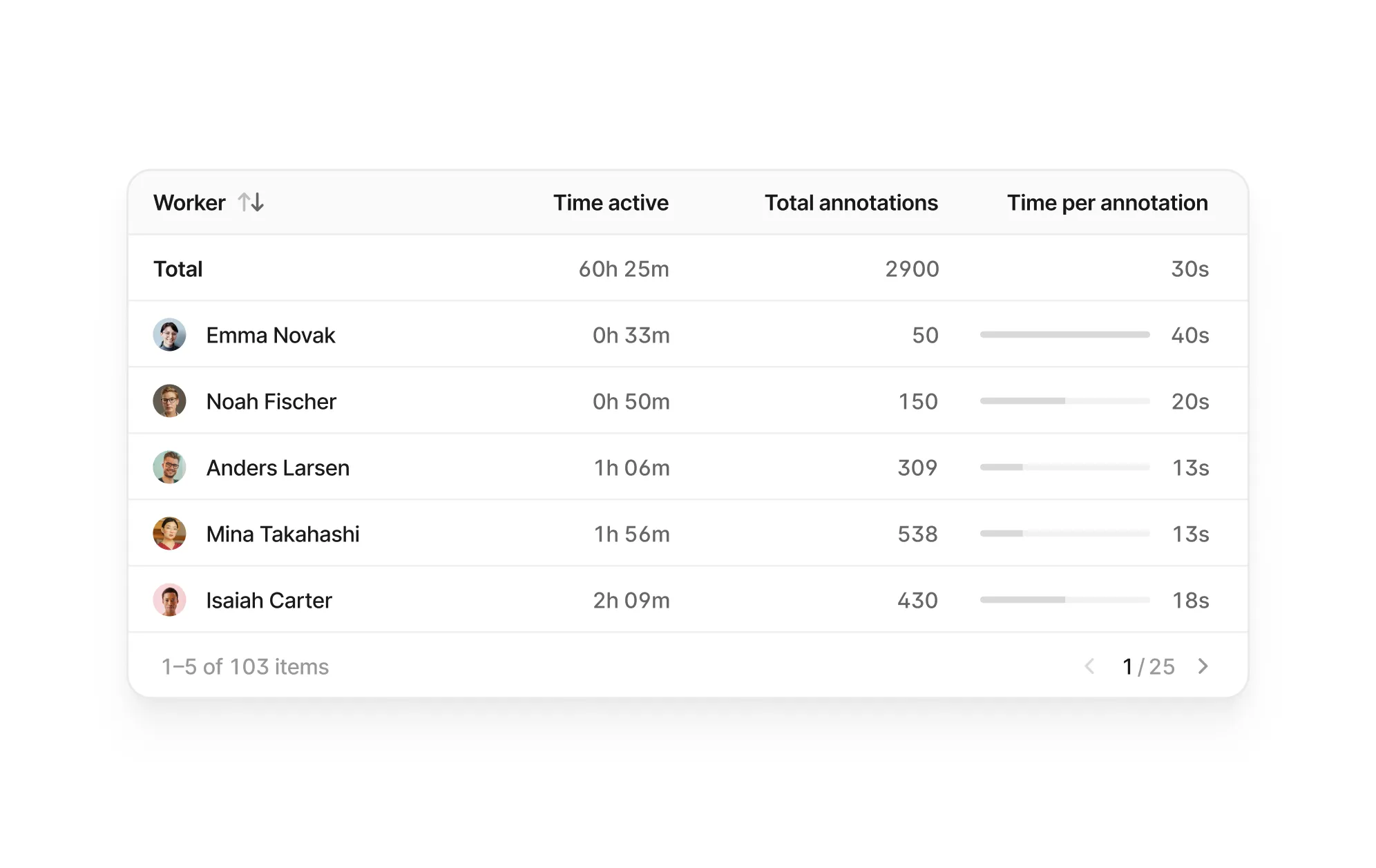
Use advanced reporting features to monitor productivity and accuracy. Identify and address bottlenecks to optimize your annotation process. Speed up the QA process by adjusting the amount of data that needs additional reviews.
Evaluate labelers
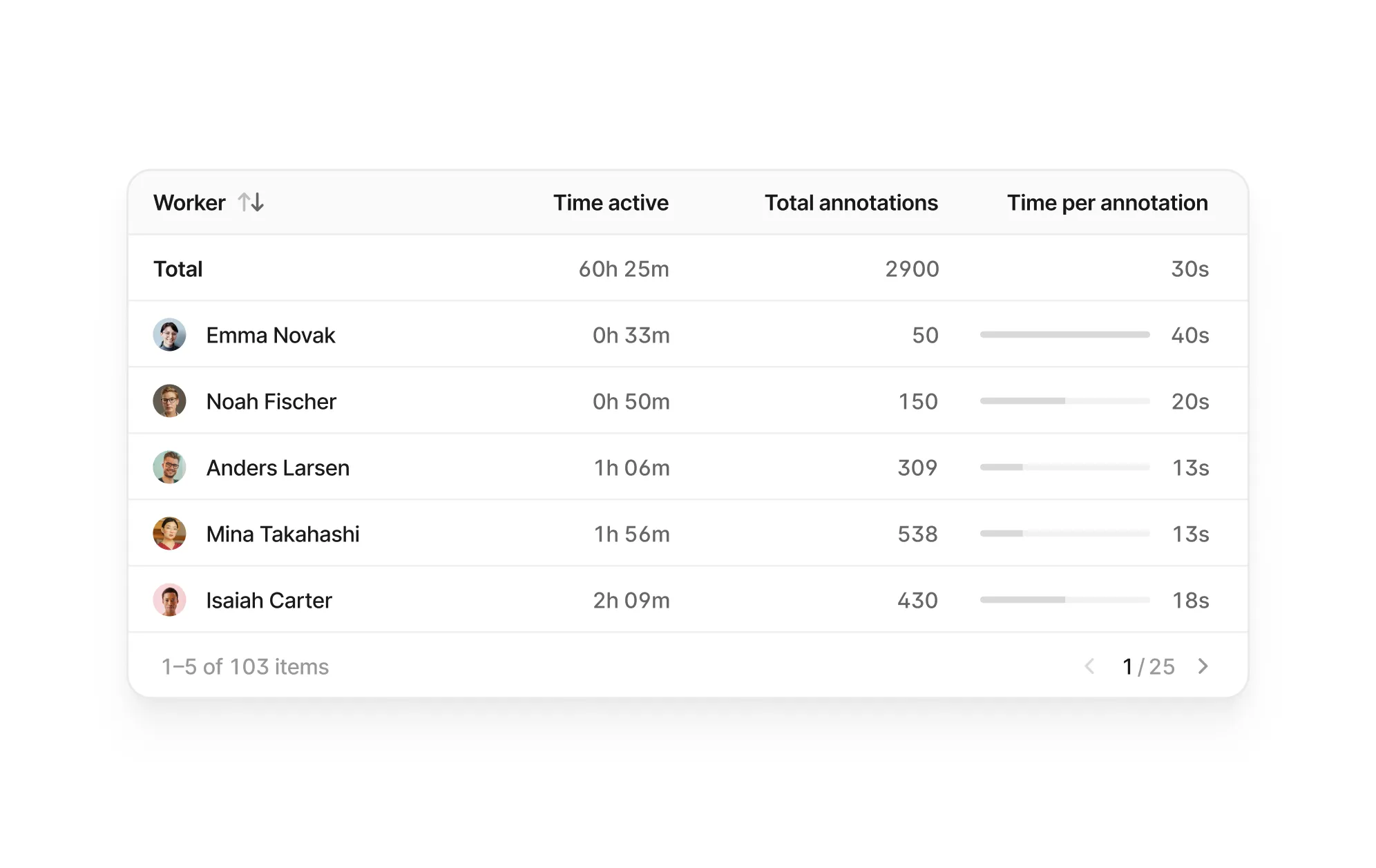
Use advanced reporting features to monitor productivity and accuracy. Identify and address bottlenecks to optimize your annotation process. Speed up the QA process by adjusting the amount of data that needs additional reviews.
Data labeling services
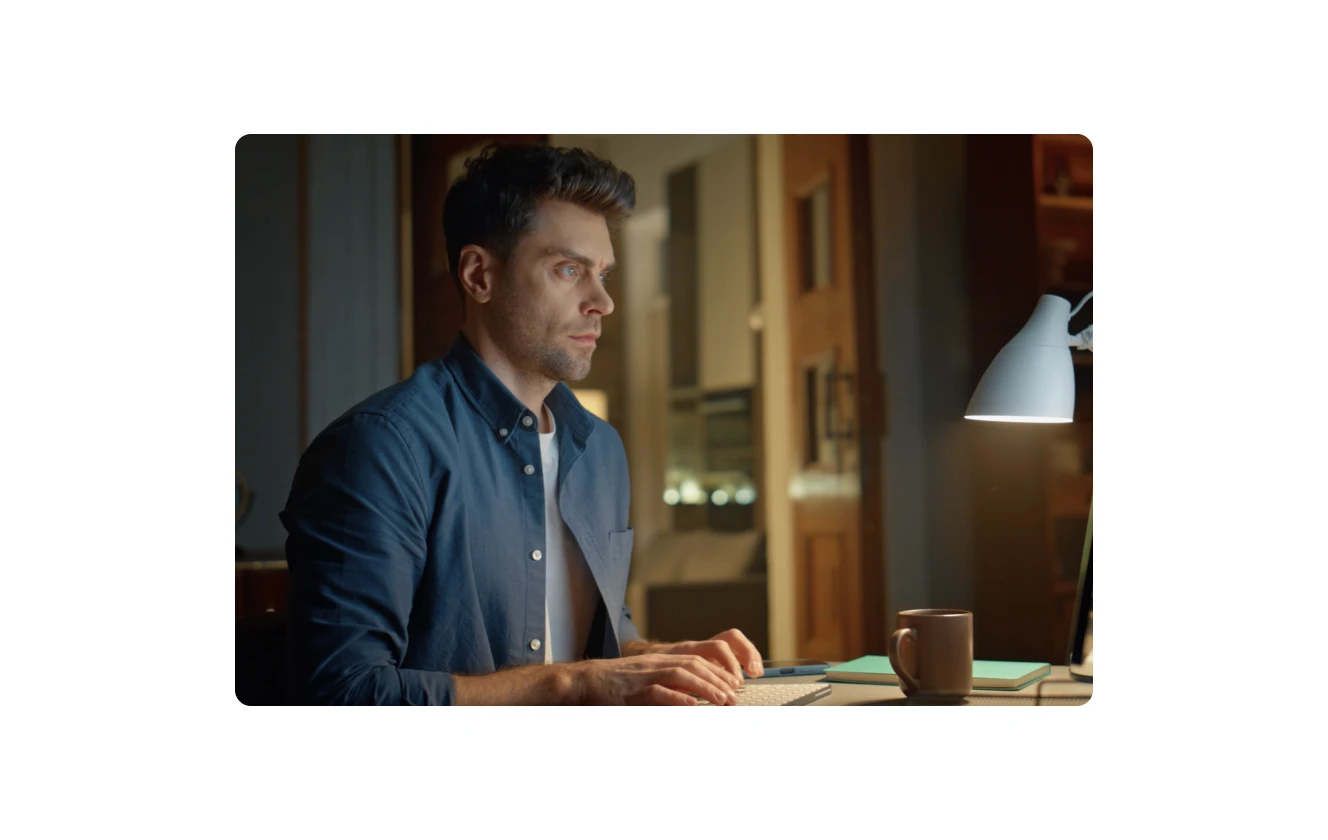
Scale your annotation efforts with on-demand professional labeling services. Access our network of trained annotators with expertise in various domains, from healthcare to linguistics. Keep full control over your projects and data.
Data labeling services
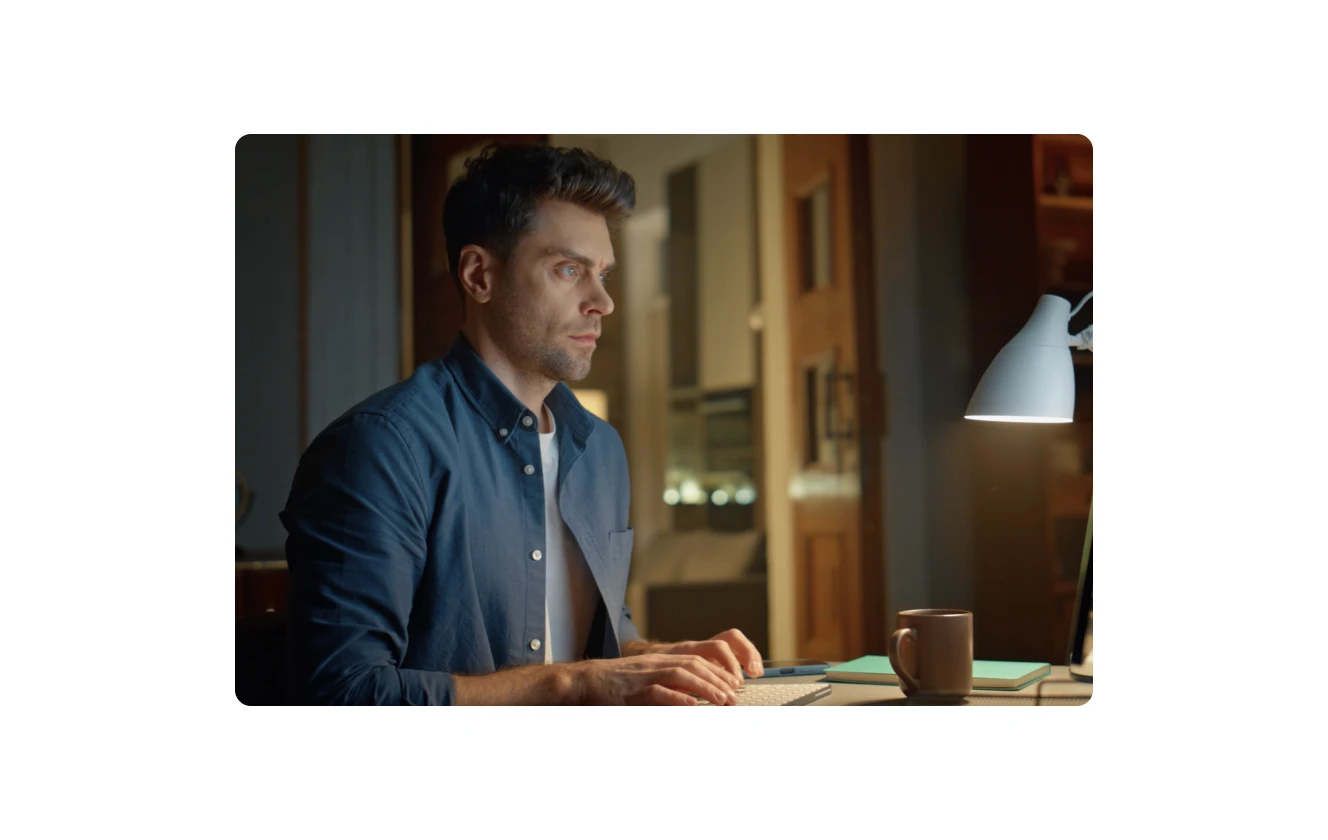
Scale your annotation efforts with on-demand professional labeling services. Access our network of trained annotators with expertise in various domains, from healthcare to linguistics. Keep full control over your projects and data.
Secure and trustworthy AI.
Here's what our customers say.
Secure and trustworthy AI.
Here's what our customers say.
We conducted extensive research of annotation tools and ultimately chose V7 as it fits our business needs best due to its customizable workflows, simple setup, consensus stages, and the ability to monitor annotator performance and project progress.
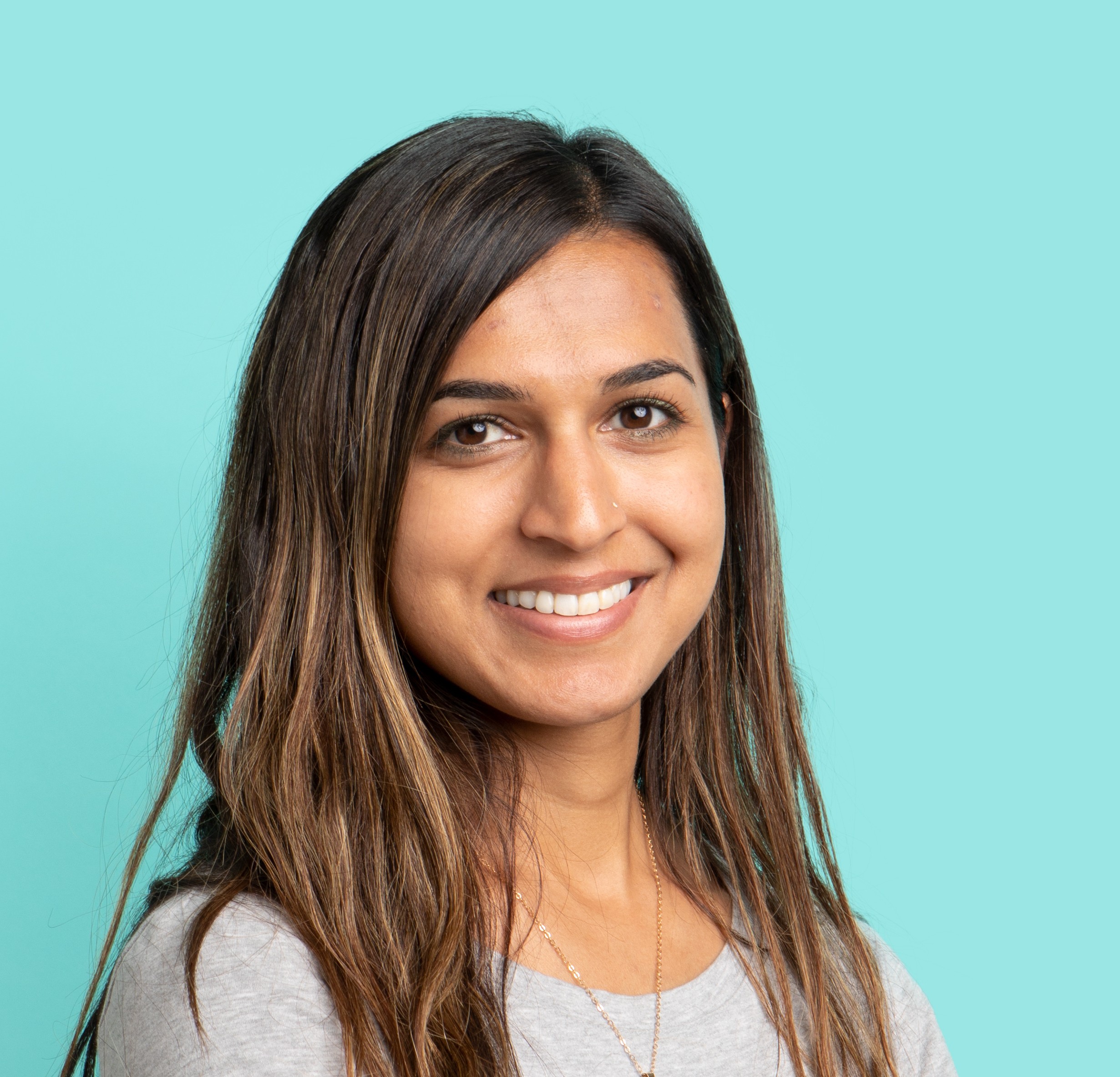
Manager of Quality and Data Curation at Imidex
Read story
→
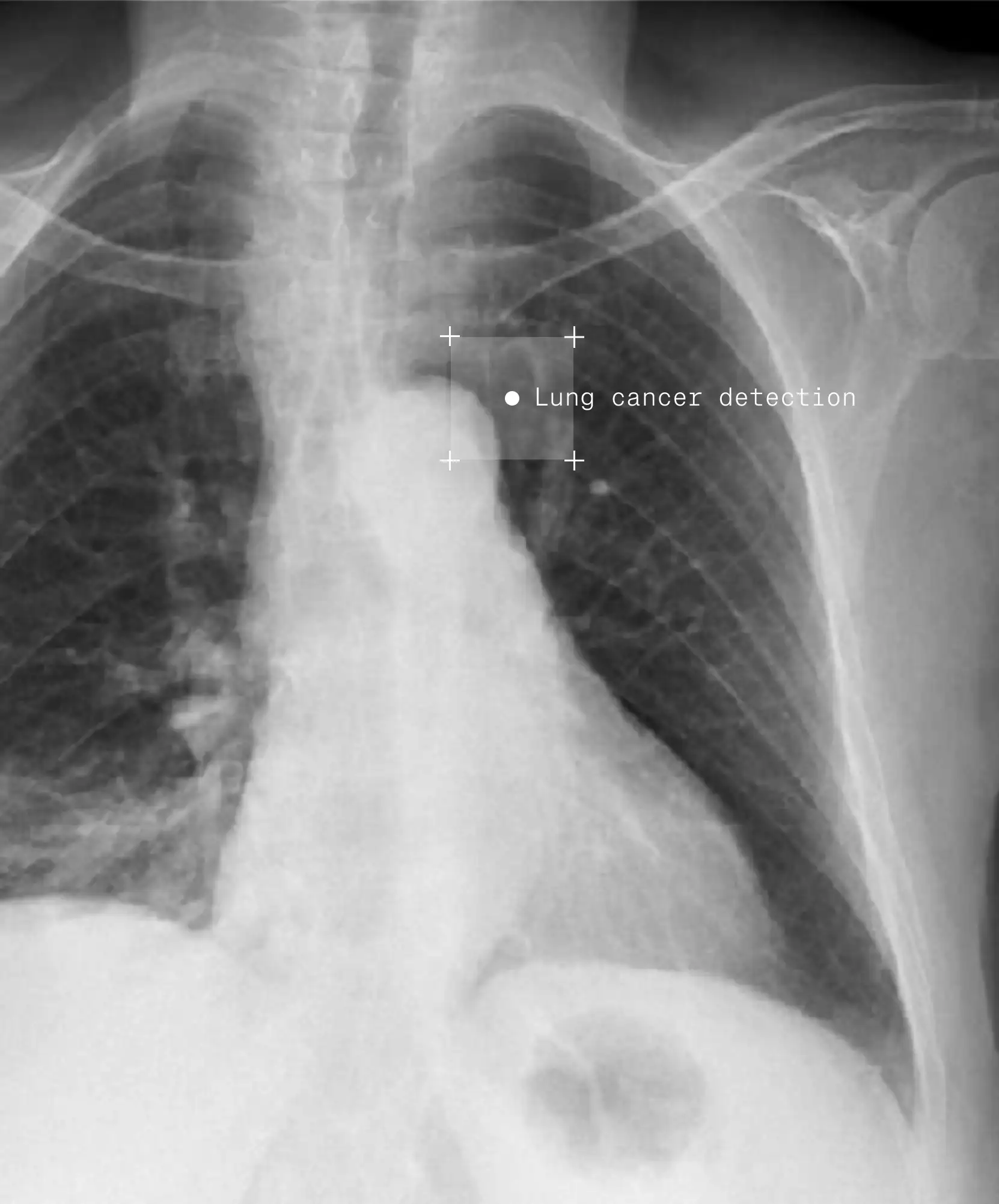
We were looking for an annotation tool that would be much faster, and V7 sped up our labeling 9–10x compared to VGG. The appeal of using V7 is that it’s commercial off-the-shelf, very intuitive, and easy to use for non-technical people involved in our project.
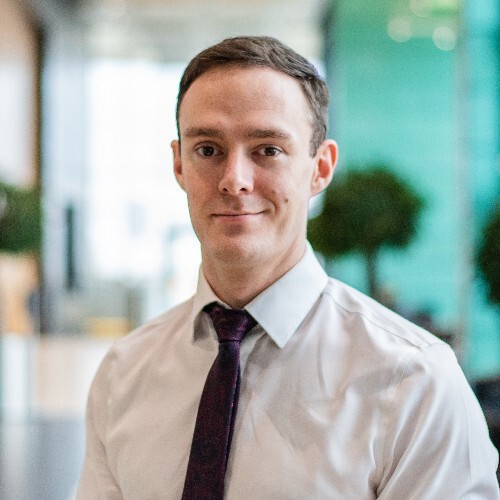
Technical Specialist at MTC
Read story
→
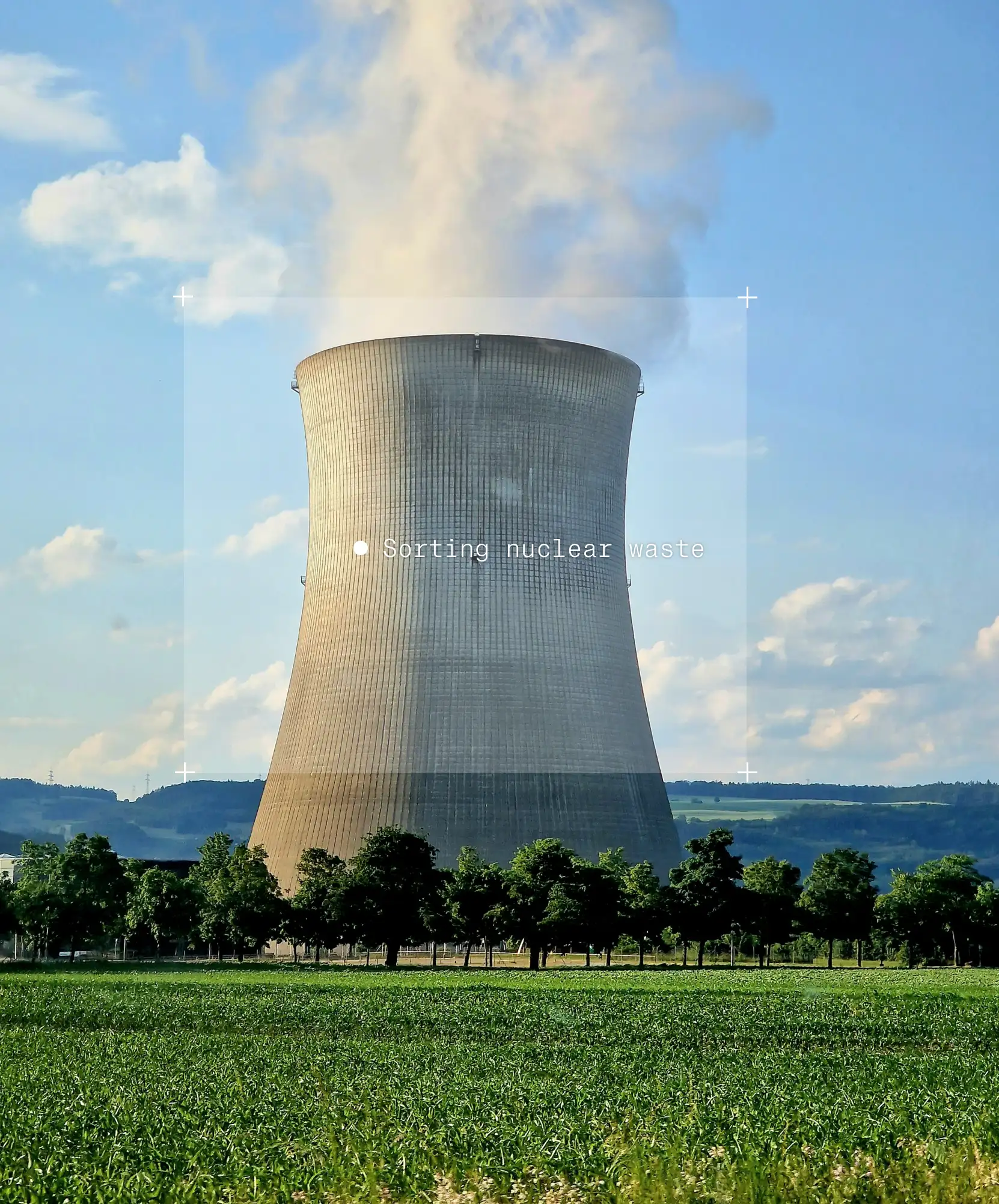
My favorite feature is the API because it automated everything for us. I like automation and being able to put things into Darwin without ever having to look at it and just review things later.
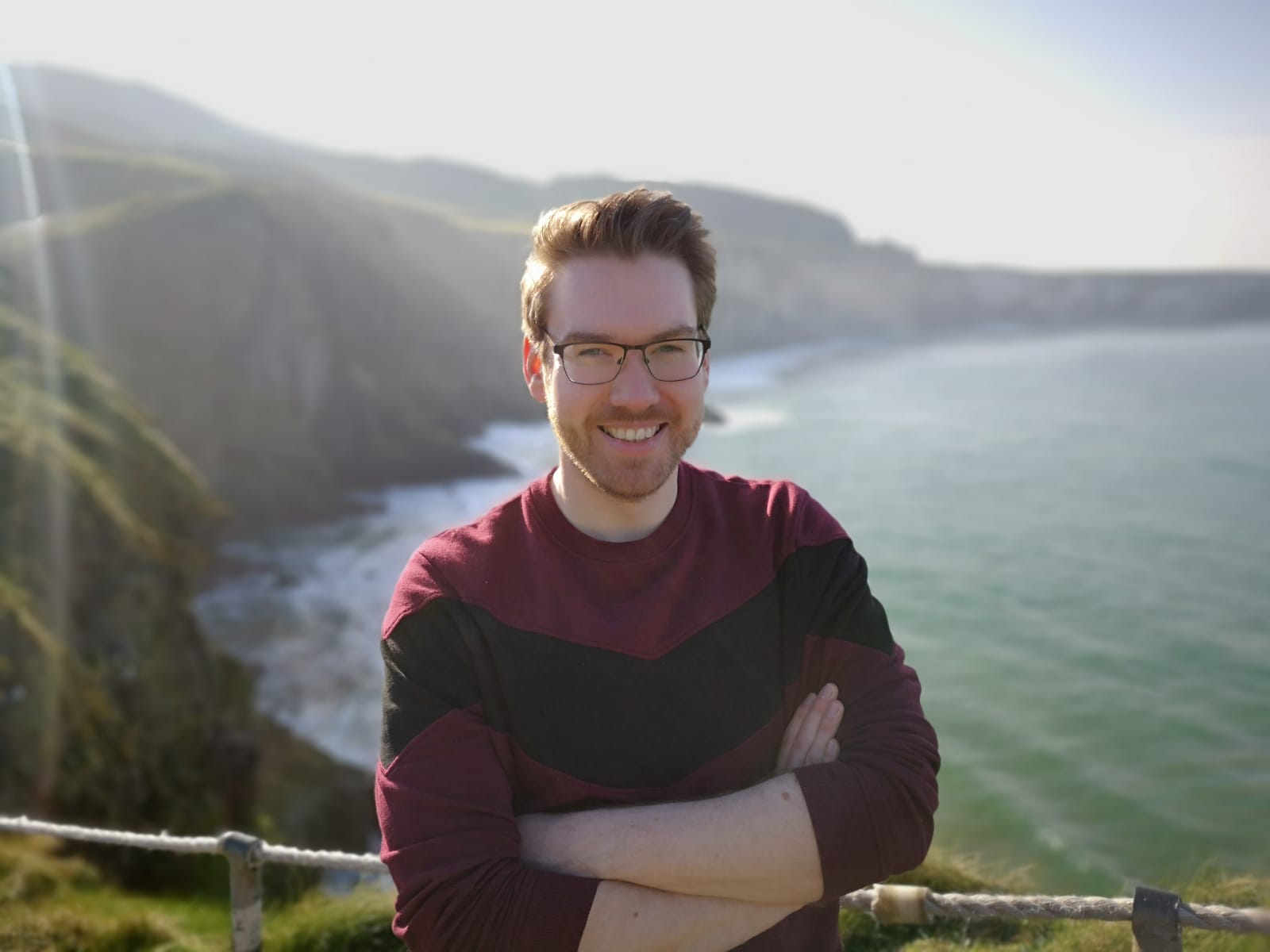
Lead Data Engineer at CattleEye
Read story
→
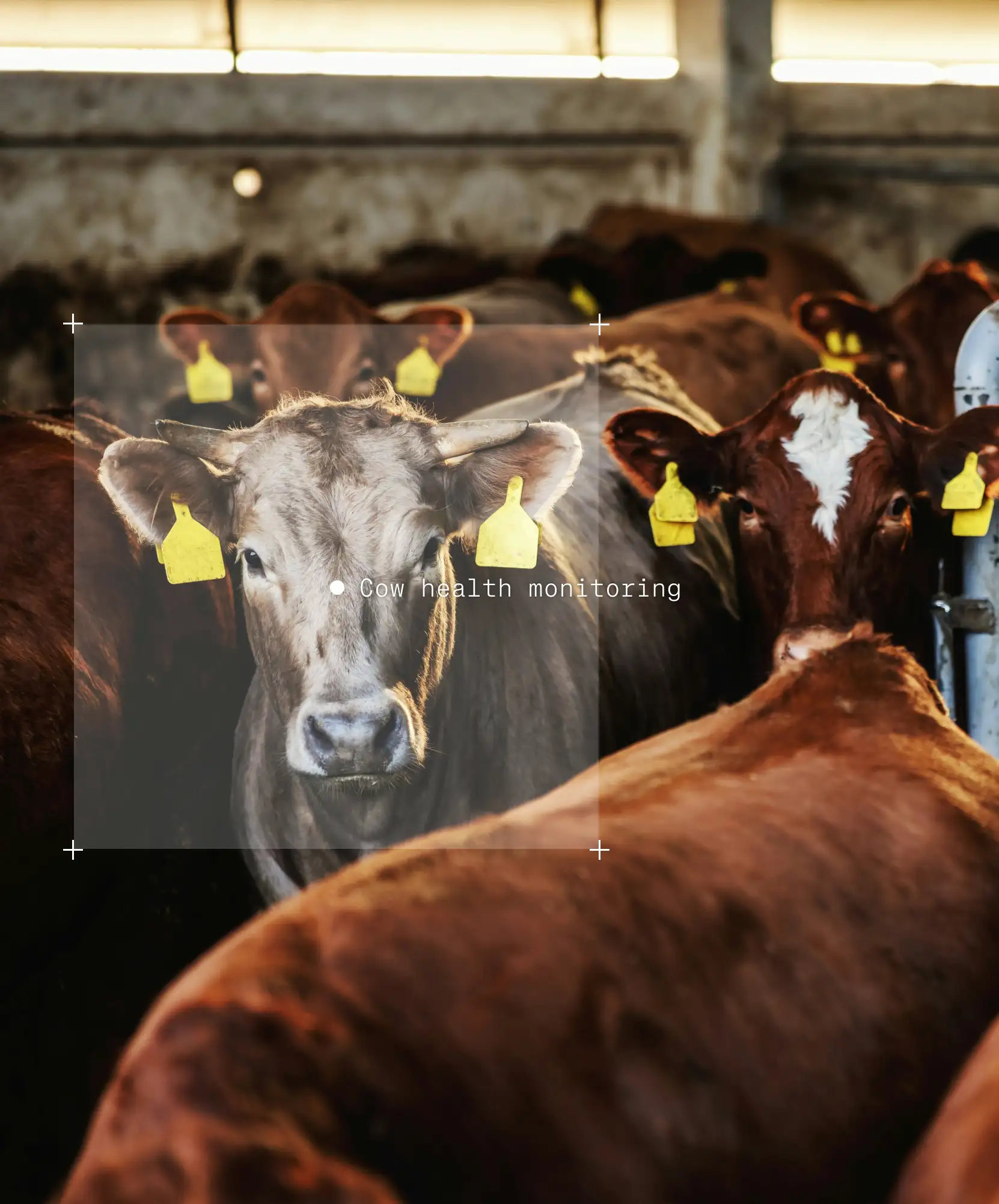
We conducted extensive research of annotation tools and ultimately chose V7 as it fits our business needs best due to its customizable workflows, simple setup, consensus stages, and the ability to monitor annotator performance and project progress.
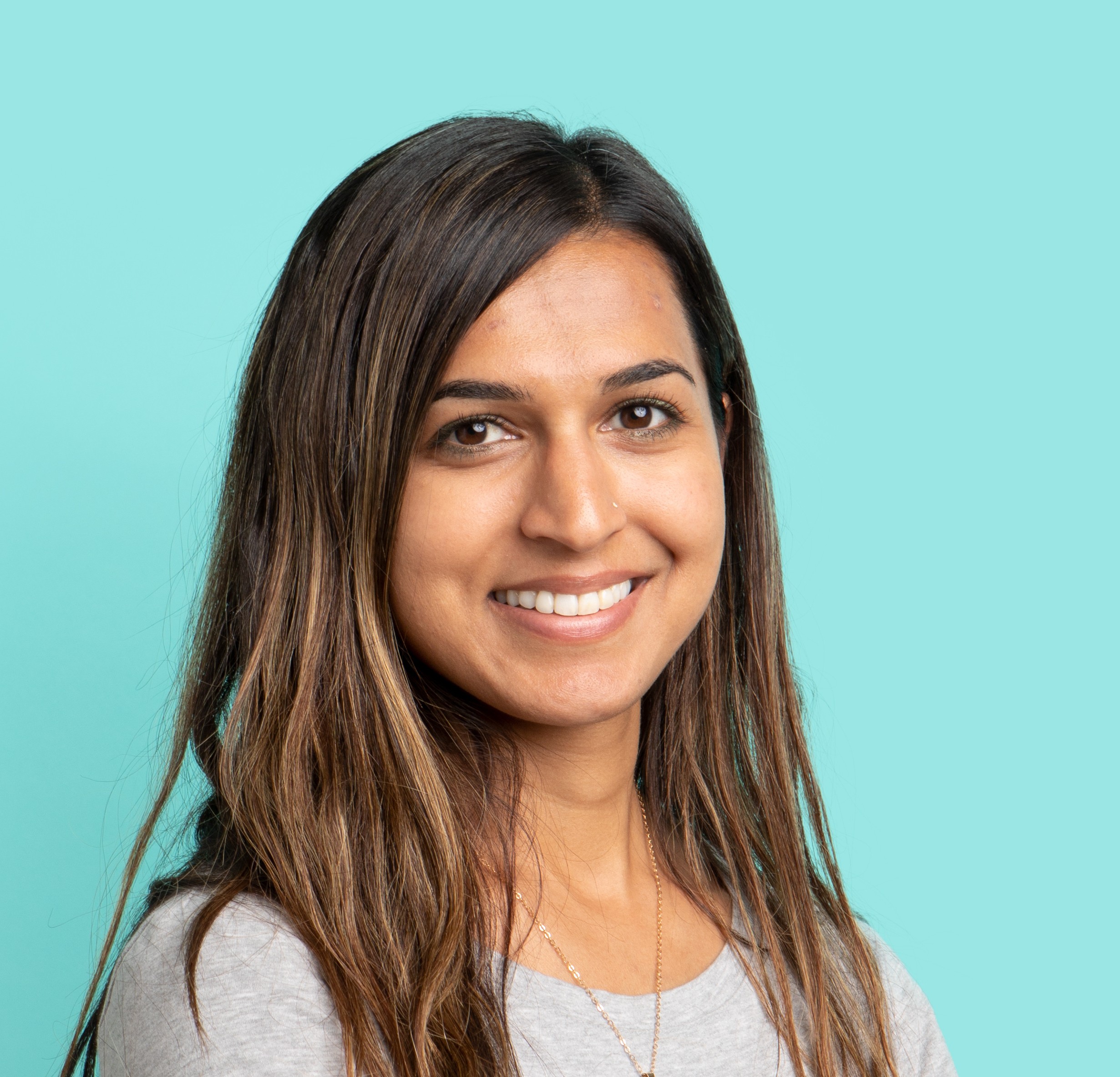
Manager of Quality and Data Curation at Imidex
Read story
→
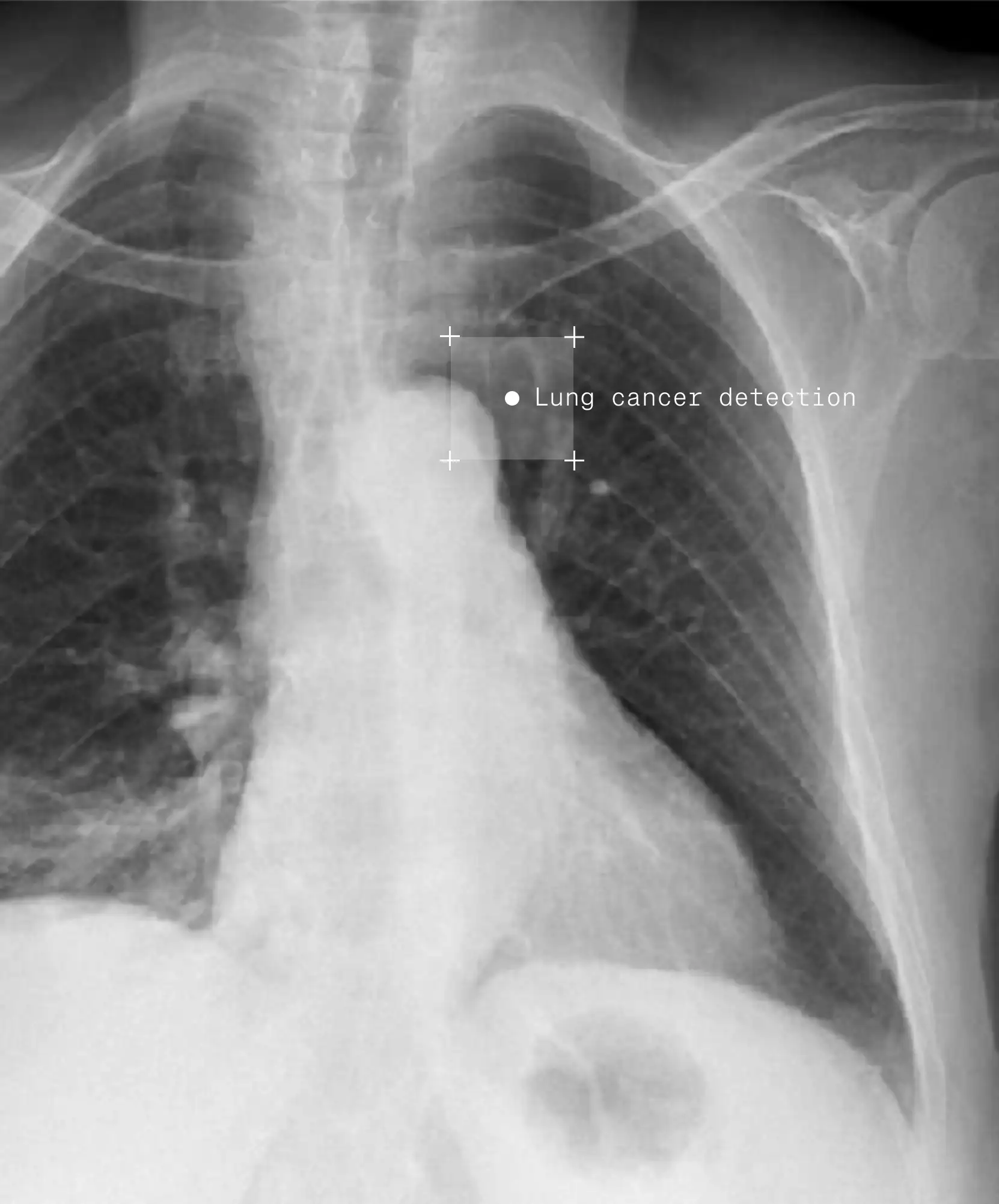
We were looking for an annotation tool that would be much faster, and V7 sped up our labeling 9–10x compared to VGG. The appeal of using V7 is that it’s commercial off-the-shelf, very intuitive, and easy to use for non-technical people involved in our project.
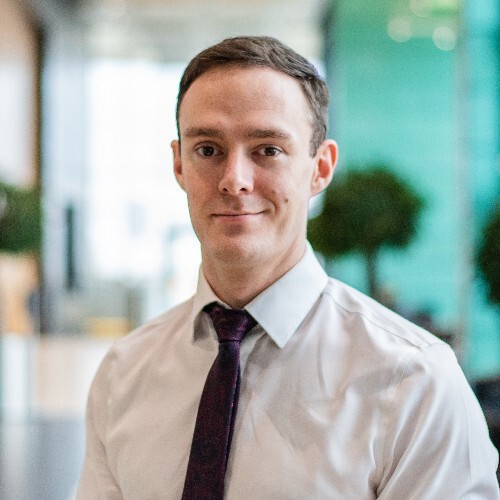
Technical Specialist at MTC
Read story
→
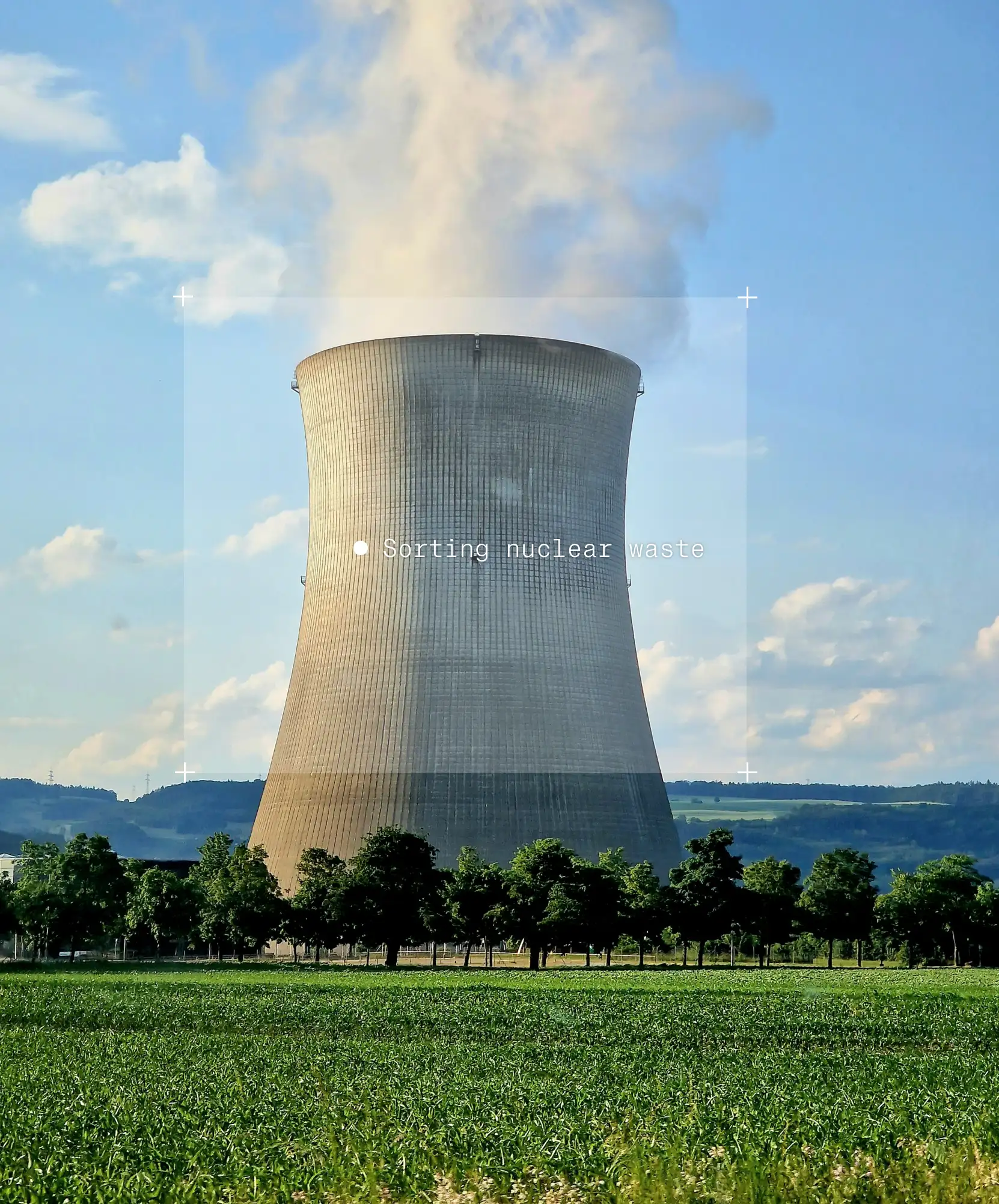
My favorite feature is the API because it automated everything for us. I like automation and being able to put things into Darwin without ever having to look at it and just review things later.
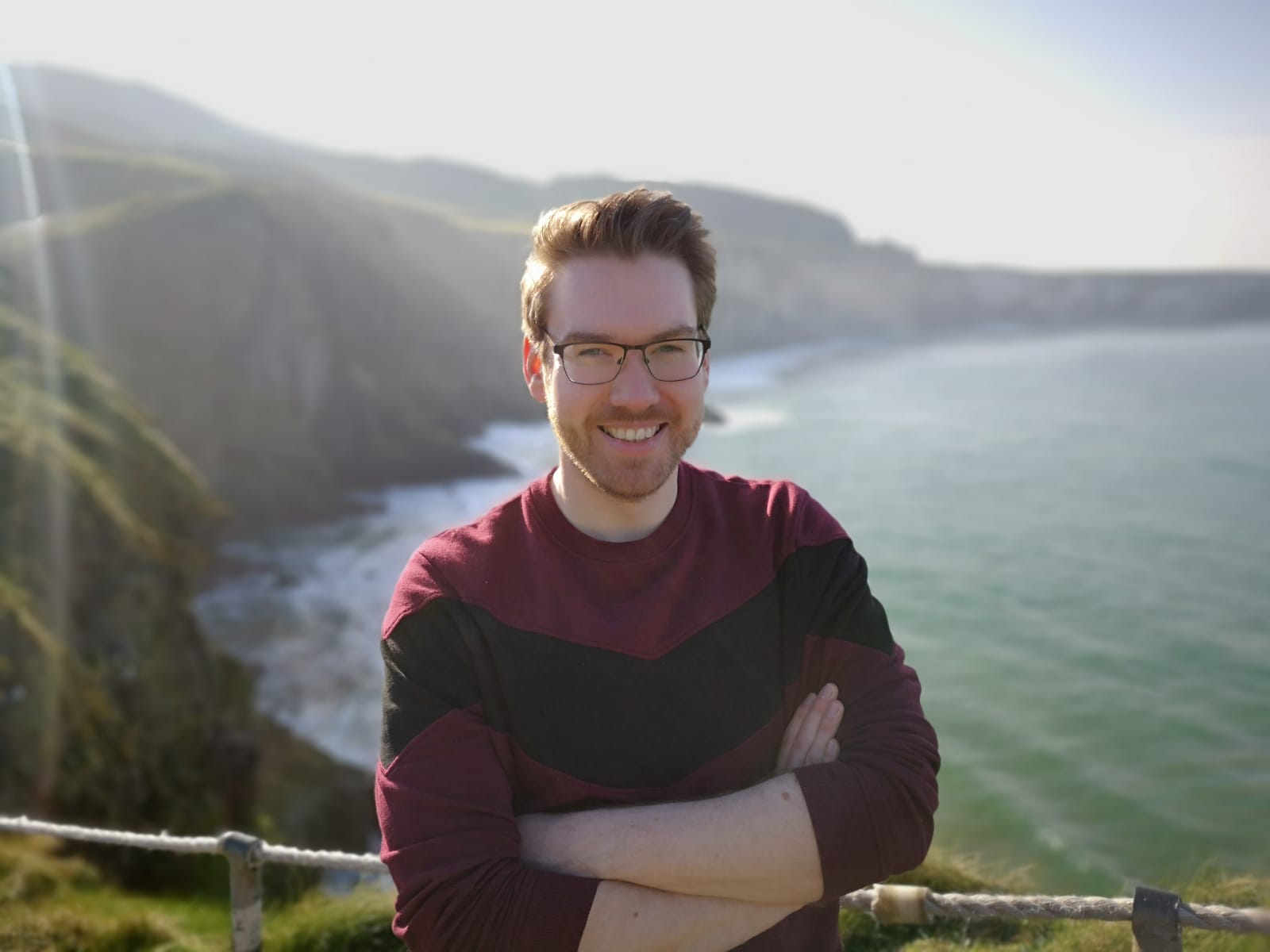
Lead Data Engineer at CattleEye
Read story
→
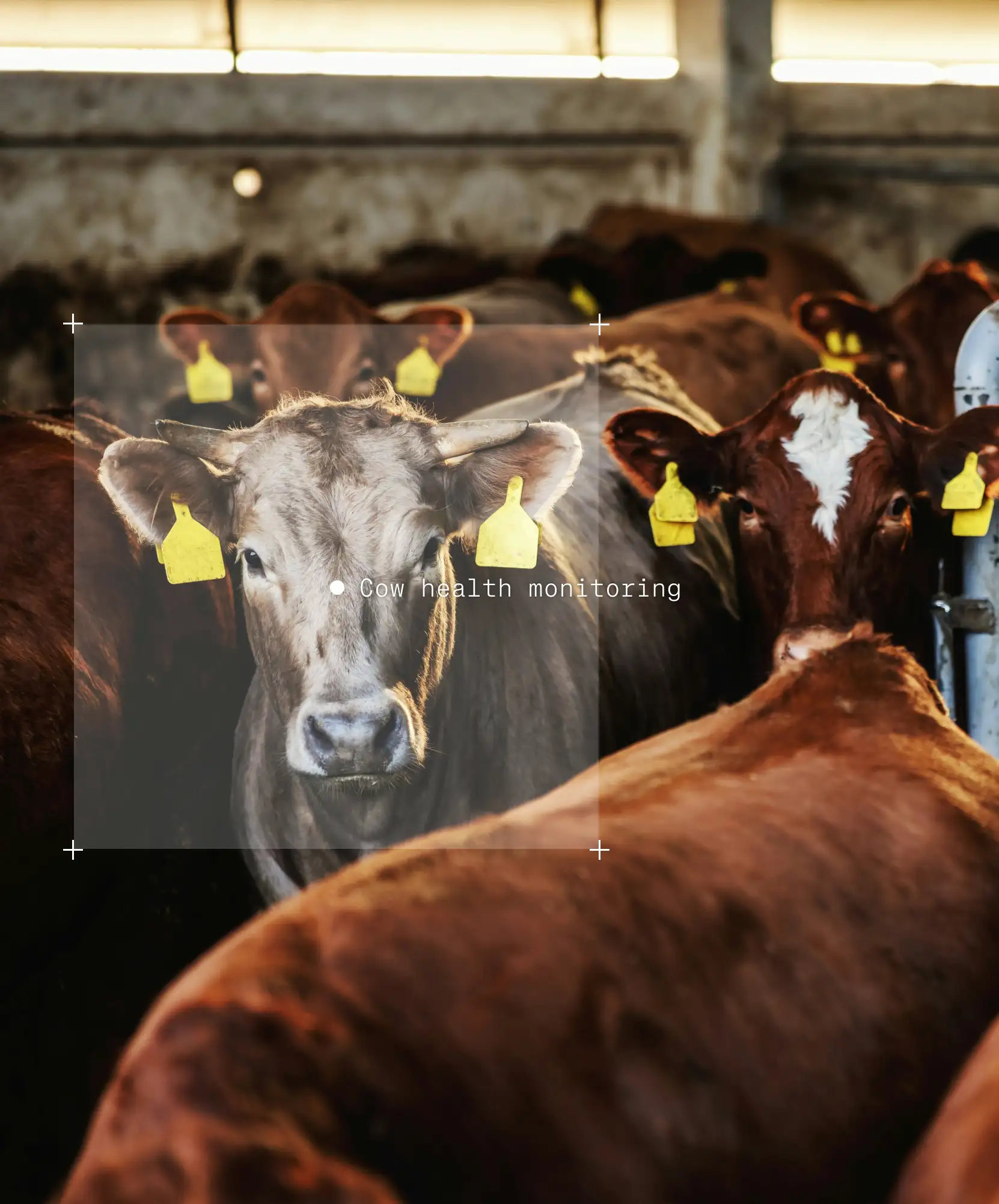
We conducted extensive research of annotation tools and ultimately chose V7 as it fits our business needs best due to its customizable workflows, simple setup, consensus stages, and the ability to monitor annotator performance and project progress.
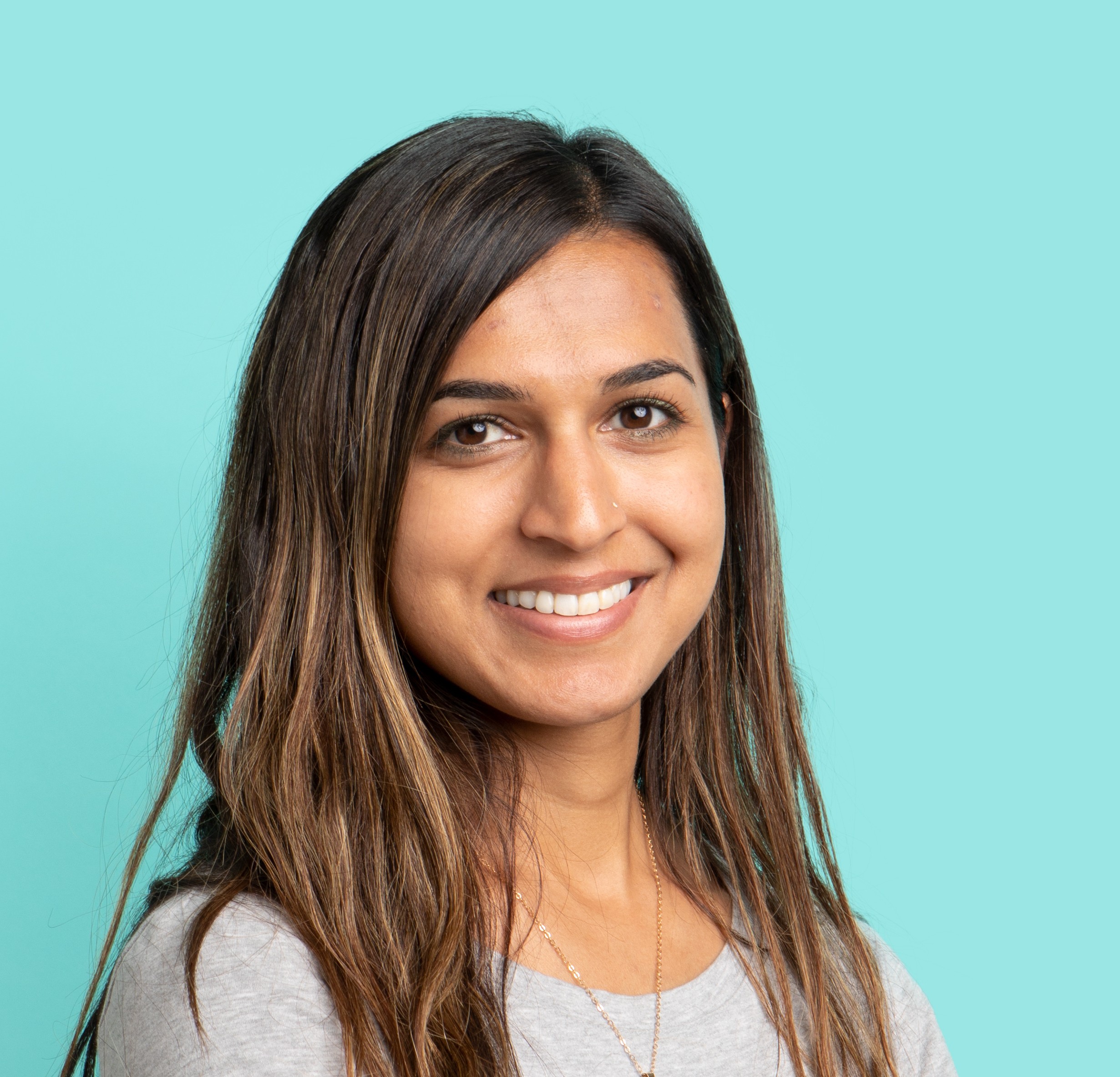
Manager of Quality and Data Curation at Imidex
Read story
→
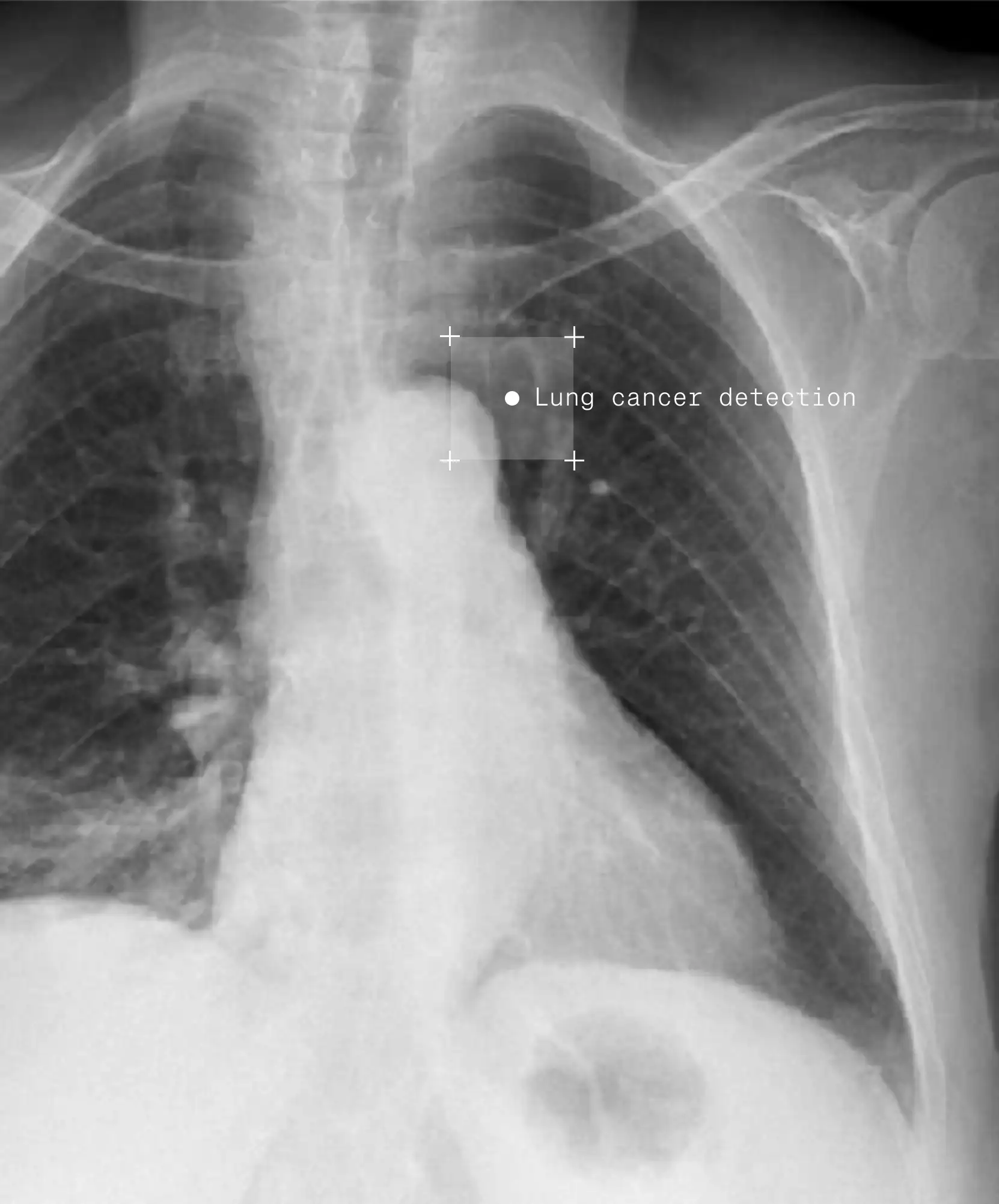
We were looking for an annotation tool that would be much faster, and V7 sped up our labeling 9–10x compared to VGG. The appeal of using V7 is that it’s commercial off-the-shelf, very intuitive, and easy to use for non-technical people involved in our project.
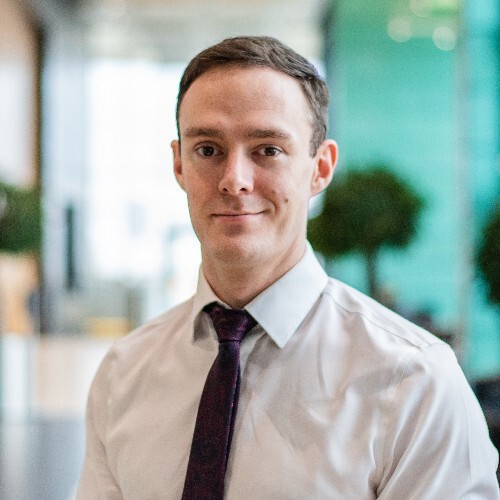
Technical Specialist at MTC
Read story
→
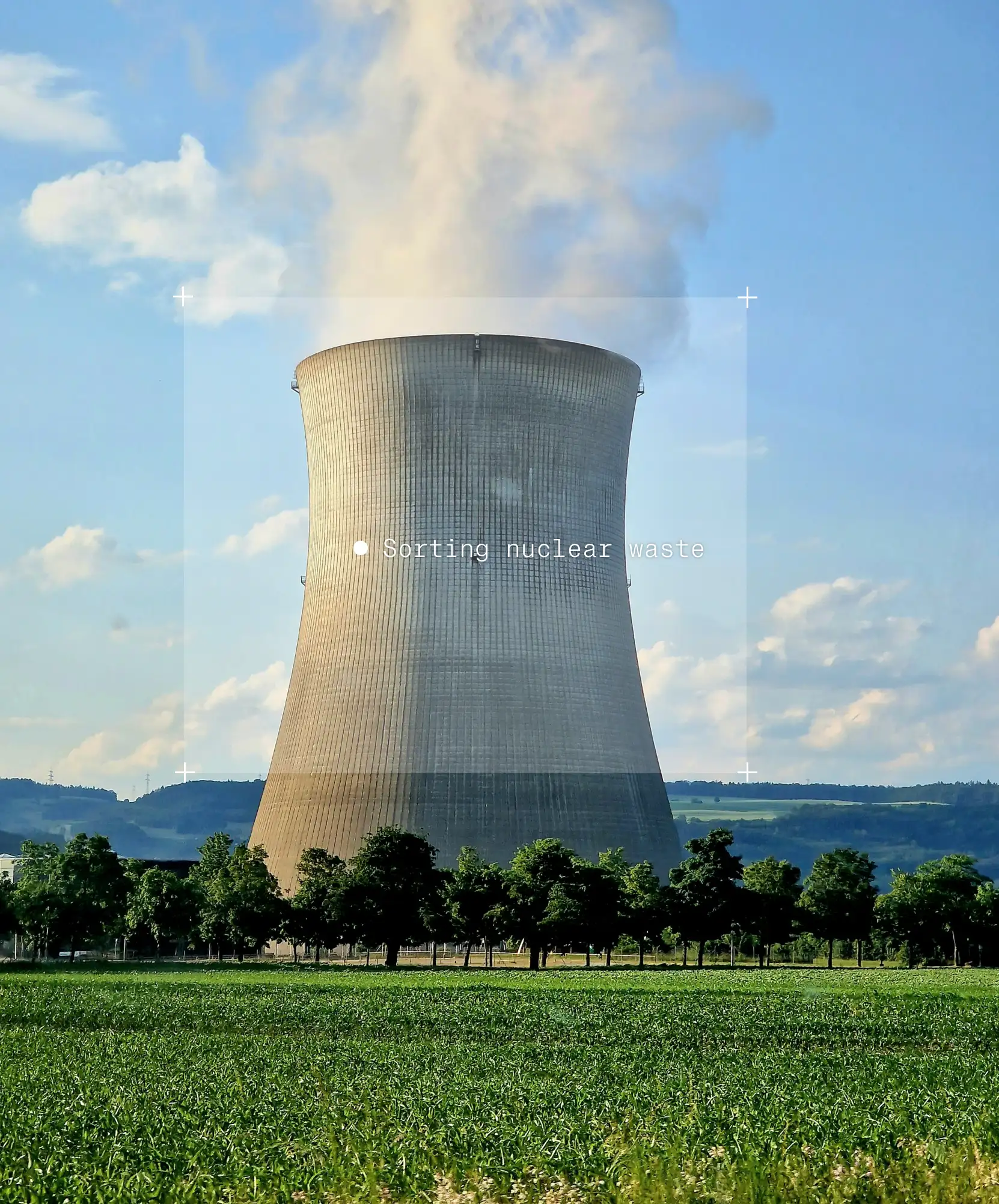
My favorite feature is the API because it automated everything for us. I like automation and being able to put things into Darwin without ever having to look at it and just review things later.
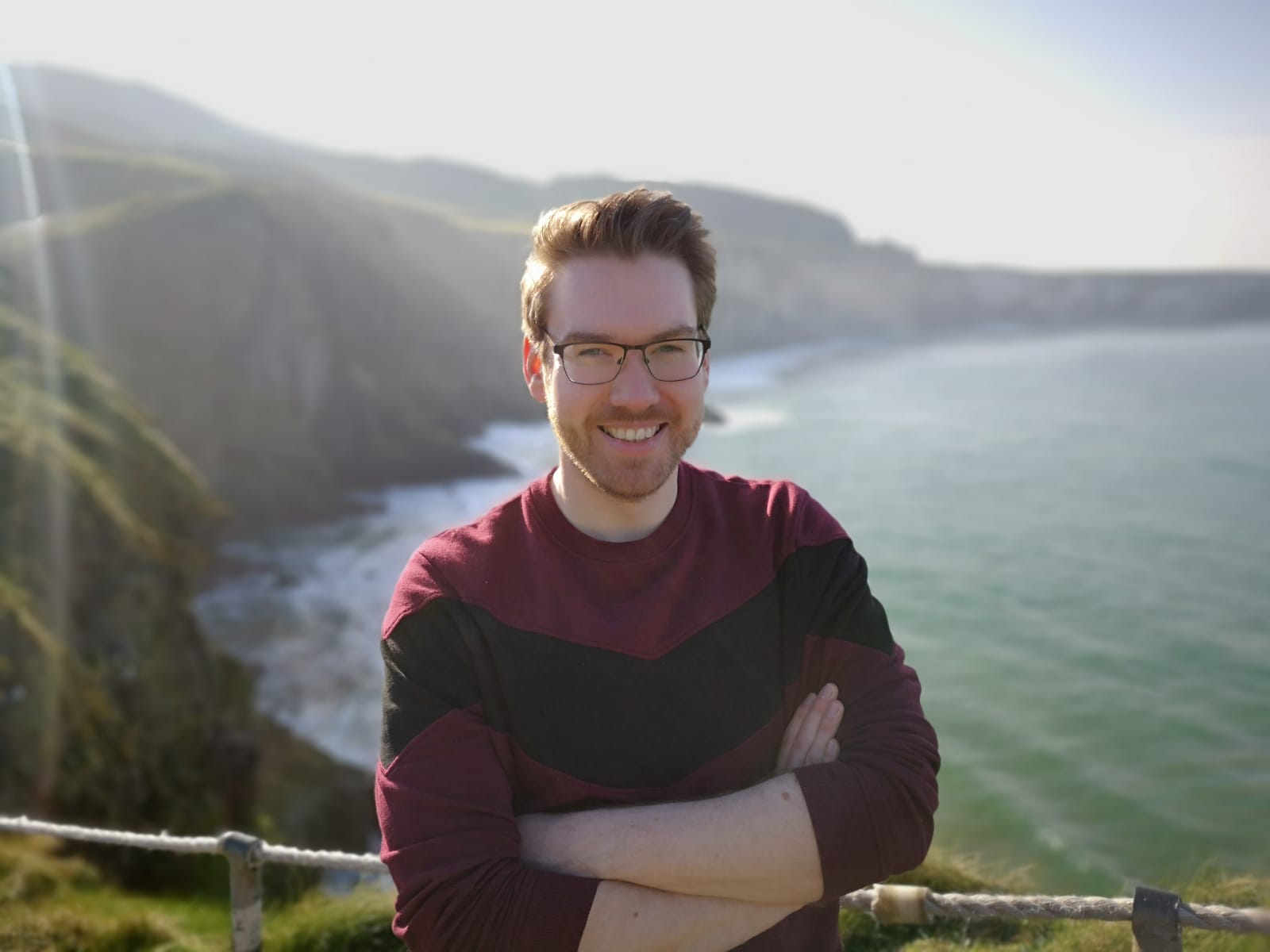
Lead Data Engineer at CattleEye
Read story
→
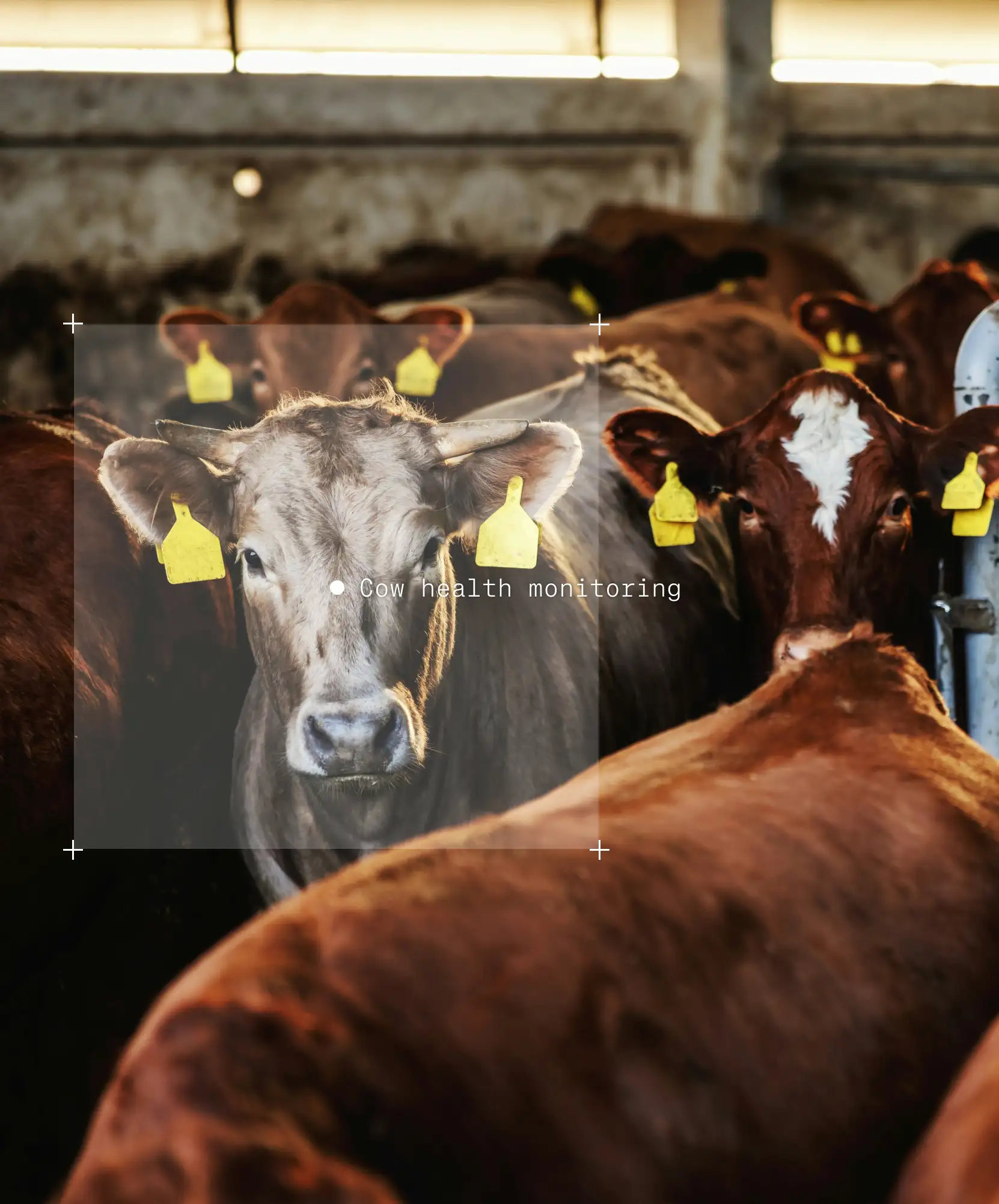
Security & Integrations
Security & Integrations
Connect your data with confidence.
Built for ML developers.
Connect your data with confidence. Built for ML developers.
Security & Integrations
Integrate with your toolstack
Integrate V7 with your existing ML infrastructure. Use your private cloud with major providers including AWS, Google Cloud, and Azure. Export annotations in formats compatible with popular frameworks like TensorFlow and PyTorch.
Integrate with your toolstack
Integrate V7 with your existing ML infrastructure. Use your private cloud with major providers including AWS, Google Cloud, and Azure. Export annotations in formats compatible with popular frameworks like TensorFlow and PyTorch.
Integrate with your toolstack
Integrate V7 with your existing ML infrastructure. Use your private cloud with major providers including AWS, Google Cloud, and Azure. Export annotations in formats compatible with popular frameworks like TensorFlow and PyTorch.
Built with security in mind
Benefit from SOC 2 Type II and HIPAA-compliant infrastructure. Set granular access controls and track all actions with detailed logs. Meet stringent security standards for industries like healthcare and finance.
Built with security in mind
Benefit from SOC 2 Type II and HIPAA-compliant infrastructure. Set granular access controls and track all actions with detailed logs. Meet stringent security standards for industries like healthcare and finance.
Built with security in mind
Benefit from SOC 2 Type II and HIPAA-compliant infrastructure. Set granular access controls and track all actions with detailed logs. Meet stringent security standards for industries like healthcare and finance.
Developer friendly API & SDK
V7 is built by developers for developers. Our tools make it easy to automate workflows, customize integrations, and extend V7's capabilities to fit your unique use cases. Enjoy clear documentation, code samples, and responsive support.
Developer friendly API & SDK
V7 is built by developers for developers. Our tools make it easy to automate workflows, customize integrations, and extend V7's capabilities to fit your unique use cases. Enjoy clear documentation, code samples, and responsive support.
Developer friendly API & SDK
V7 is built by developers for developers. Our tools make it easy to automate workflows, customize integrations, and extend V7's capabilities to fit your unique use cases. Enjoy clear documentation, code samples, and responsive support.
Built for scale
Handle projects of any size. Create multiple workspaces and invite users to collaborate. Manage and filter datasets with intuitive GUI dashboards. Set up custom views, add tags, and save advanced filtering conditions.
Built for scale
Handle projects of any size. Create multiple workspaces and invite users to collaborate. Manage and filter datasets with intuitive GUI dashboards. Set up custom views, add tags, and save advanced filtering conditions.
Built for scale
Handle projects of any size. Create multiple workspaces and invite users to collaborate. Manage and filter datasets with intuitive GUI dashboards. Set up custom views, add tags, and save advanced filtering conditions.
Next steps
Tell us more about your project.
Let's build a proof of concept.
Try our free tier or talk to one of our experts.
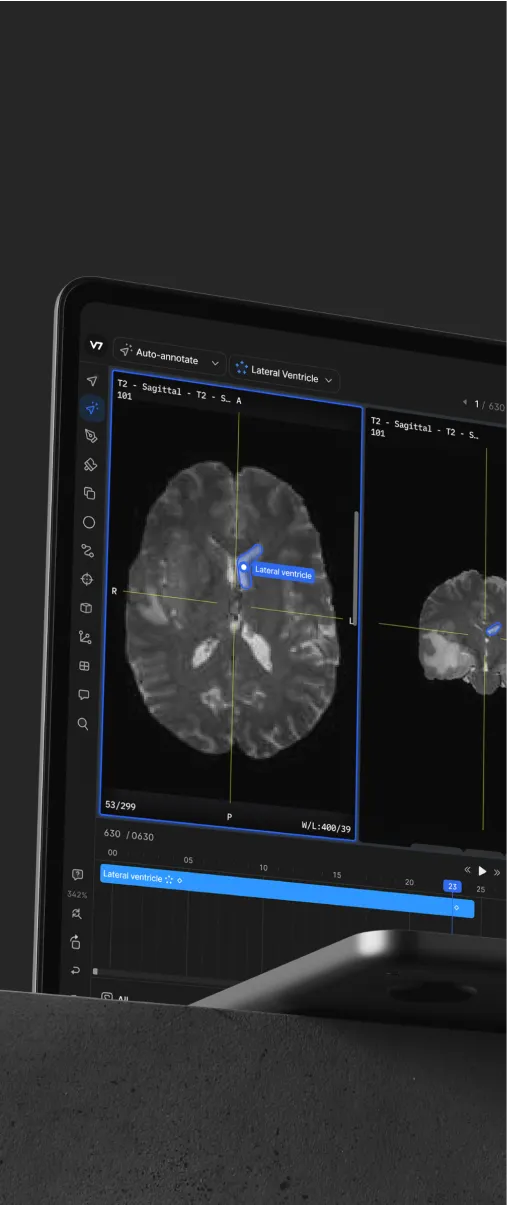
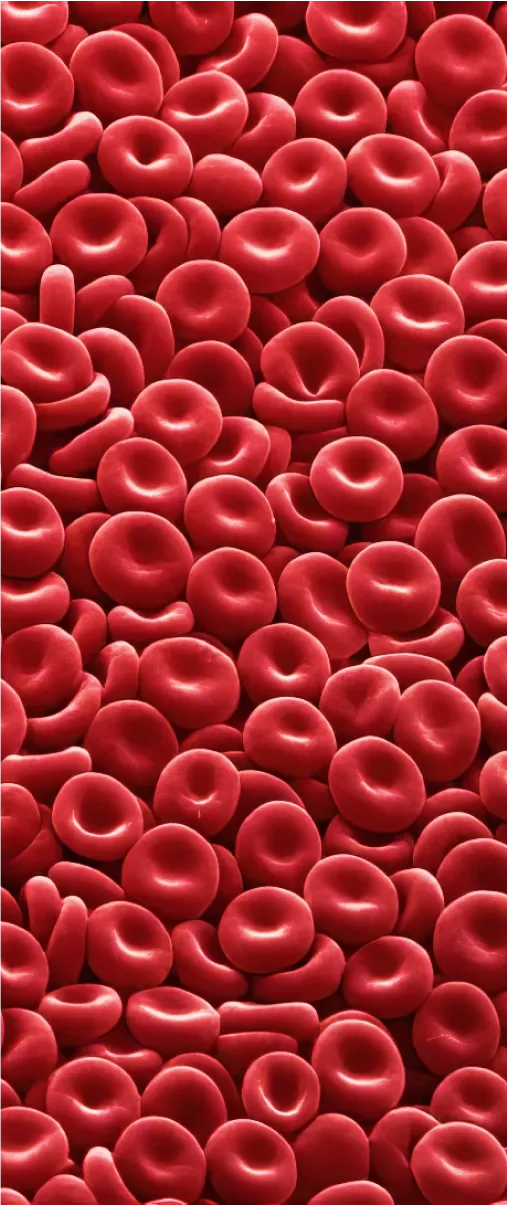
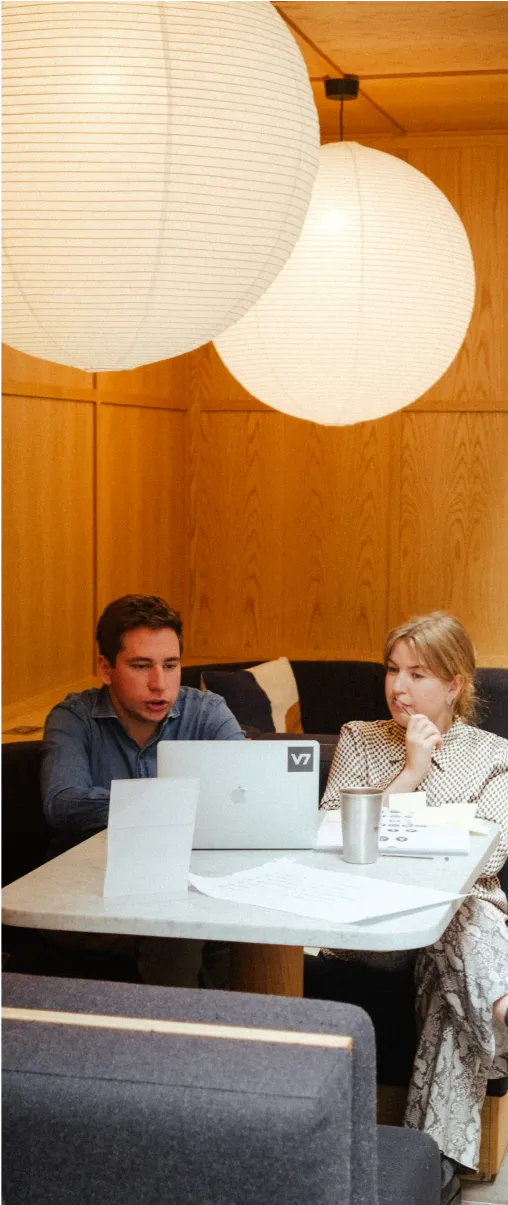
Next steps
Tell us more about your project.
Let's build a proof of concept.
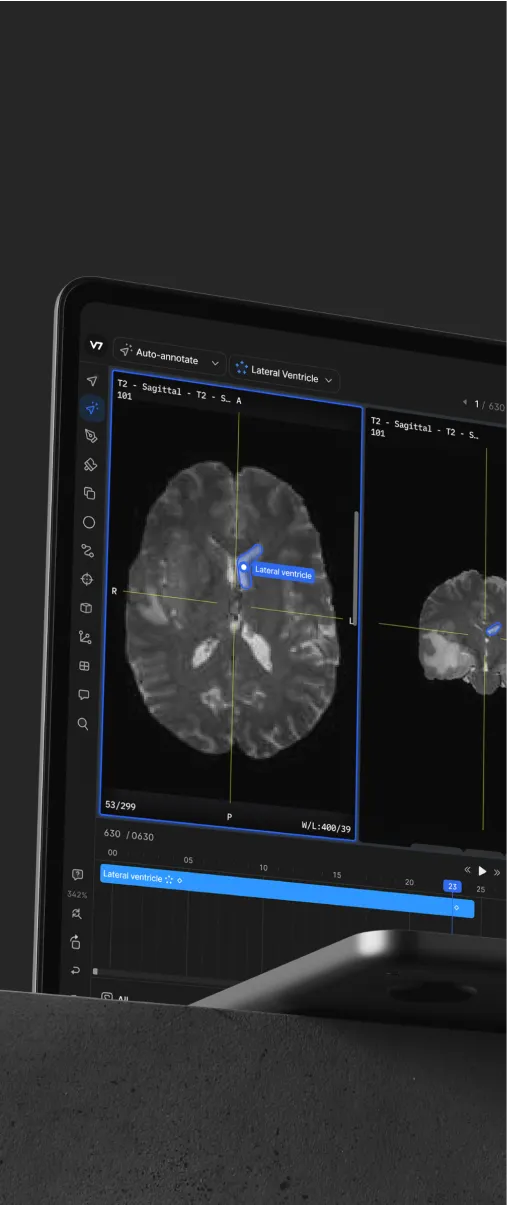
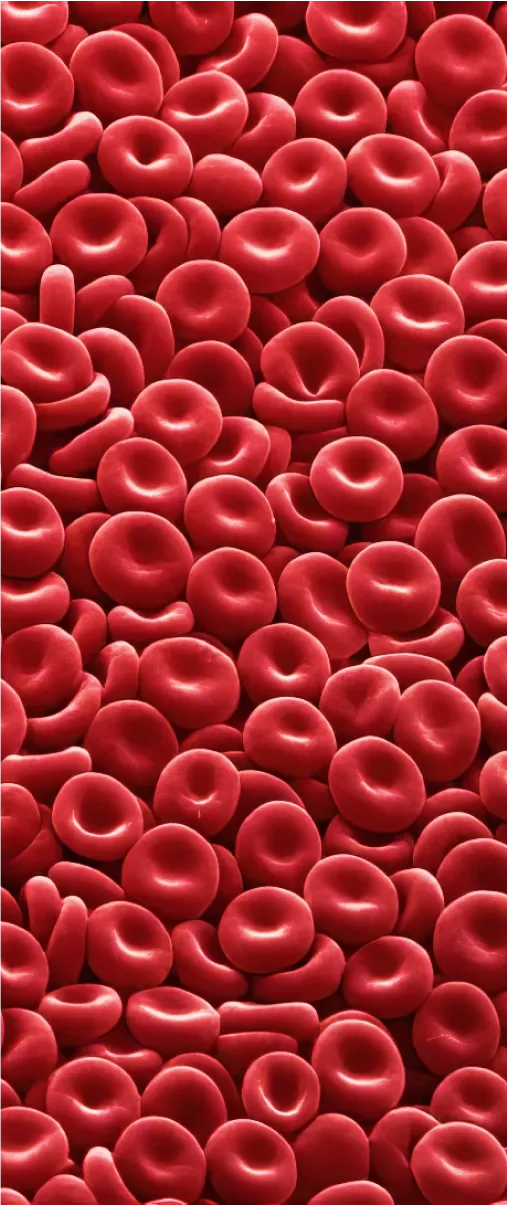
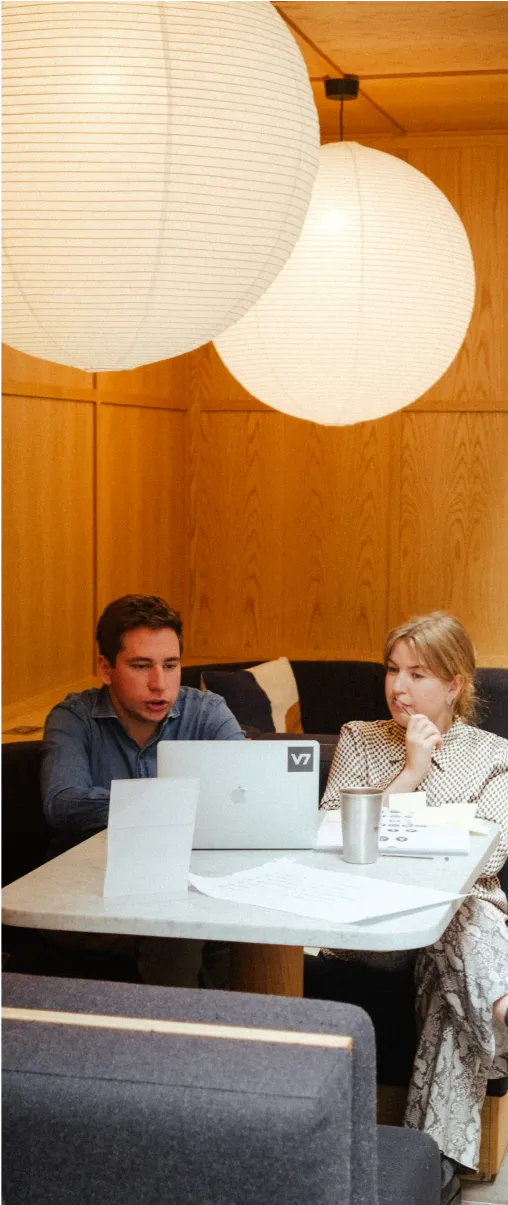