Medical AI
Medical AI
Medical AI
Boost medical AI development
in all modalities by 80%.
Boost medical AI development in all modalities by 80%.
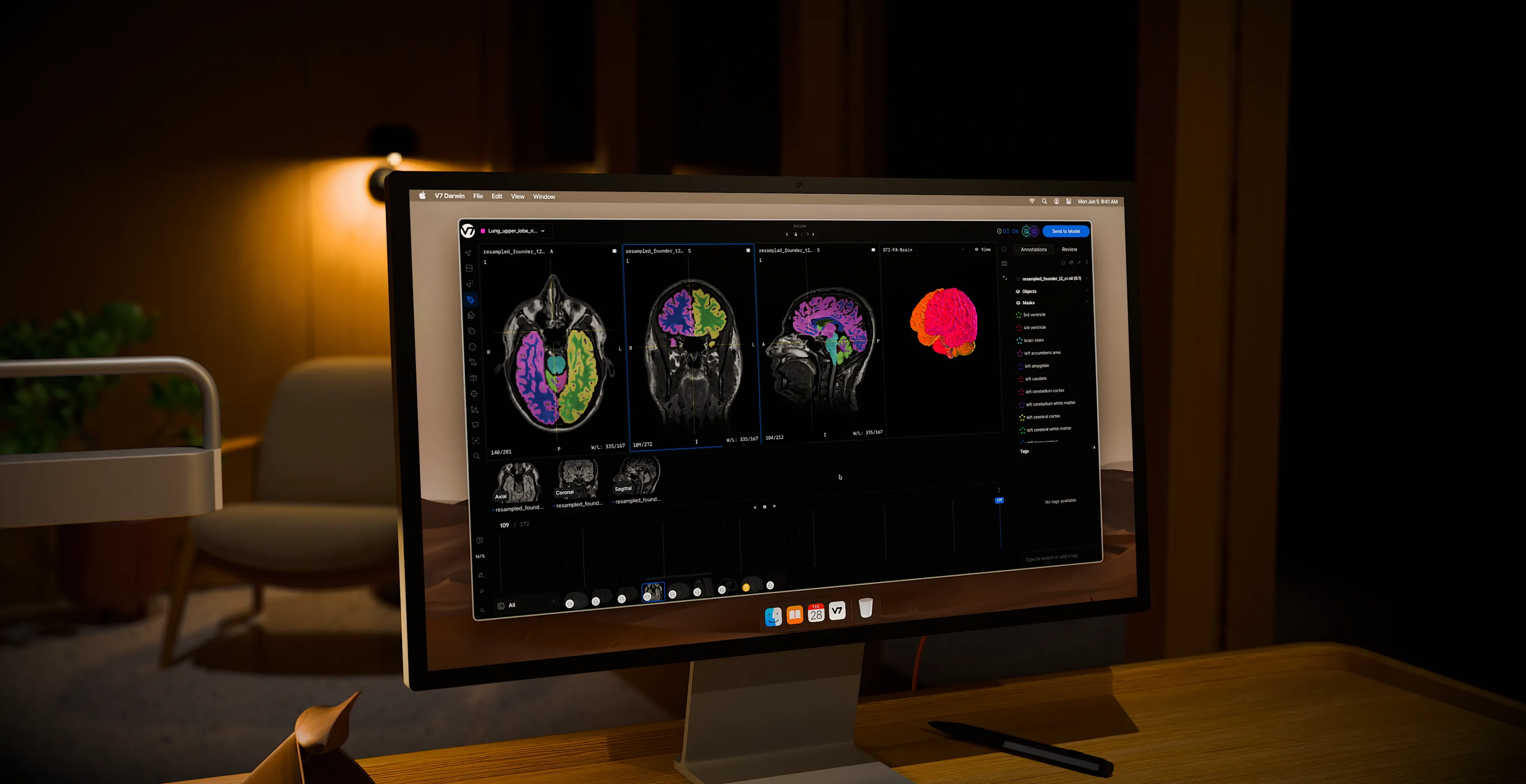

Use cases
Use cases
Enabling life-changing breakthroughs.
V7 powers the best healthcare companies.
Use cases
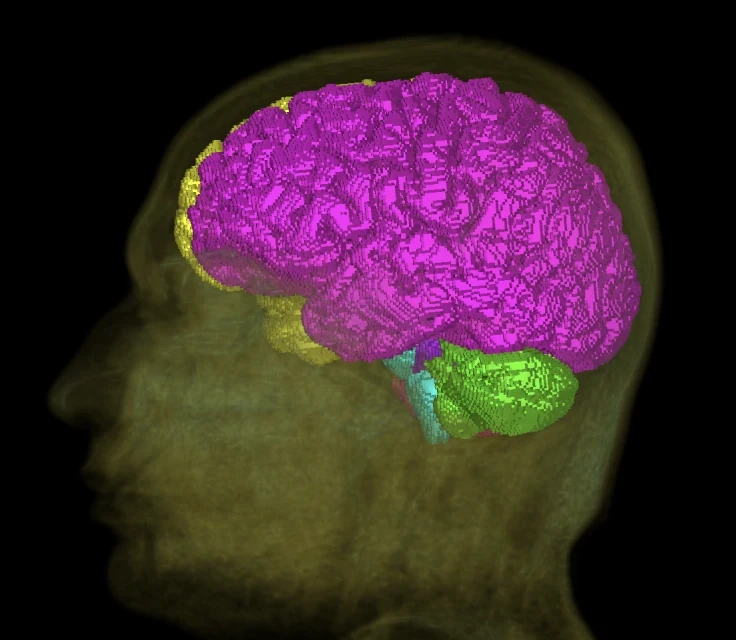
CT and MRI
Healthcare companies use V7 to build AI for breast cancer detection, lung nodule identification, and more.
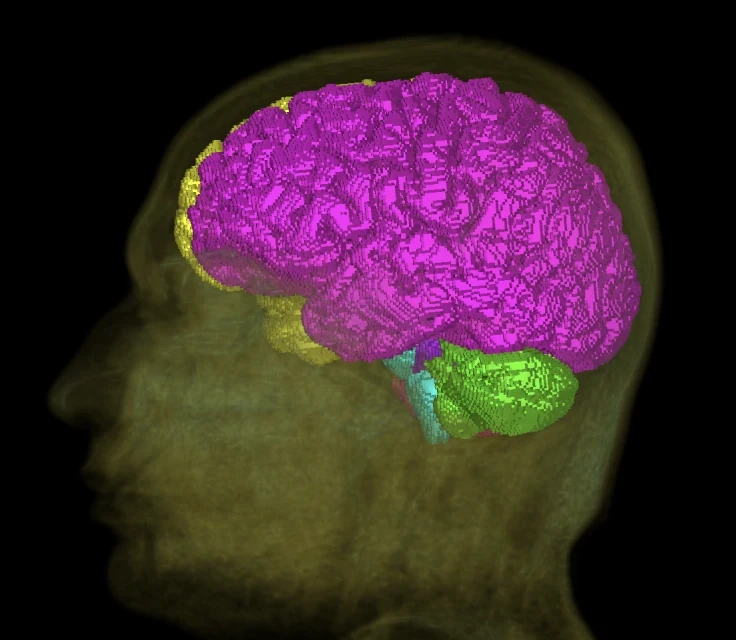
CT and MRI
Healthcare companies use V7 to build AI for breast cancer detection, lung nodule identification, and more.
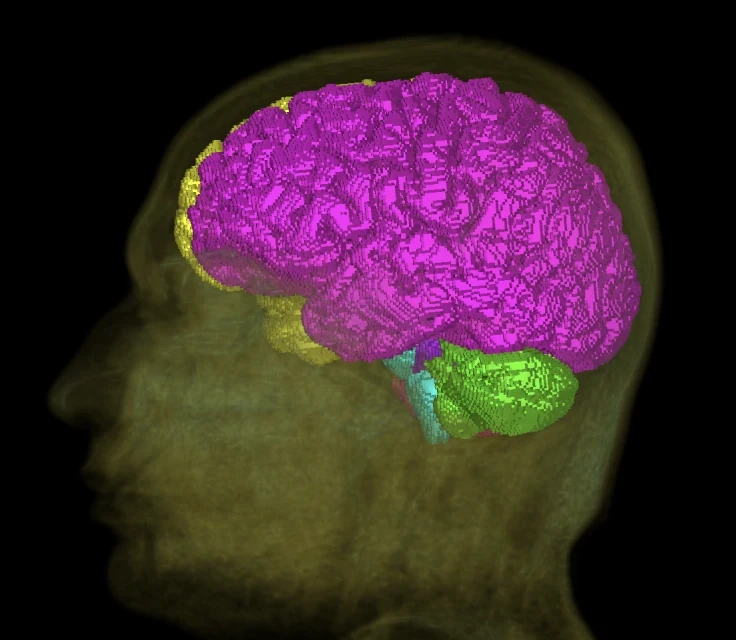
CT and MRI
Healthcare companies use V7 to build AI for breast cancer detection, lung nodule identification, and more.
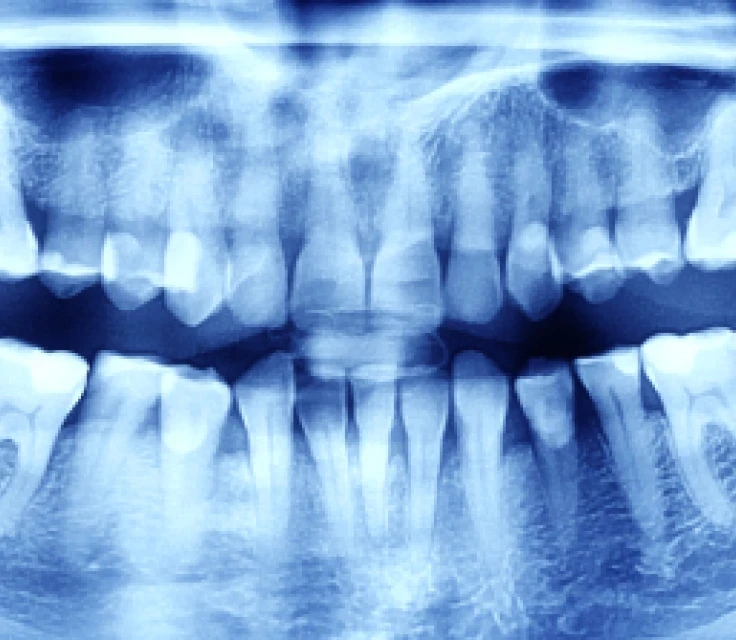
X-rays
Healthcare companies use V7 to build AI for identifying bone fractures, dental caries in panoramic X-rays, and more.
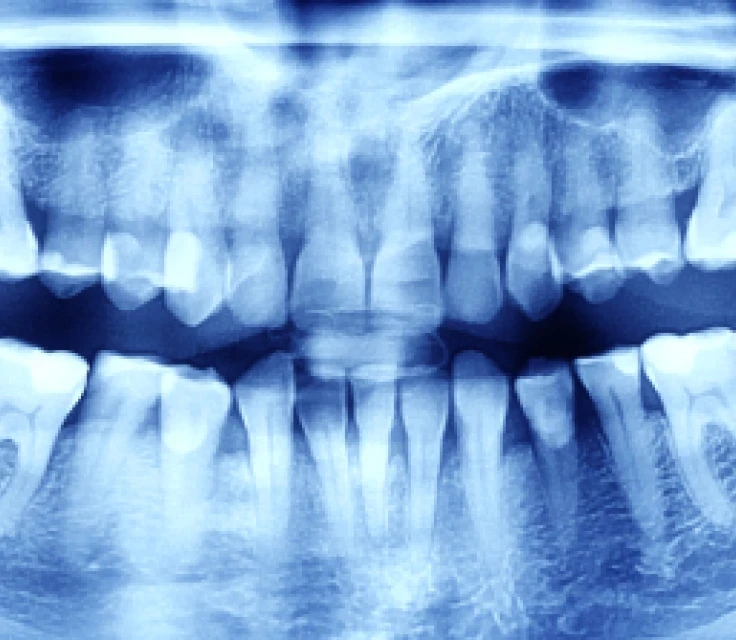
X-rays
Healthcare companies use V7 to build AI for identifying bone fractures, dental caries in panoramic X-rays, and more.
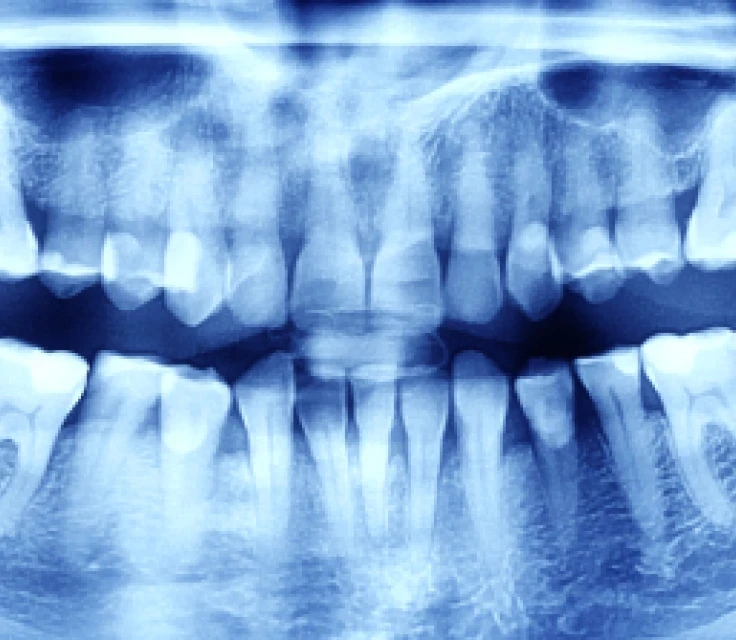
X-rays
Healthcare companies use V7 to build AI for identifying bone fractures, dental caries in panoramic X-rays, and more.
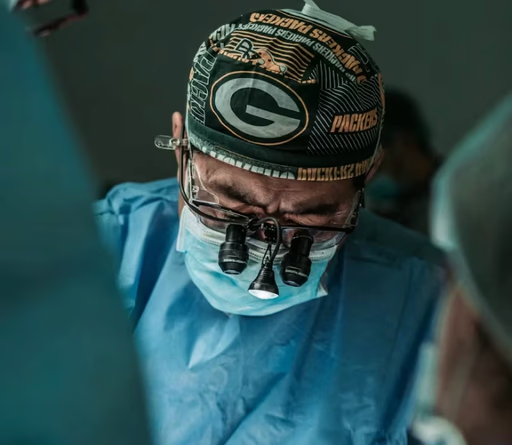
Surgery
Healthcare companies use V7 to build AI for smart endoscopic surgery tools, fluoroscopy, and more.
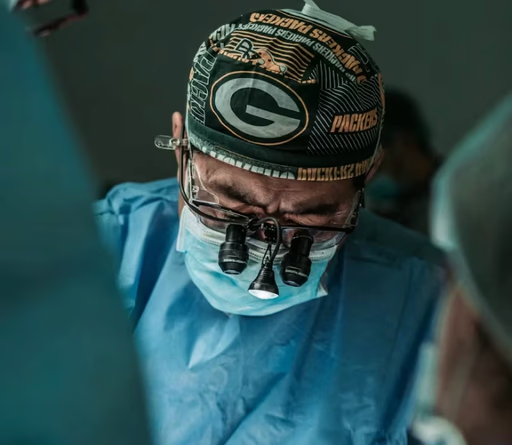
Surgery
Healthcare companies use V7 to build AI for smart endoscopic surgery tools, fluoroscopy, and more.
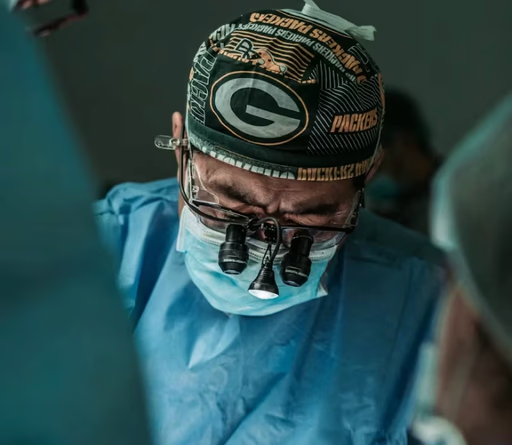
Surgery
Healthcare companies use V7 to build AI for smart endoscopic surgery tools, fluoroscopy, and more.

Microscopy
Healthcare companies use V7 to build AI for cell counting, classification in pathology slides, live cell imaging, and more.

Microscopy
Healthcare companies use V7 to build AI for cell counting, classification in pathology slides, live cell imaging, and more.

Microscopy
Healthcare companies use V7 to build AI for cell counting, classification in pathology slides, live cell imaging, and more.

Medical records
Healthcare companies use V7 to tag, organize, and extract data from medical records, reports, and academic papers.

Medical records
Healthcare companies use V7 to tag, organize, and extract data from medical records, reports, and academic papers.

Medical records
Healthcare companies use V7 to tag, organize, and extract data from medical records, reports, and academic papers.

Ultrasound
Healthcare companies use V7 to build AI that supports pre-transplantation examinations using ultrasound.

Ultrasound
Healthcare companies use V7 to build AI that supports pre-transplantation examinations using ultrasound.

Ultrasound
Healthcare companies use V7 to build AI that supports pre-transplantation examinations using ultrasound.
About
About
About
End-to-end AI toolkit for medical image annotation.
Accelerate high-quality training data creation and build more efficient AI workflows for healthcare.
End-to-end AI toolkit for medical image annotation. Accelerate high-quality training data creation and build more efficient AI workflows for healthcare.
Built for speed
Built for accuracy
Built for size
Built for compliance
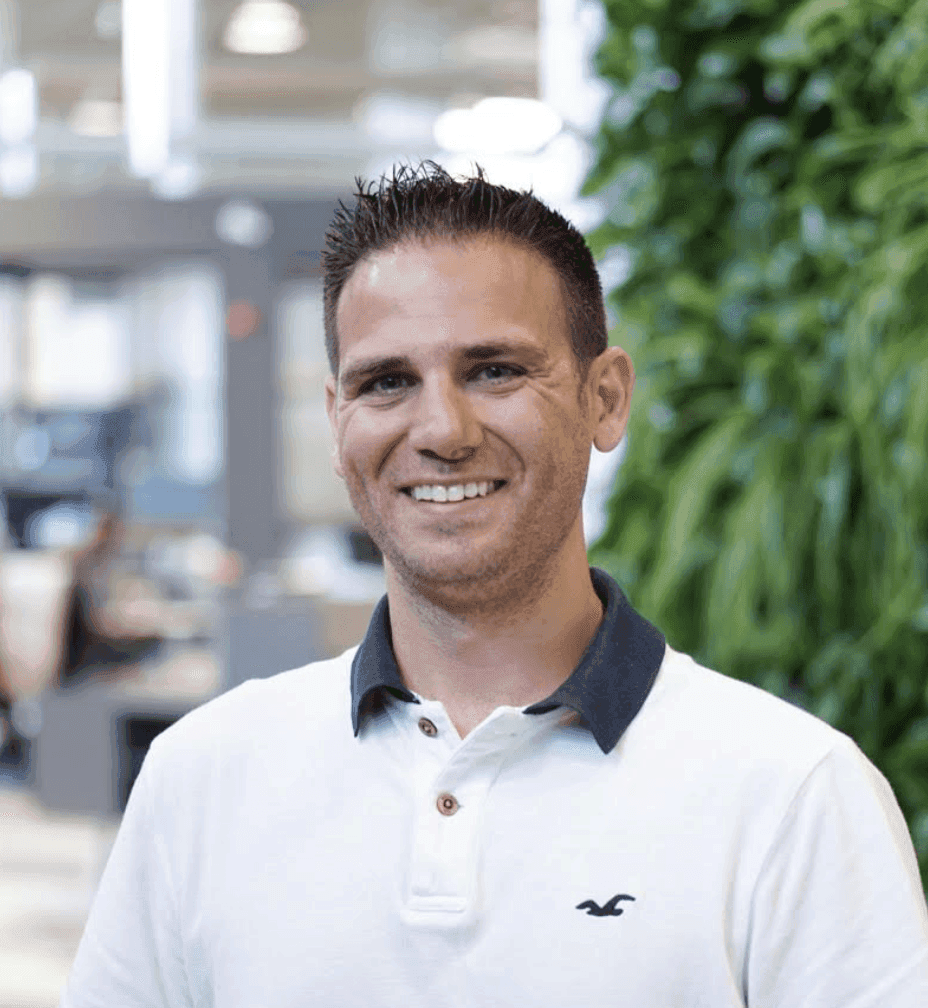
“Visibility on metrics in V7 is very helpful to us, and it's something we didn’t have in our internal solution.”
Andrew Achkar
Technical Director at Miovison
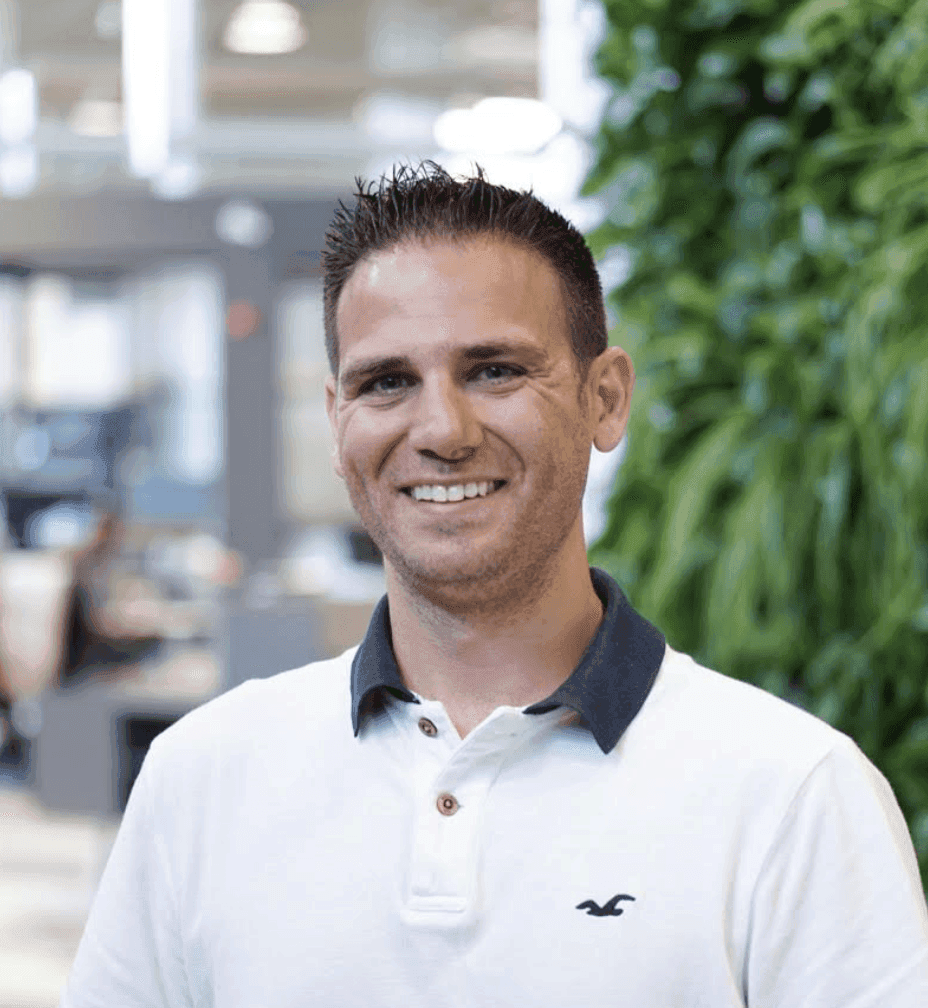
“Visibility on metrics in V7 is very helpful to us, and it's something we didn’t have in our internal solution.”
Andrew Achkar
Technical Director at Miovison
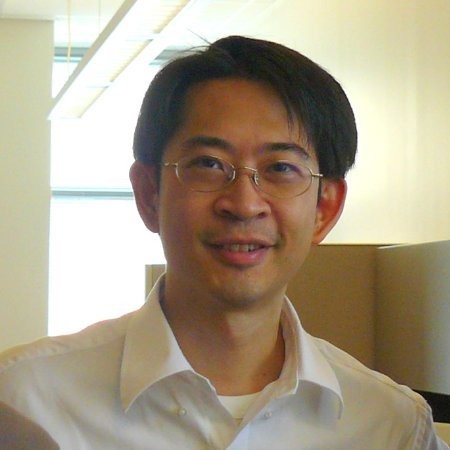
“V7 is great. The API is very straightforward to use, so we can easily get data into our system.”
David Soong
Director, Translational Data Science at Genmab
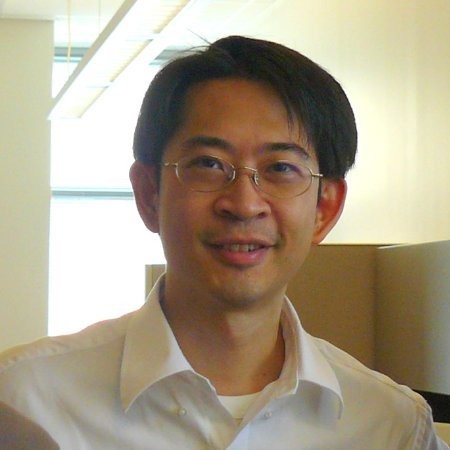
“V7 is great. The API is very straightforward to use, so we can easily get data into our system.”
David Soong
Director, Translational Data Science at Genmab
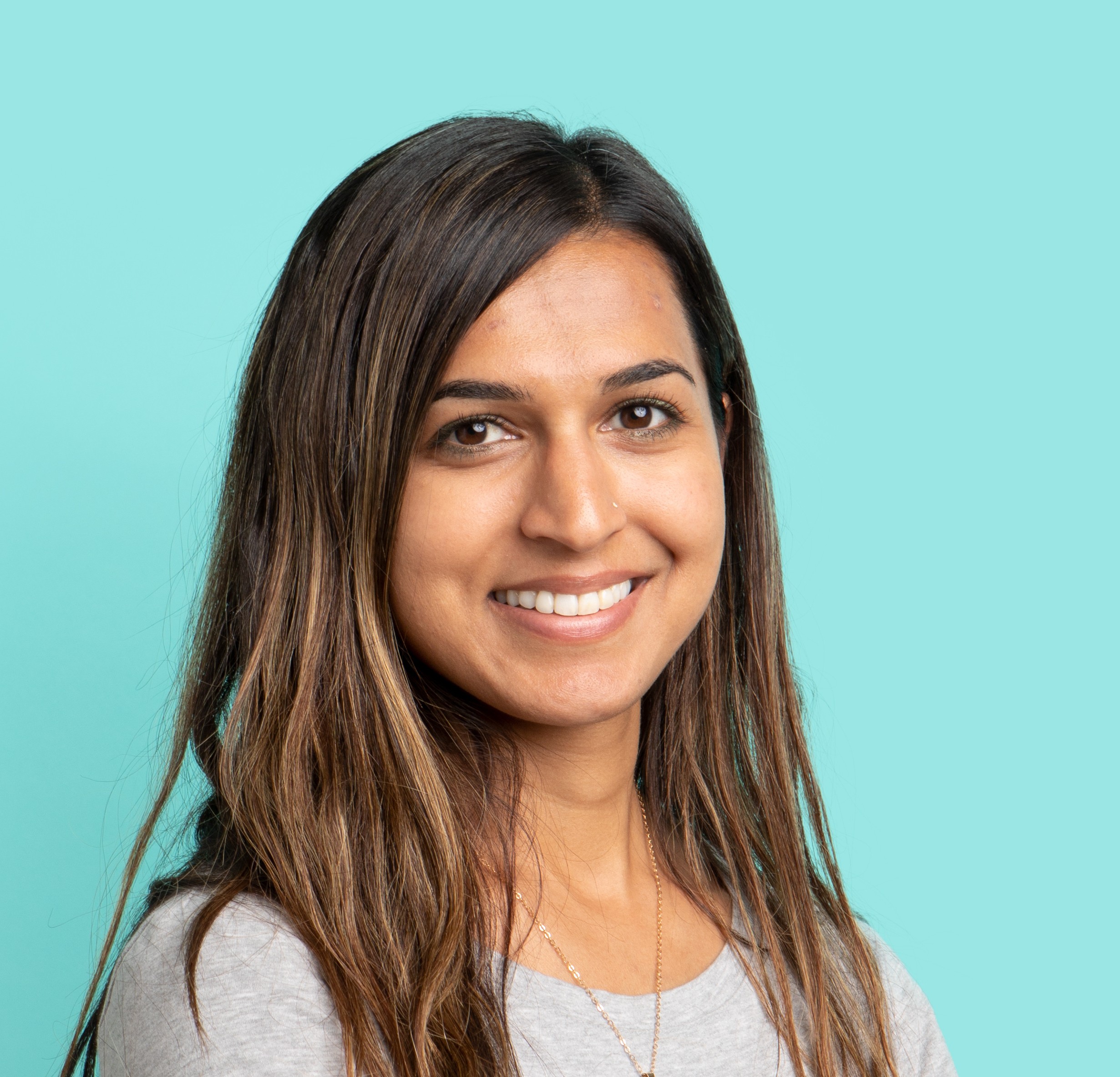
“We conducted extensive research of annotation tools and ultimately chose V7.”
Maleeha Nawaz
Manager of Quality and Data Curation at Imidex
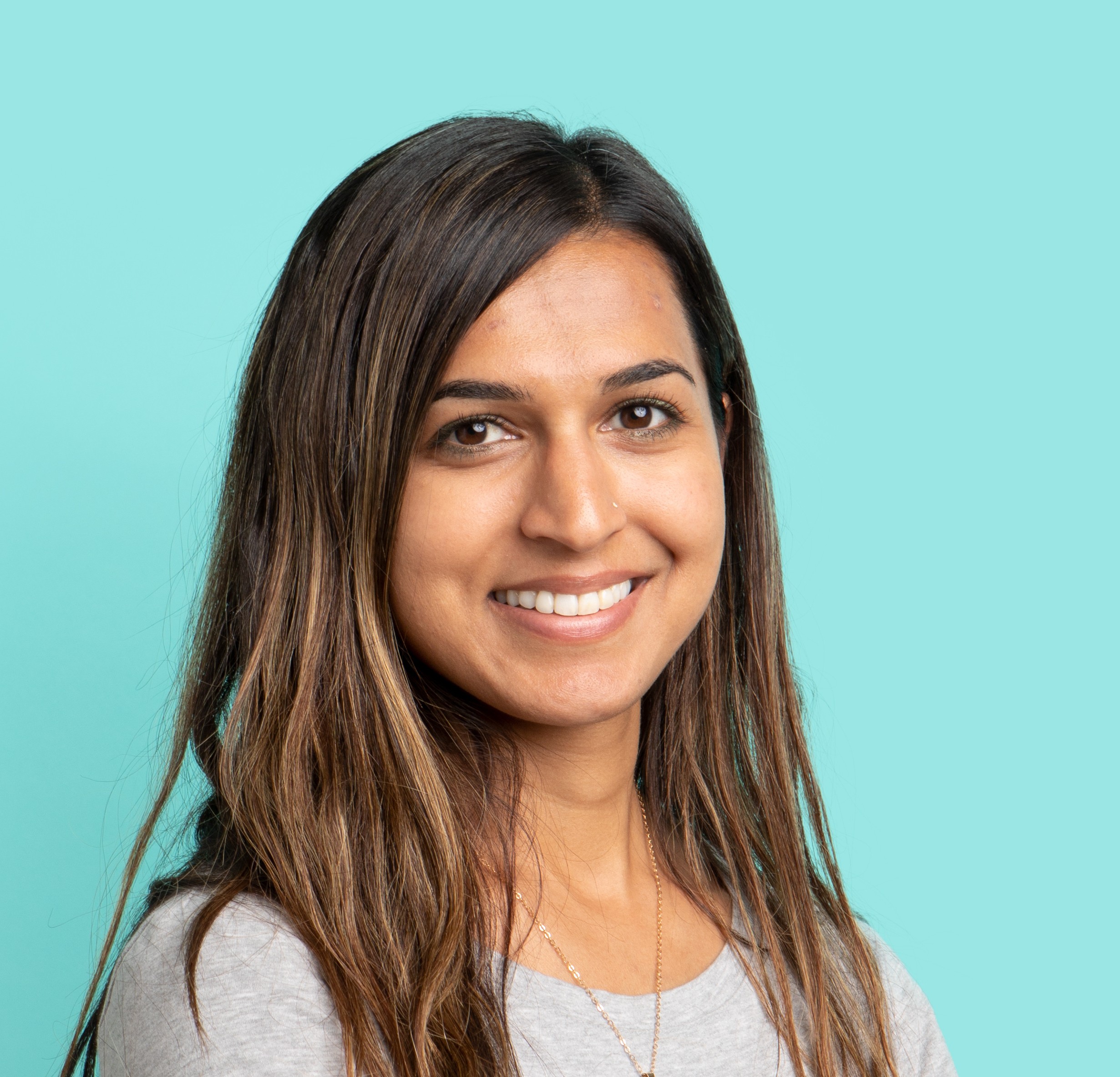
“We conducted extensive research of annotation tools and ultimately chose V7.”
Maleeha Nawaz
Manager of Quality and Data Curation at Imidex
File formats
File formats
File formats
Handle any medical imaging format with ease.
Handle any medical imaging format with ease.
DICOM and NIfTI support
Explore advanced visualization tools like oblique plane views, precise crosshairs, and 3D reconstructions. Adjust windowing, switch between MPR views, and interact with volumetric data in cinematic 3D for in-depth analysis.
Whole slide imaging (WSI)
Navigate and annotate high-resolution microscopy images at any zoom level with precision. Unlock insights from multi-layer samples with different stains or fluorescence channels. Improve your histopathology workflows with powerful digital pathology tools.
Surgical and ultrasound videos
Customize playback speed and synchronize multi-camera surgical setups. Accelerate video annotations with auto-tracking for objects that change position across frames. Automatically detect in-view and out-of-view objects, and track instances across long videos.
Multi-slot and multi-channel data
Create custom layouts to compare different imaging modalities or time points. Use hanging protocols and presets for analysis of complex medical studies. Import images with multiple overlays and easily navigate between layers or views using shortcuts.
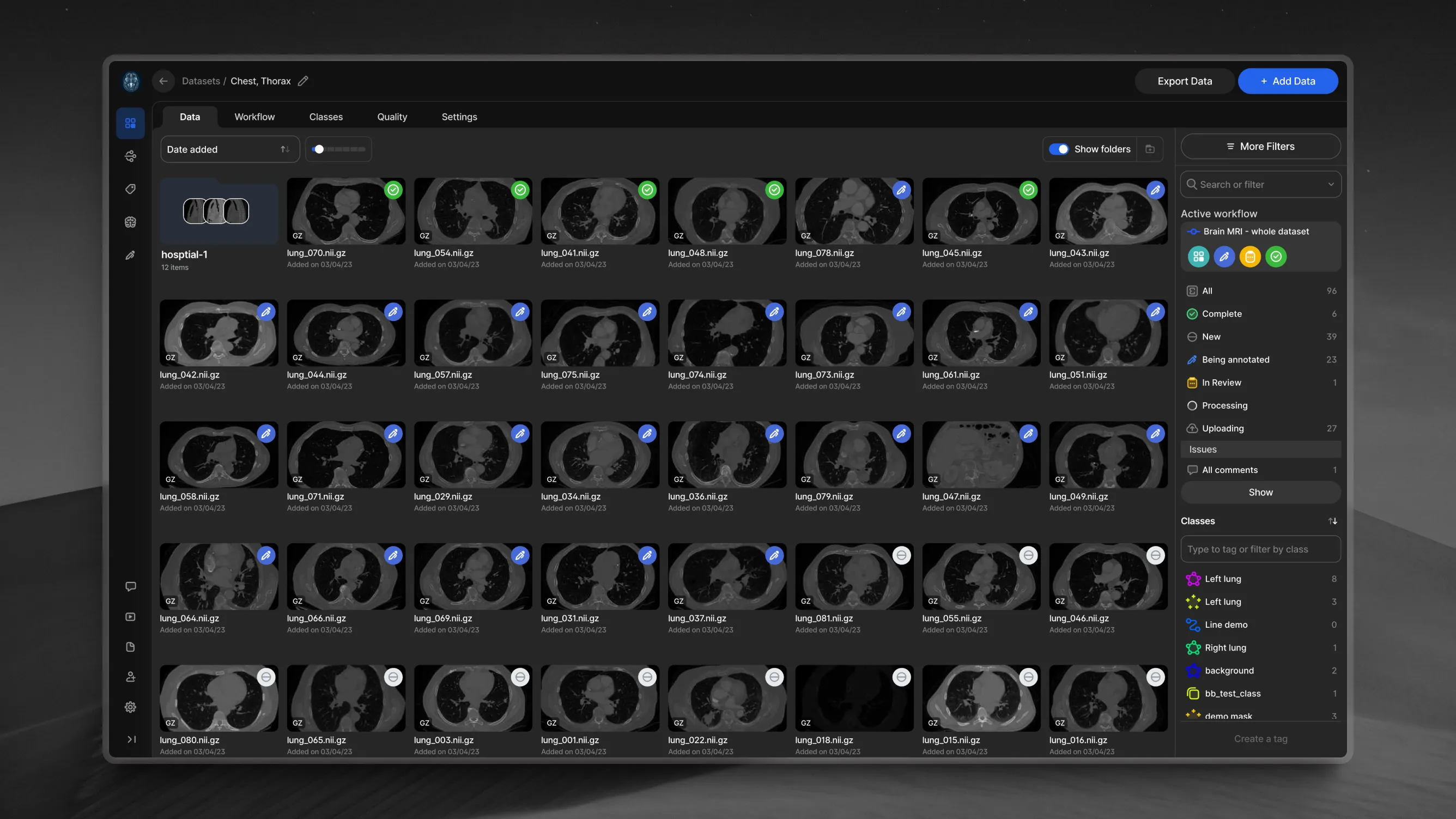
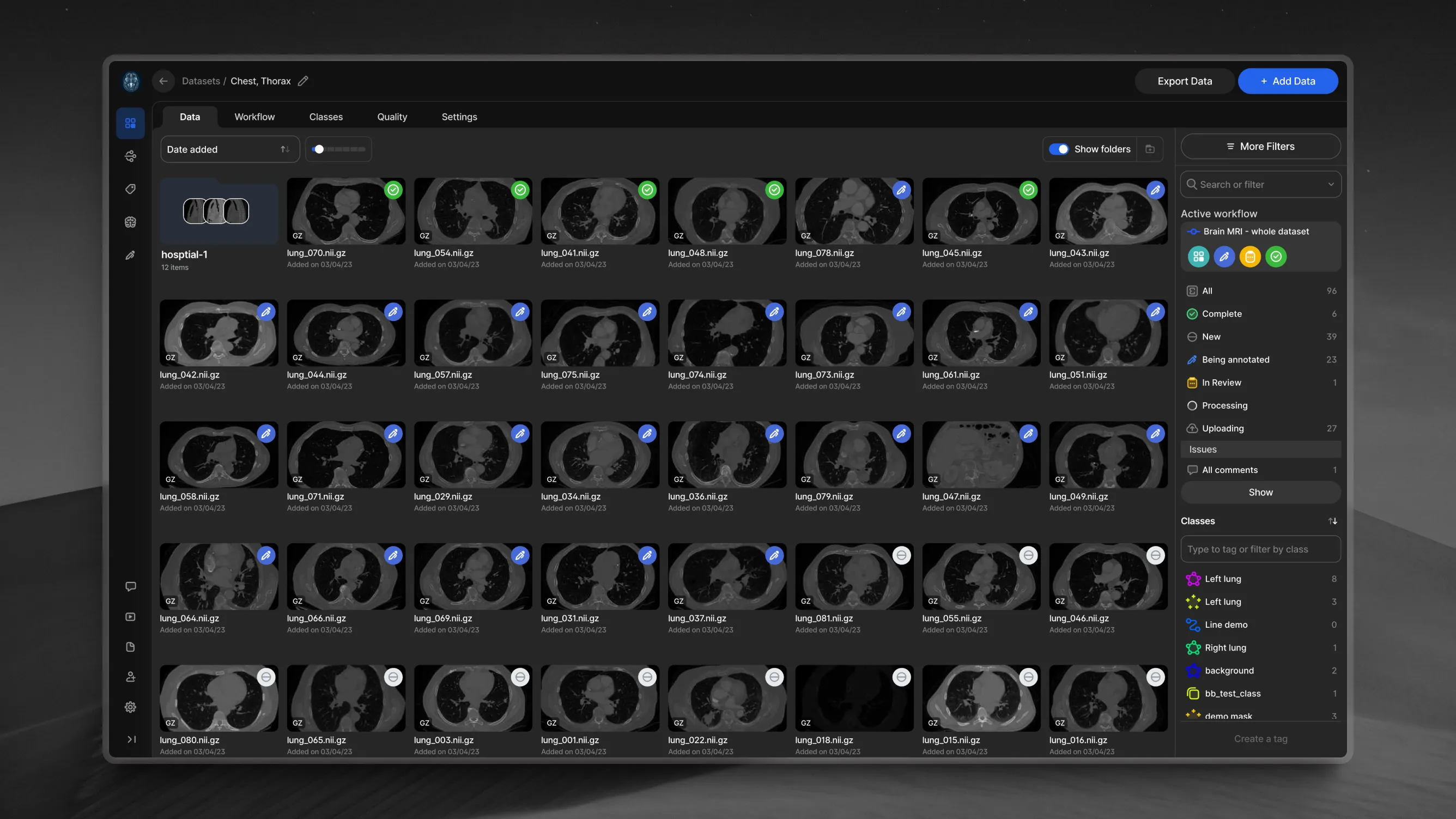
Collaborative ecosystem for medical experts
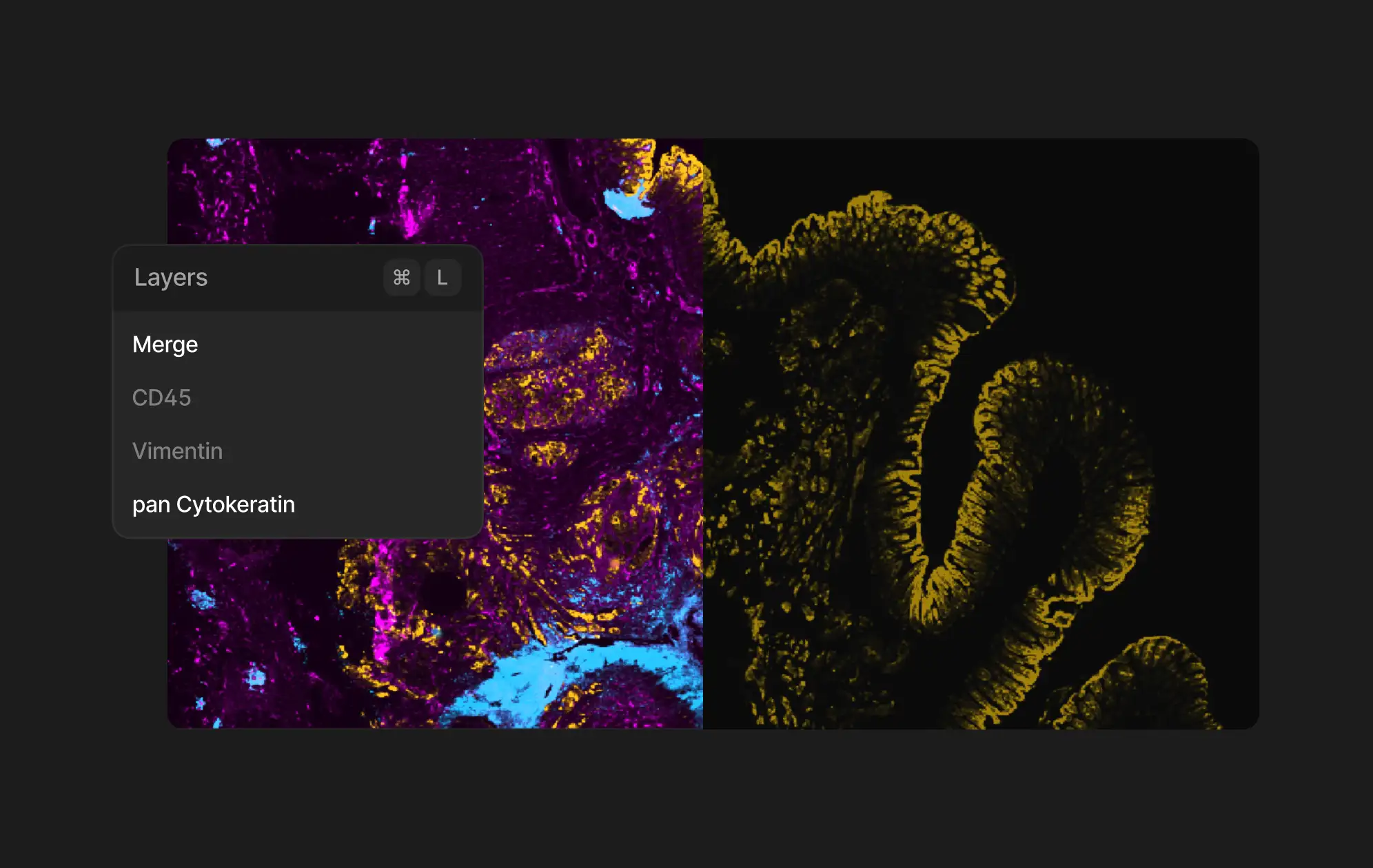
Break down geographical barriers in medical AI development. Explore collaboration features and allow multidisciplinary teams to annotate, review, and discuss medical imaging data. Assign tasks, flag issues for review, measure inter-reader variability, and maintain a clear audit trail of decisions—all within a single platform.
Collaborative ecosystem for medical experts
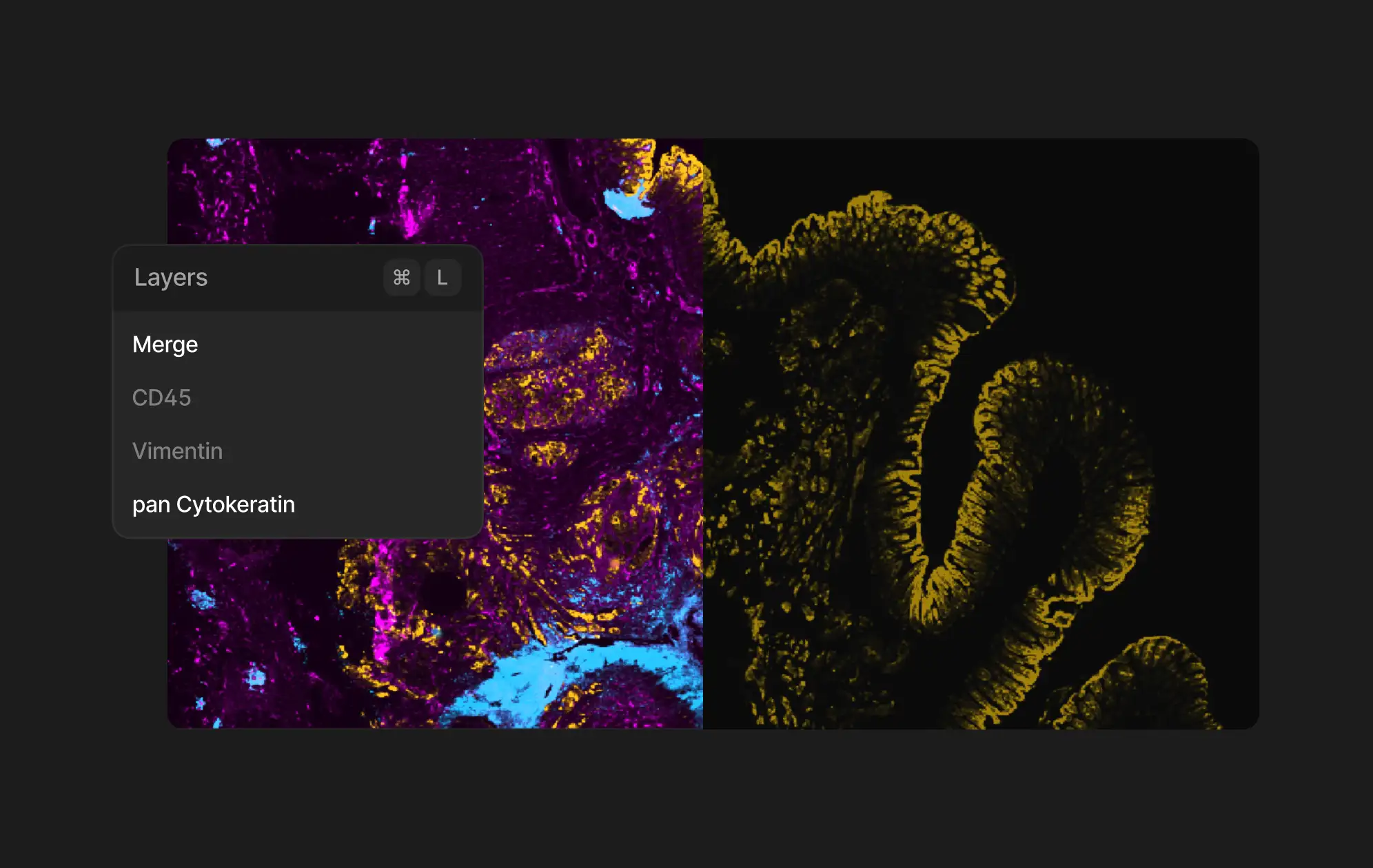
Break down geographical barriers in medical AI development. Explore collaboration features and allow multidisciplinary teams to annotate, review, and discuss medical imaging data. Assign tasks, flag issues for review, measure inter-reader variability, and maintain a clear audit trail of decisions—all within a single platform.
Just like your favorite DICOM viewer
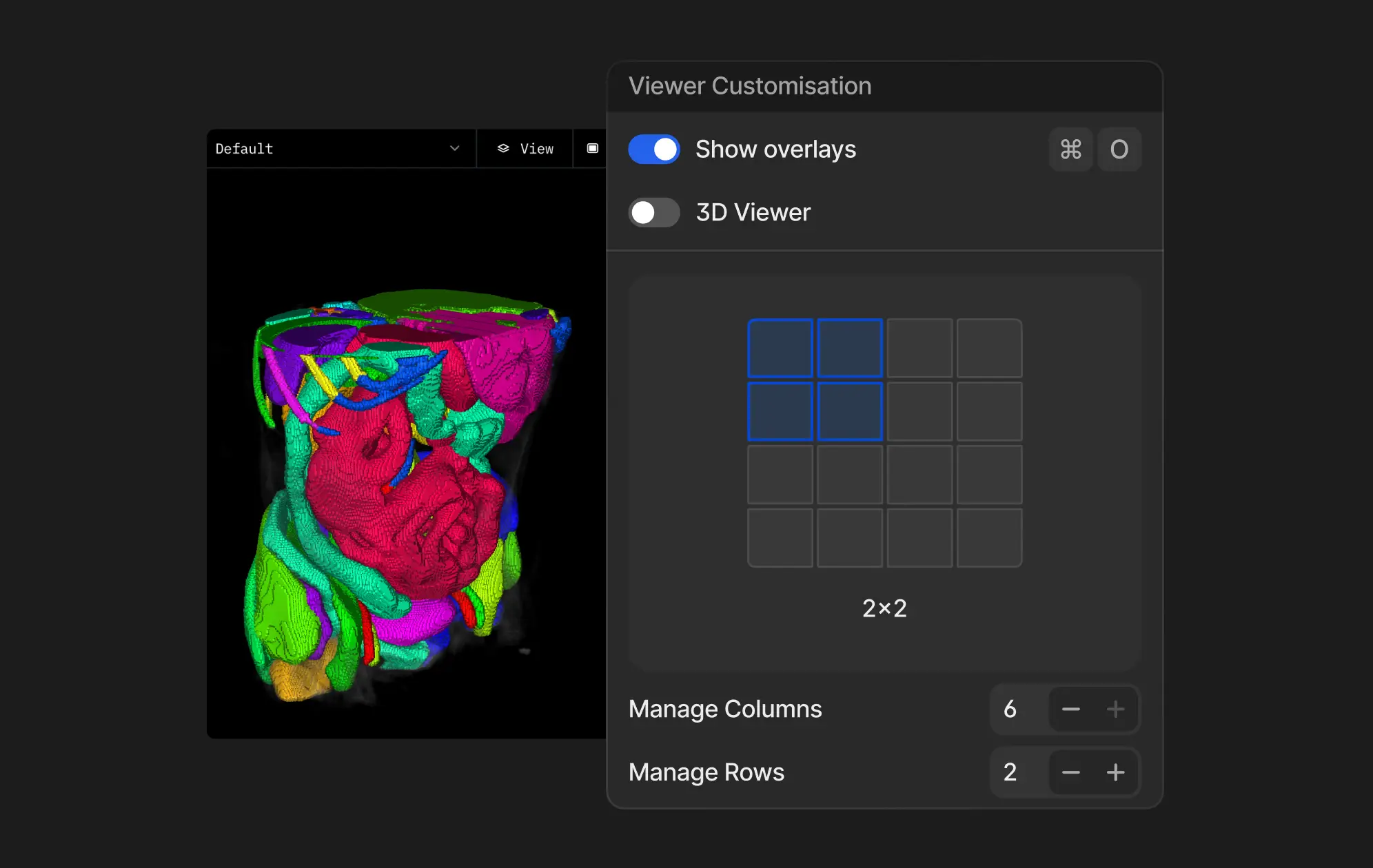
V7 Darwin is like a top-tier DICOM viewer, enhanced with state-of-the-art AI solutions such as MedSAM and Auto-track. Use MPR views, precise crosshair navigation, color maps, and windowing. Manage volumetric files and accelerate workflows with AI-assisted annotations and quality control features.
Just like your favorite DICOM viewer
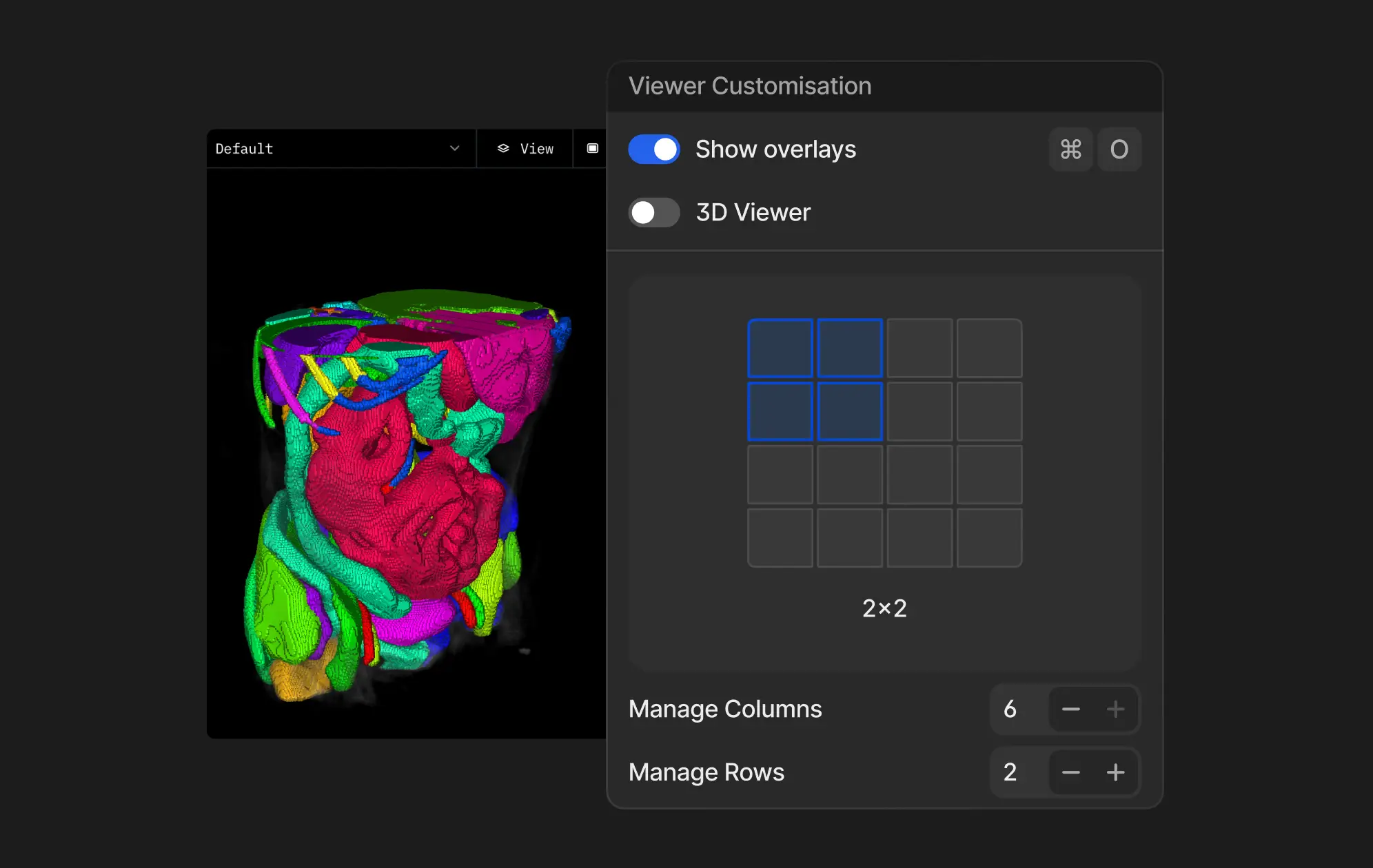
V7 Darwin is like a top-tier DICOM viewer, enhanced with state-of-the-art AI solutions such as MedSAM and Auto-track. Use MPR views, precise crosshair navigation, color maps, and windowing. Manage volumetric files and accelerate workflows with AI-assisted annotations and quality control features.
Automated labeling
Automated labeling
Automated labeling
Feel the speed.
Pixel perfect annotations.
Feel the speed.
Pixel perfect annotations.
Auto-track volumetric series
Thresholding 3D brush
Segmentation with MedSAM
TotalSegmentator
Bring your own model
Annotate regions of interest in a selected slice and generate automatic annotation for the whole series to speed up the labeling.
Auto-track volumetric series
Thresholding 3D brush
Segmentation with MedSAM
TotalSegmentator
Bring your own model
Annotate regions of interest in a selected slice and generate automatic annotation for the whole series to speed up the labeling.
Features
Features
Features
DICOM viewer experience.
Powerful visuals, full control.
DICOM viewer experience.
Powerful visuals, full control.
V7 DICOM annotation viewer combines familiar tools with advanced AI features to enhance your medical imaging workflow. Explore multiplanar reconstructions, cinematic 3D, and auto-segmentation tools that bring your data to life.
We've improved the standard viewing experience with practical collaboration tools. Add comments directly on images, volumes, or videos. Share feedback in real-time, and use the Consensus feature to measure agreement between annotators and resolve issues.


Multi-planar views
Visualize complex anatomy from multiple angles, including oblique plane support
Multi-planar views
Visualize complex anatomy from multiple angles, including oblique plane support
Flexible hanging protocols
Customize and replicate preferred layouts for consistent viewing
Flexible hanging protocols
Customize and replicate preferred layouts for consistent viewing
Multiple channel support
Analyze different layers of your pathology samples
Multiple channel support
Analyze different layers of your pathology samples
Cinematic 3D rendering
Use presets, zoom in, and rotate to see structures with clarity
Cinematic 3D rendering
Use presets, zoom in, and rotate to see structures with clarity
3D voxel support
Create volumetric pixel masks with brushes or AI tools
3D voxel support
Create volumetric pixel masks with brushes or AI tools
Intuitive timeline navigation
User-friendly interface for navigating between slices or video frames
Intuitive timeline navigation
User-friendly interface for navigating between slices or video frames
Stories
Hear what customers say.
Advancing healthcare with AI.
Hear what customers say. Advancing healthcare with AI.
With the help of V7-trained models, we can save up to 4.5 days of work per patient—and optimize the screenings even more in the future.
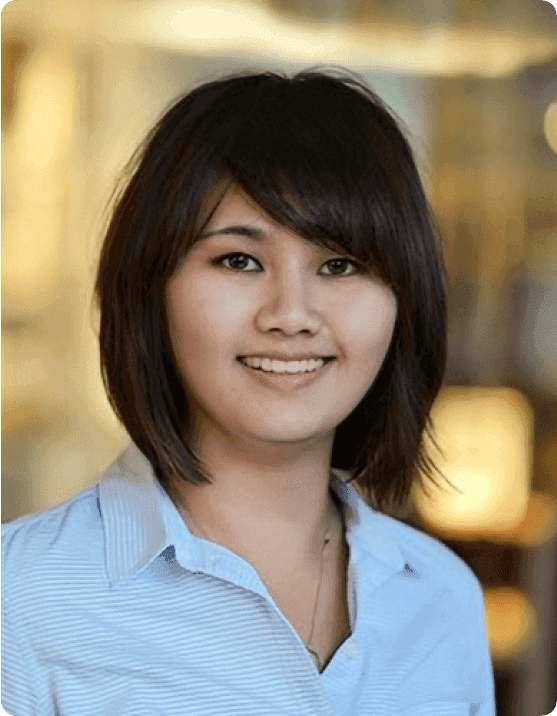
VP Compliance & Lab Automation at Vivan Therapeutics
Read story
→
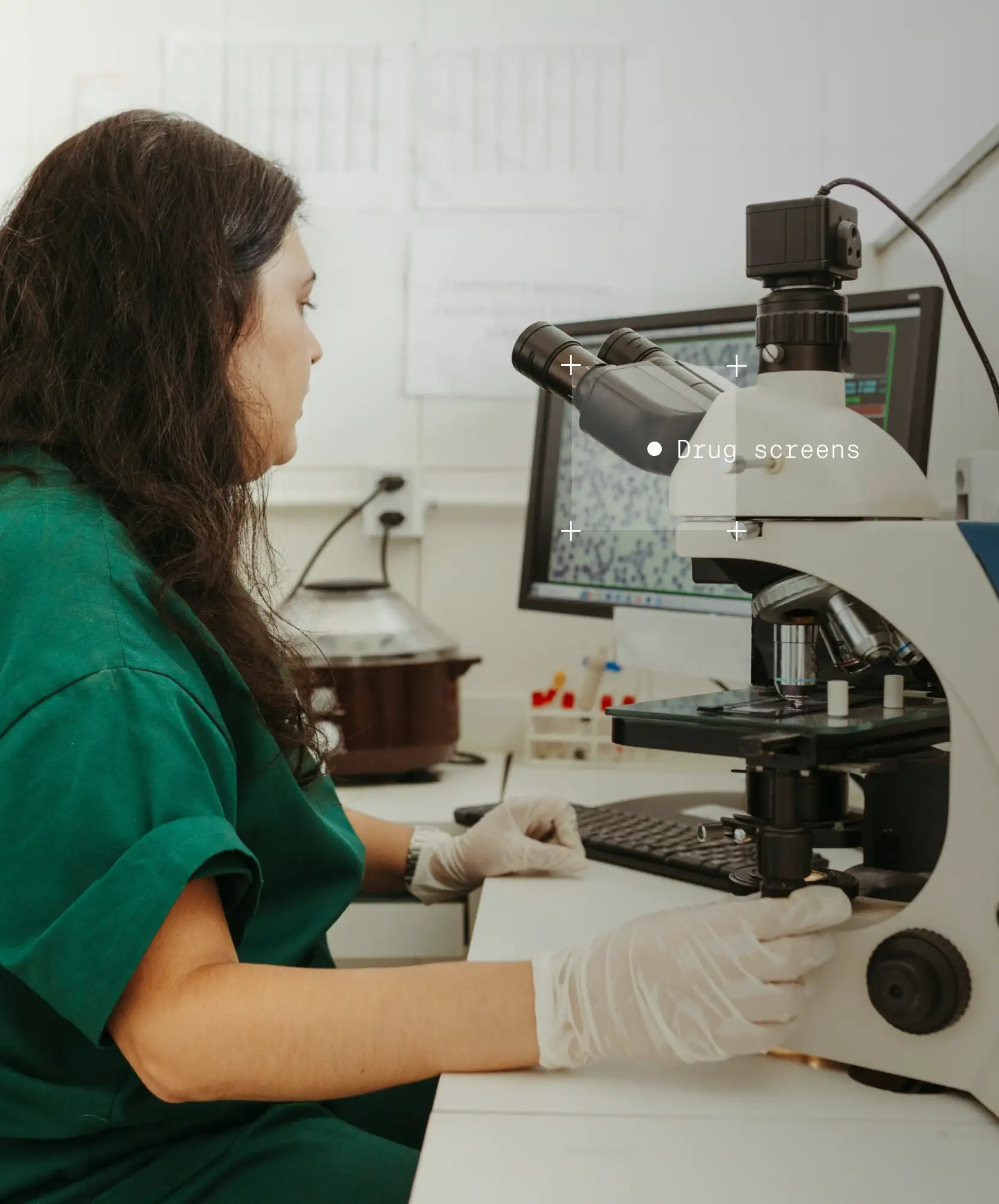
I have worked with 600+ vendors in my life. I always look at good technology, stable company, good people, and how much a vendor is invested in your success. I found all of those things with V7.
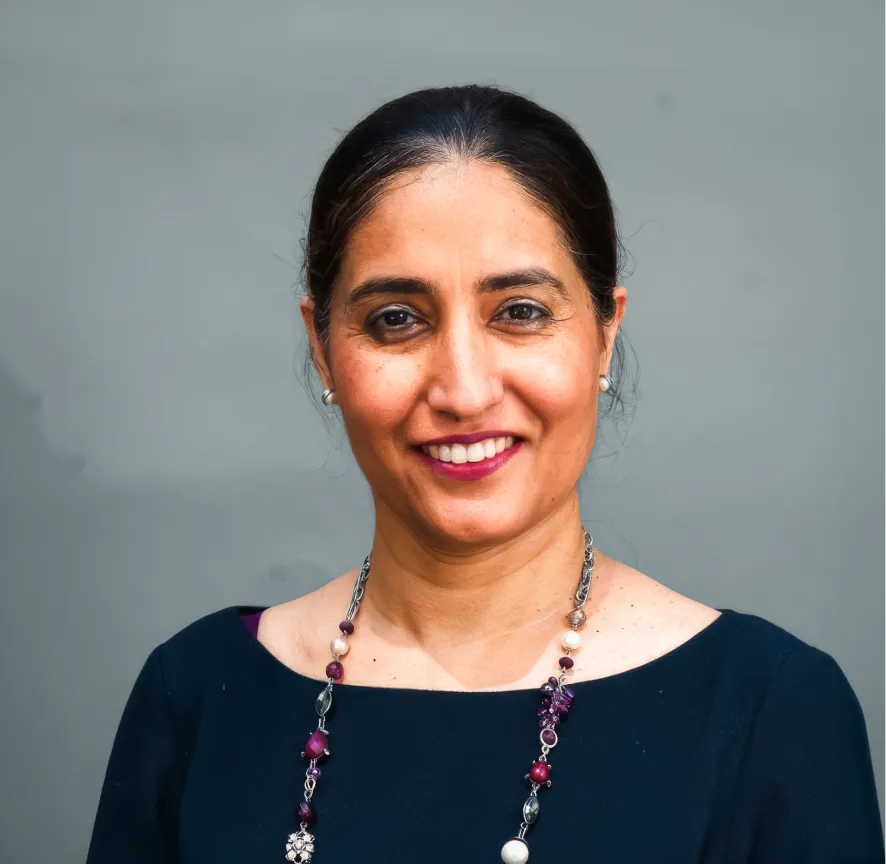
Co-founder & CEO of VELMENI
Read story
→
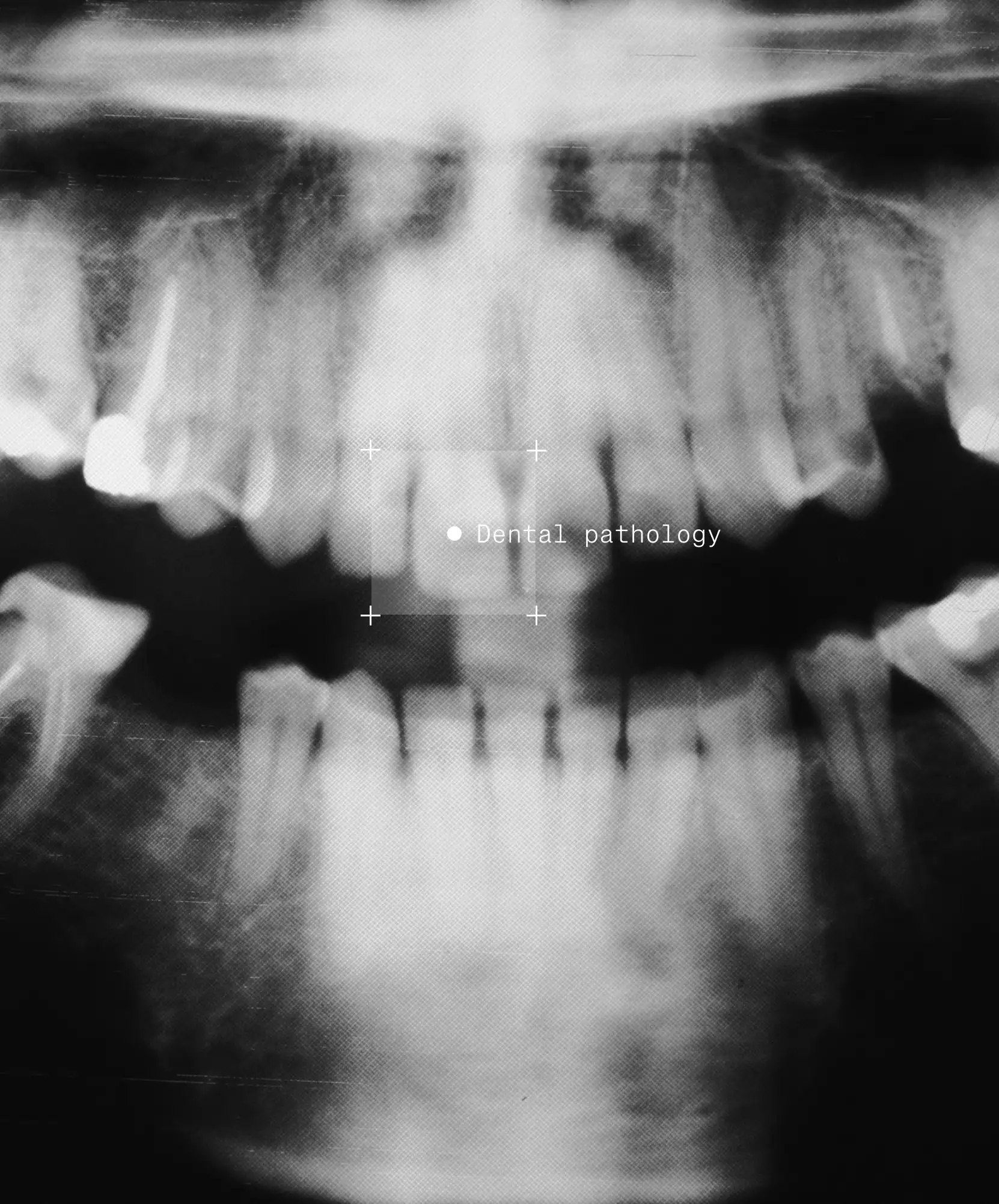
We use V7 to make our workflow for deep learning training and annotation streamlined and efficient. From the pathologist’s point of view, V7 turned out to be much easier to learn and use than other software - I can easily understand what I’m doing.
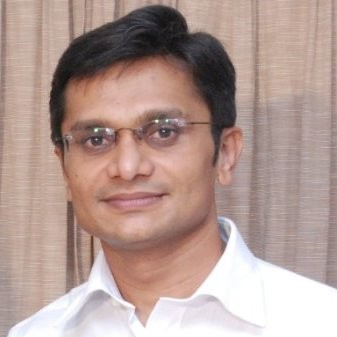
Director, Translational Research at Genmab
Read story
→
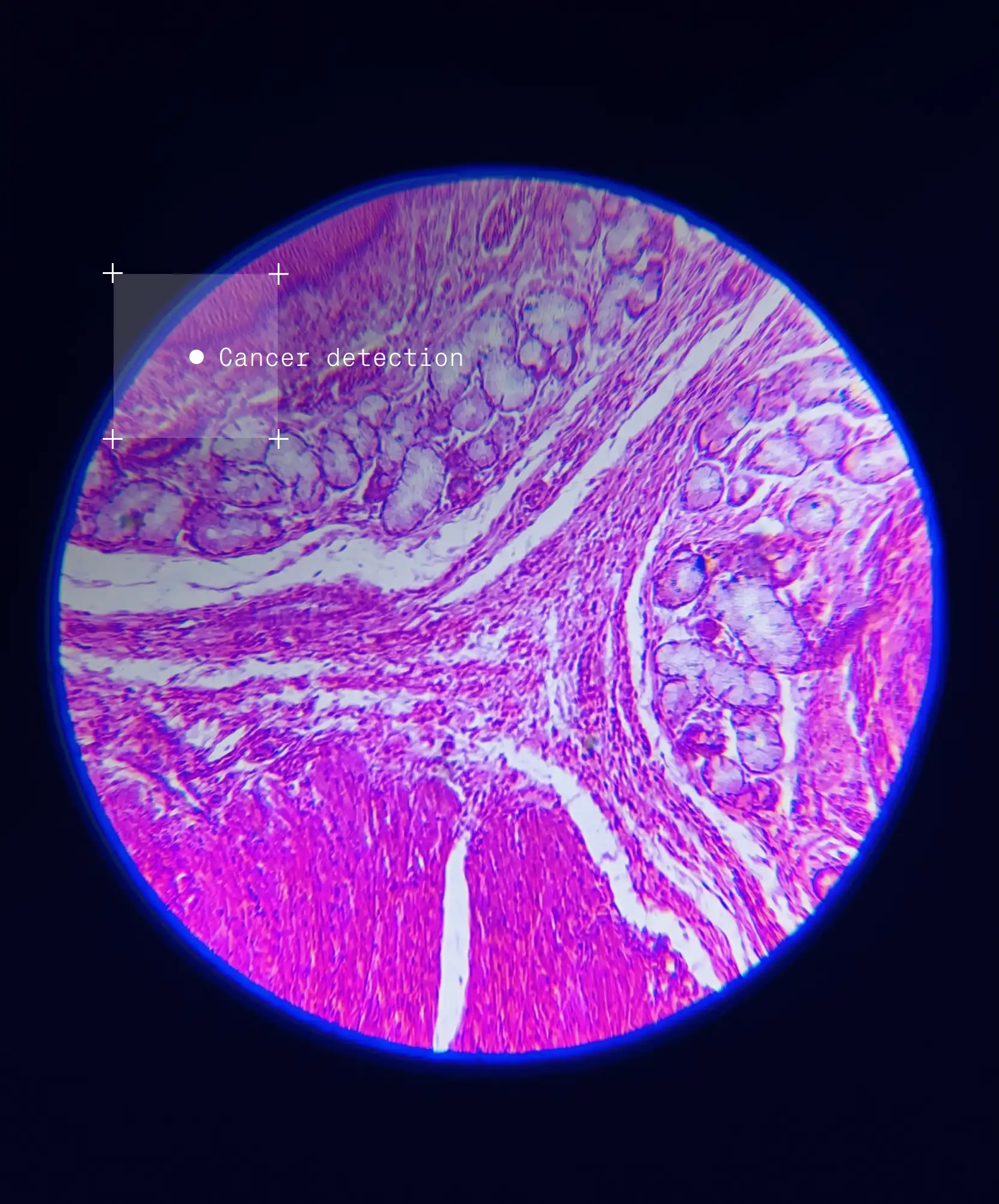
With the help of V7-trained models, we can save up to 4.5 days of work per patient—and optimize the screenings even more in the future.
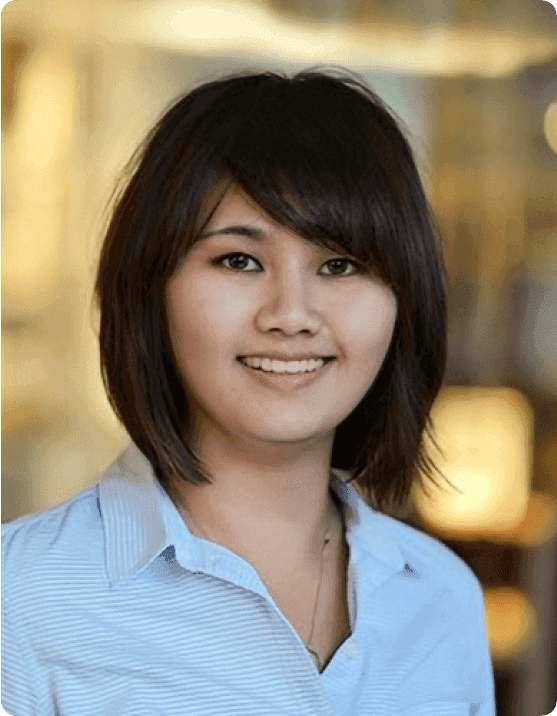
VP Compliance & Lab Automation at Vivan Therapeutics
Read story
→
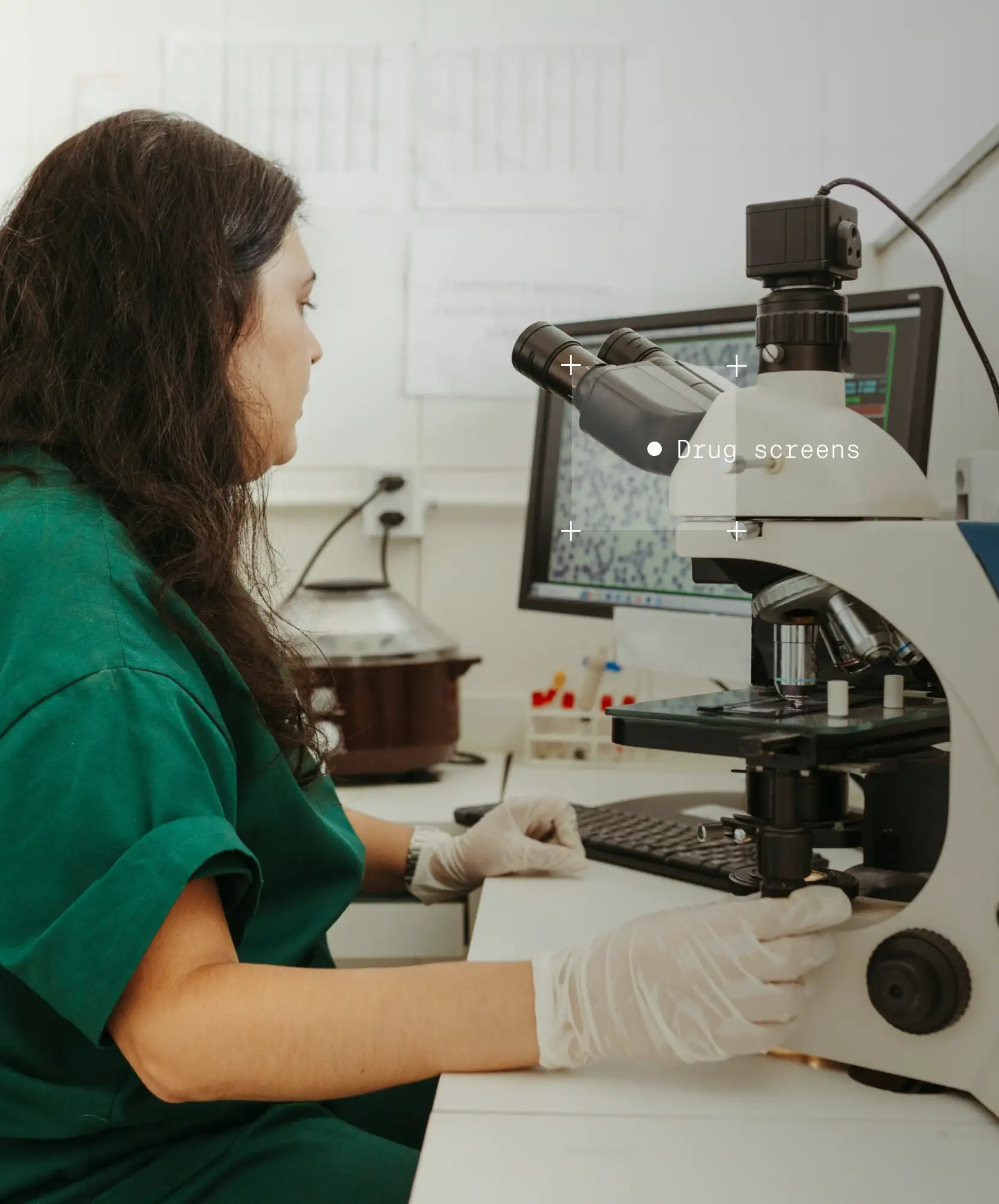
I have worked with 600+ vendors in my life. I always look at good technology, stable company, good people, and how much a vendor is invested in your success. I found all of those things with V7.
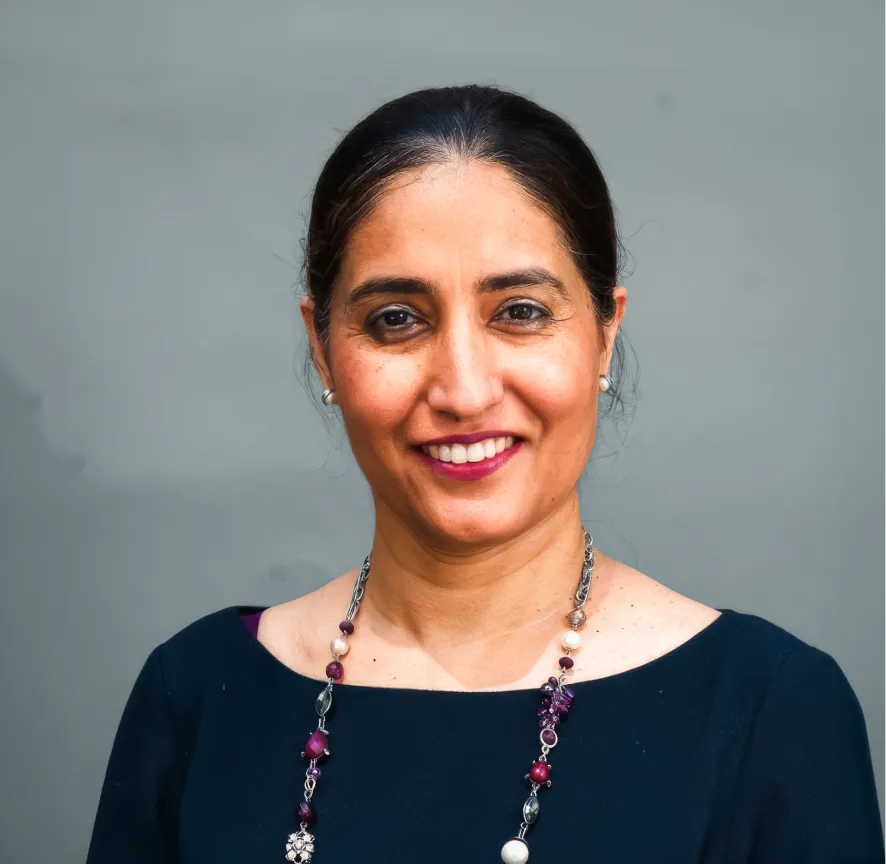
Co-founder & CEO of VELMENI
Read story
→
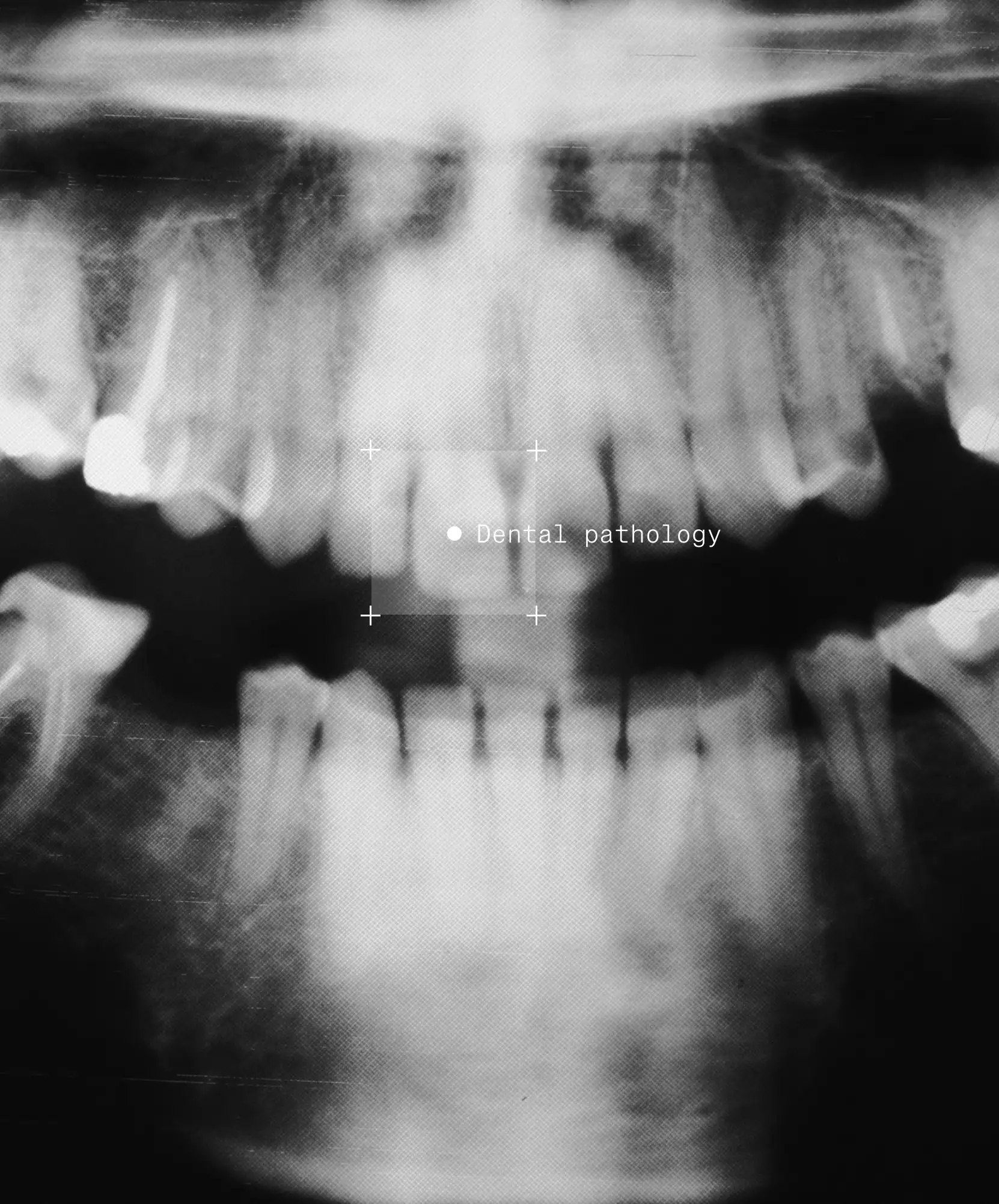
We use V7 to make our workflow for deep learning training and annotation streamlined and efficient. From the pathologist’s point of view, V7 turned out to be much easier to learn and use than other software - I can easily understand what I’m doing.
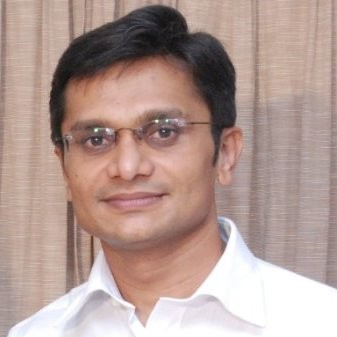
Director, Translational Research at Genmab
Read story
→
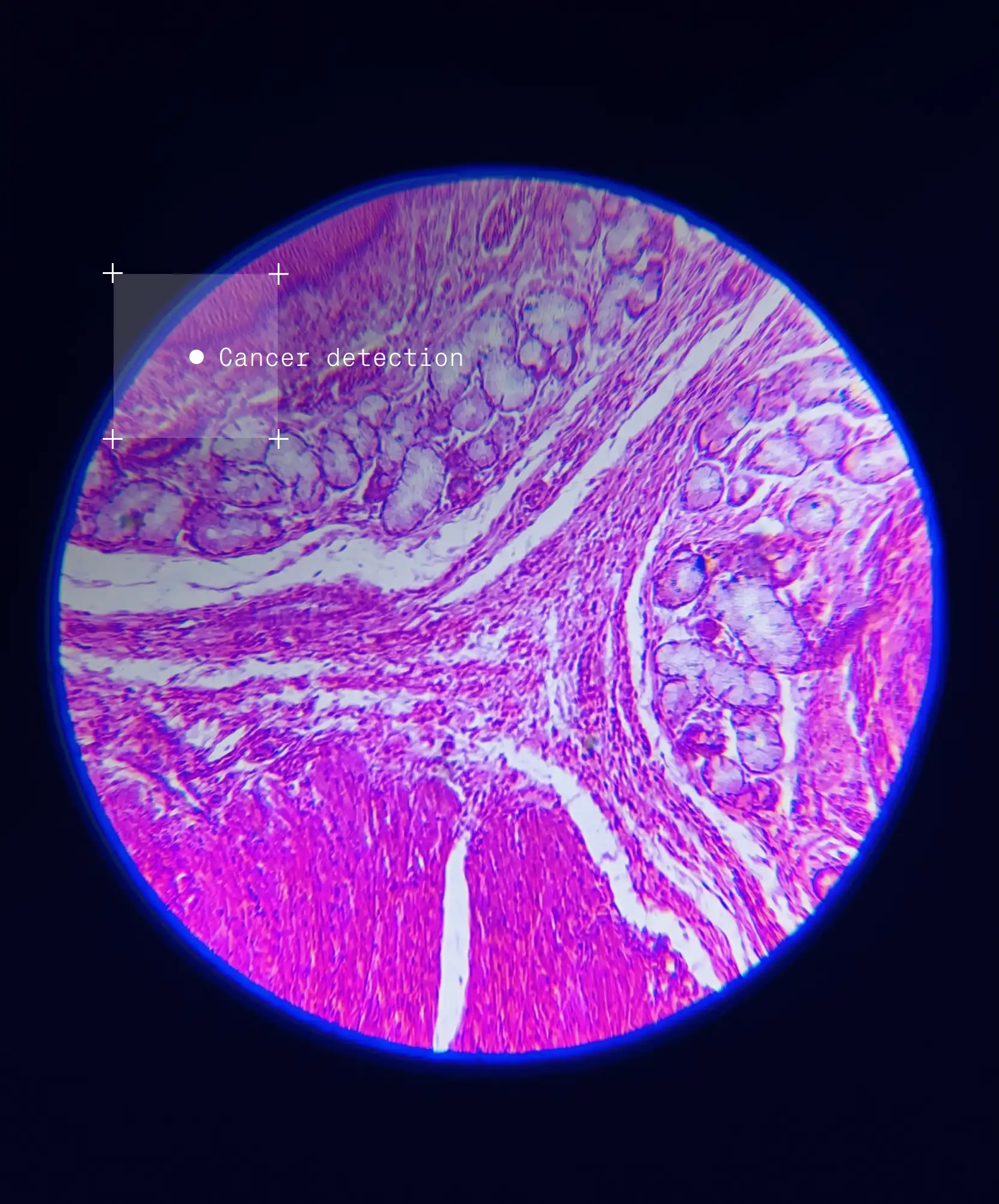
With the help of V7-trained models, we can save up to 4.5 days of work per patient—and optimize the screenings even more in the future.
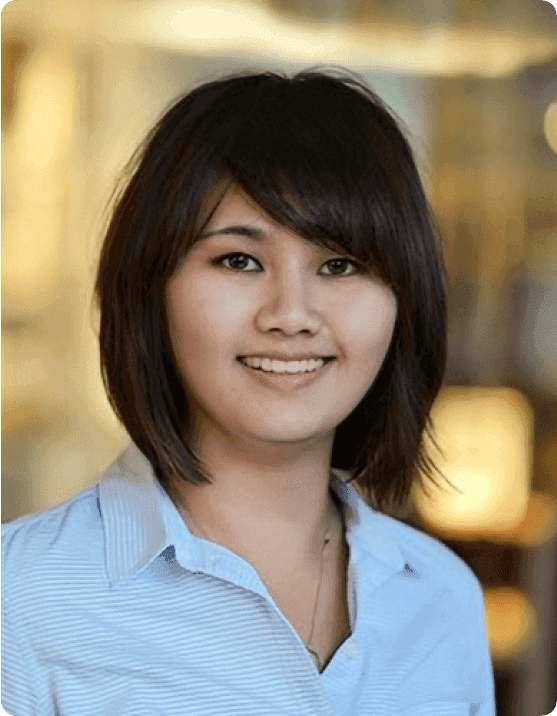
VP Compliance & Lab Automation at Vivan Therapeutics
Read story
→
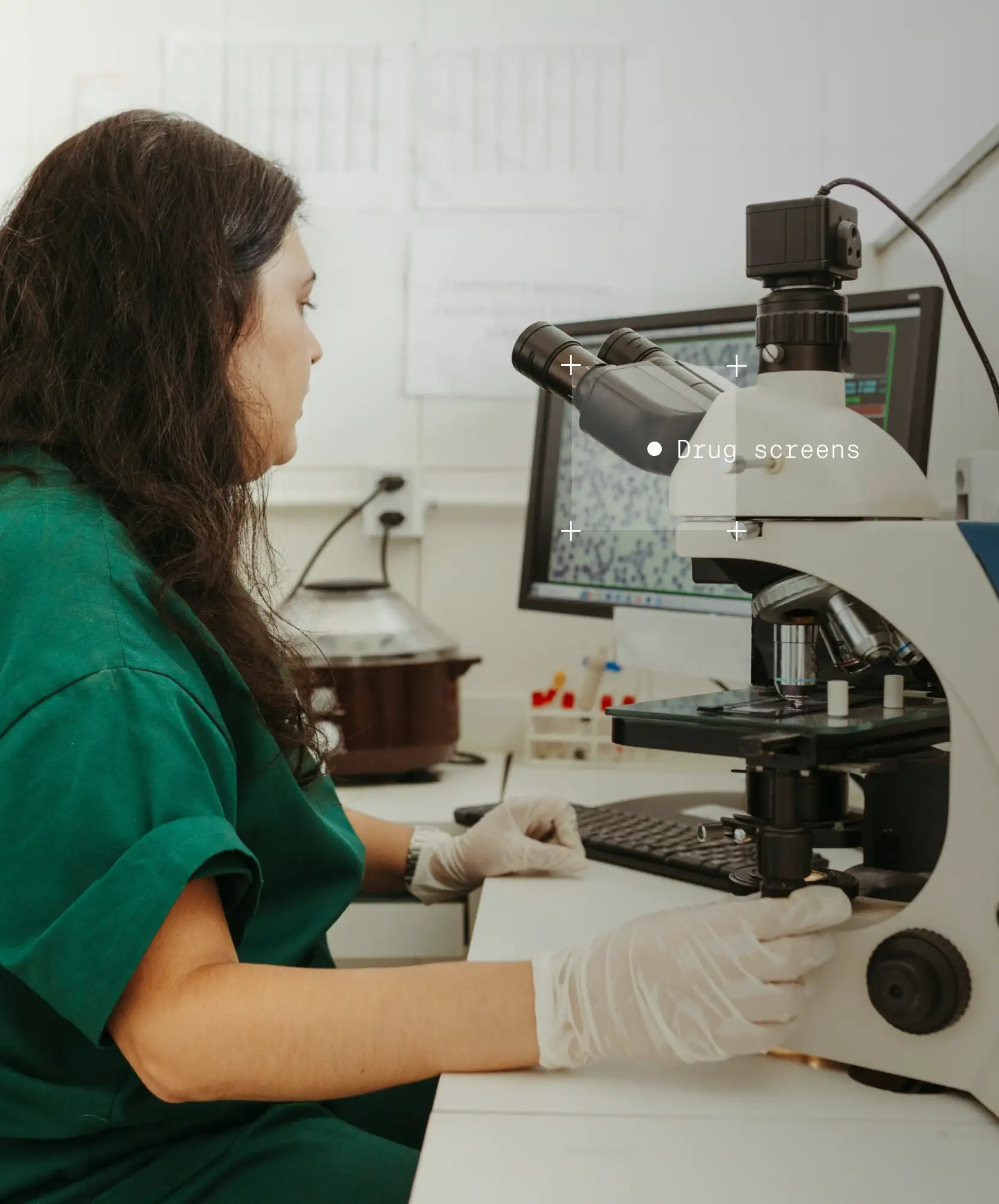
I have worked with 600+ vendors in my life. I always look at good technology, stable company, good people, and how much a vendor is invested in your success. I found all of those things with V7.
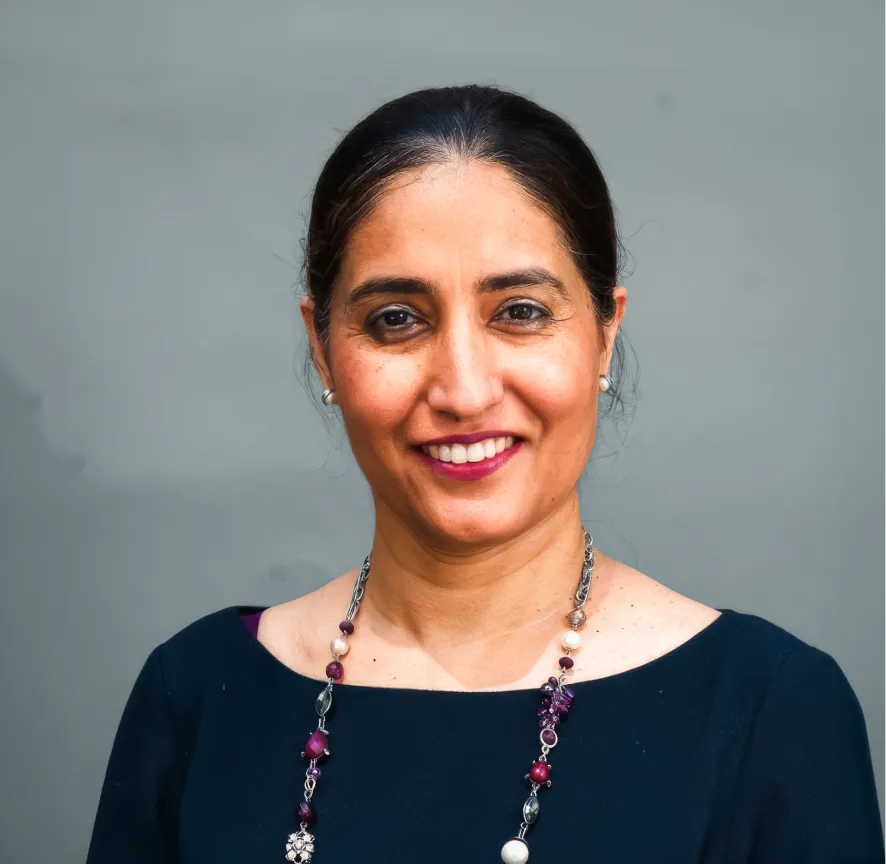
Co-founder & CEO of VELMENI
Read story
→
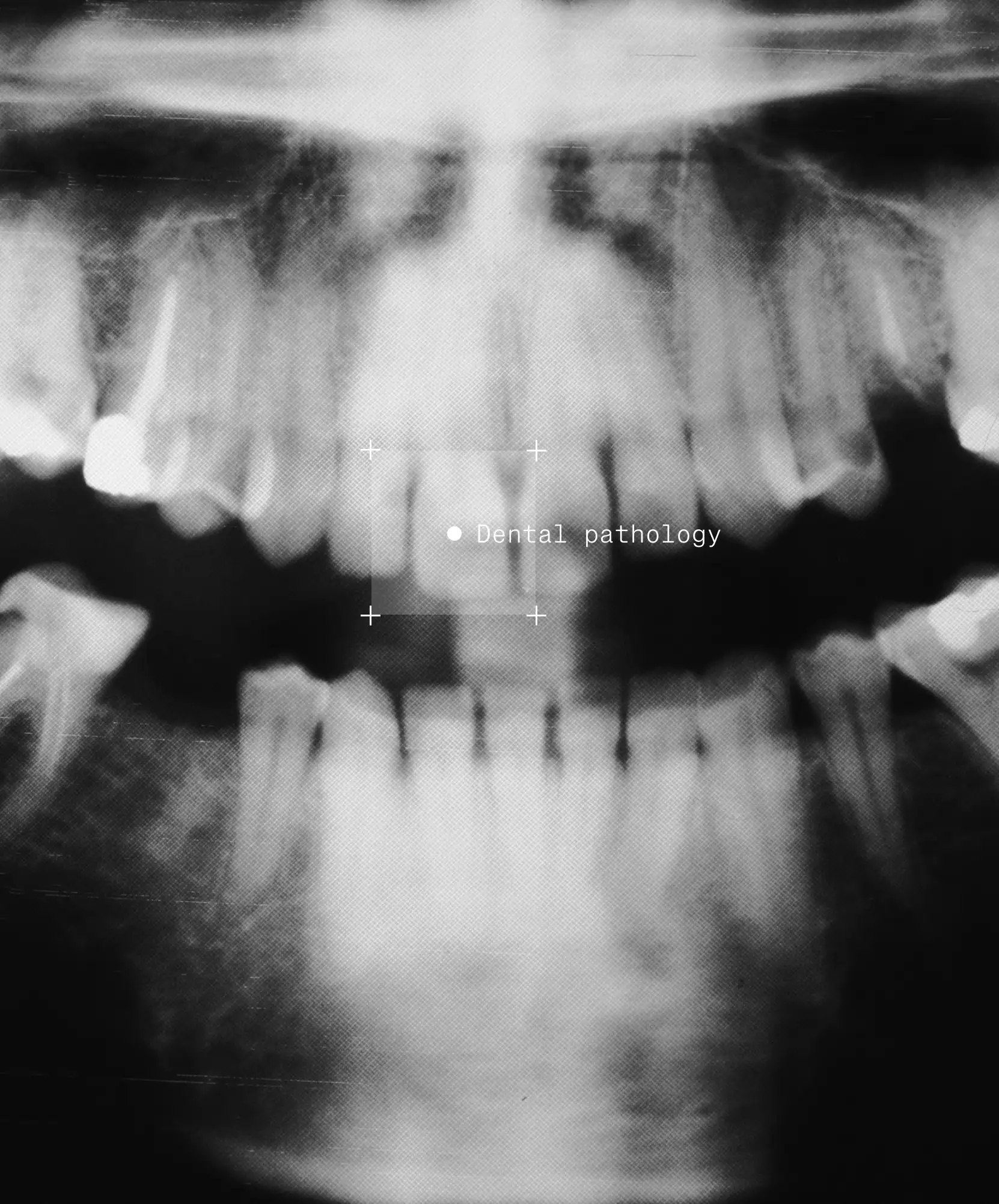
We use V7 to make our workflow for deep learning training and annotation streamlined and efficient. From the pathologist’s point of view, V7 turned out to be much easier to learn and use than other software - I can easily understand what I’m doing.
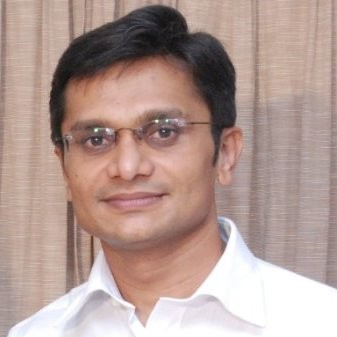
Director, Translational Research at Genmab
Read story
→
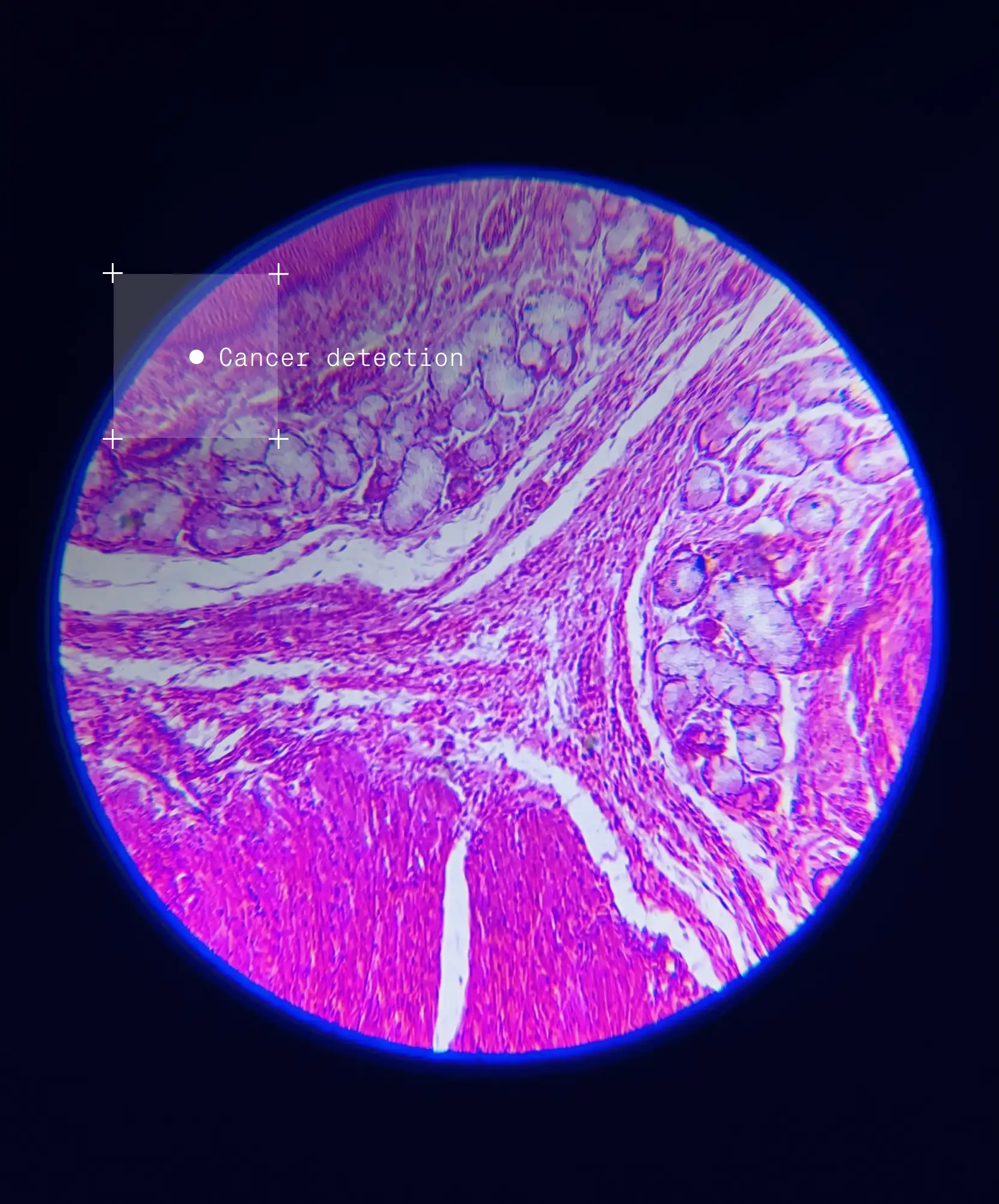
Labeling services
Labeling services
Labeling services
Looking for expert labeling services for medical AI?
Looking for expert labeling services for medical AI?
If you don’t have your own data labeling workforce or engineering team, we provide comprehensive labeling and AI development solutions. V7 can support your project from initial ideation through proof of concept, all the way to final deployment and evaluation.
If you don’t have your own data labeling workforce or engineering team, we provide comprehensive labeling and AI development solutions. V7 can support your project from initial ideation through proof of concept, all the way to final deployment and evaluation.
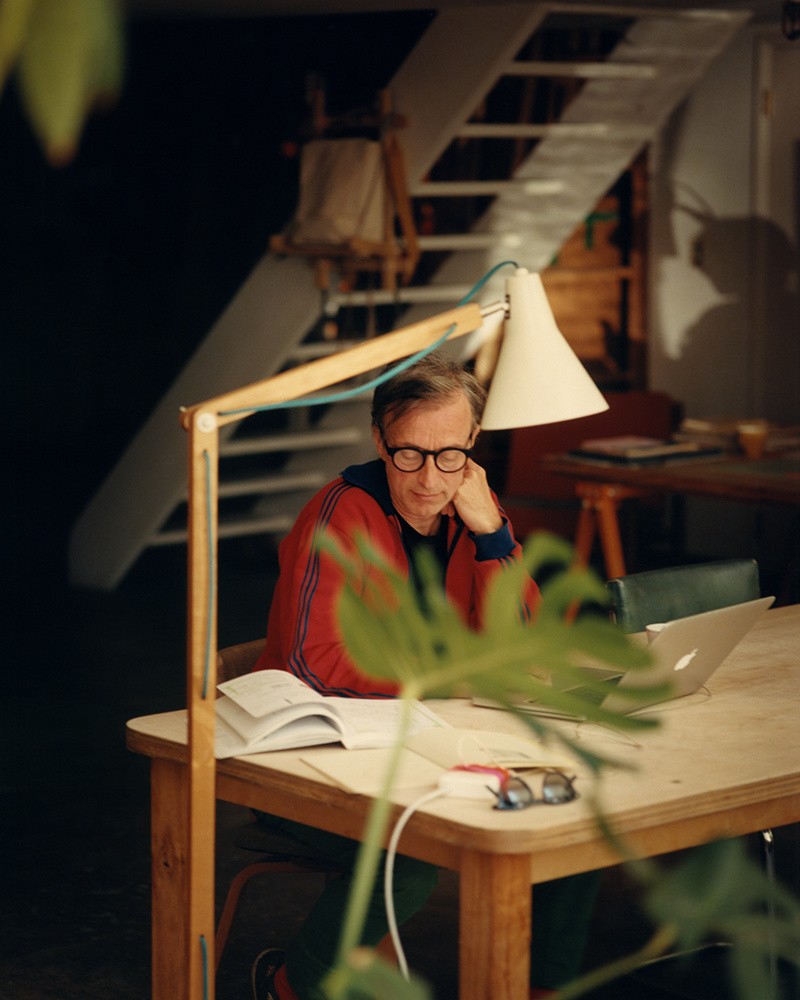
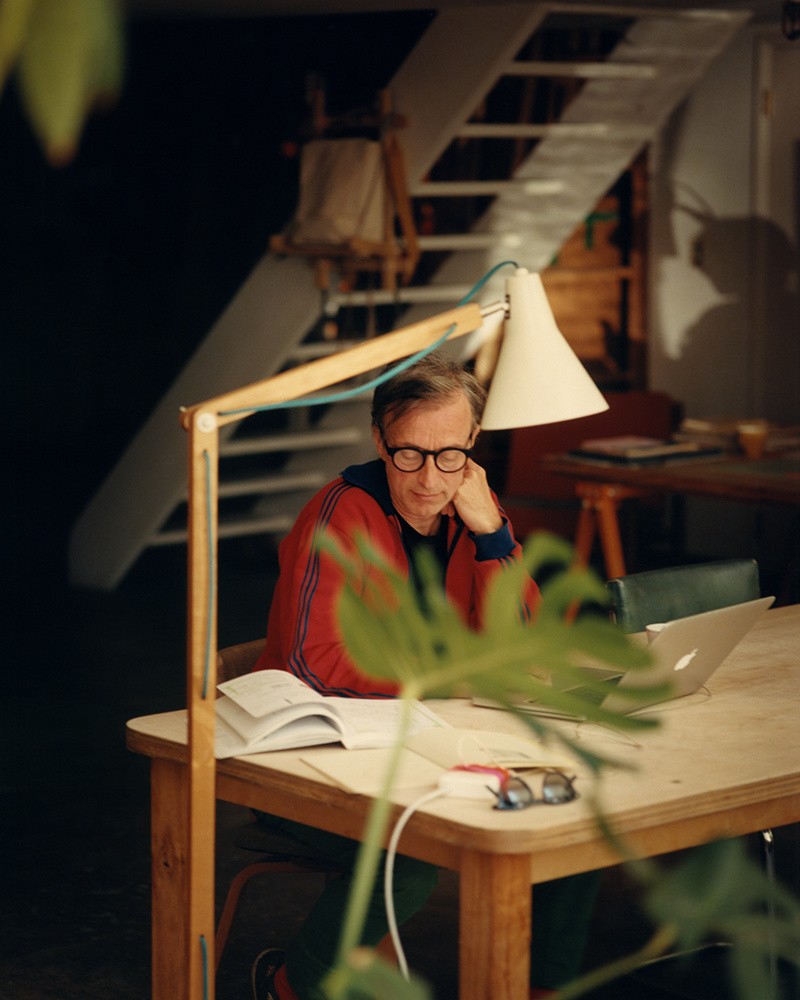
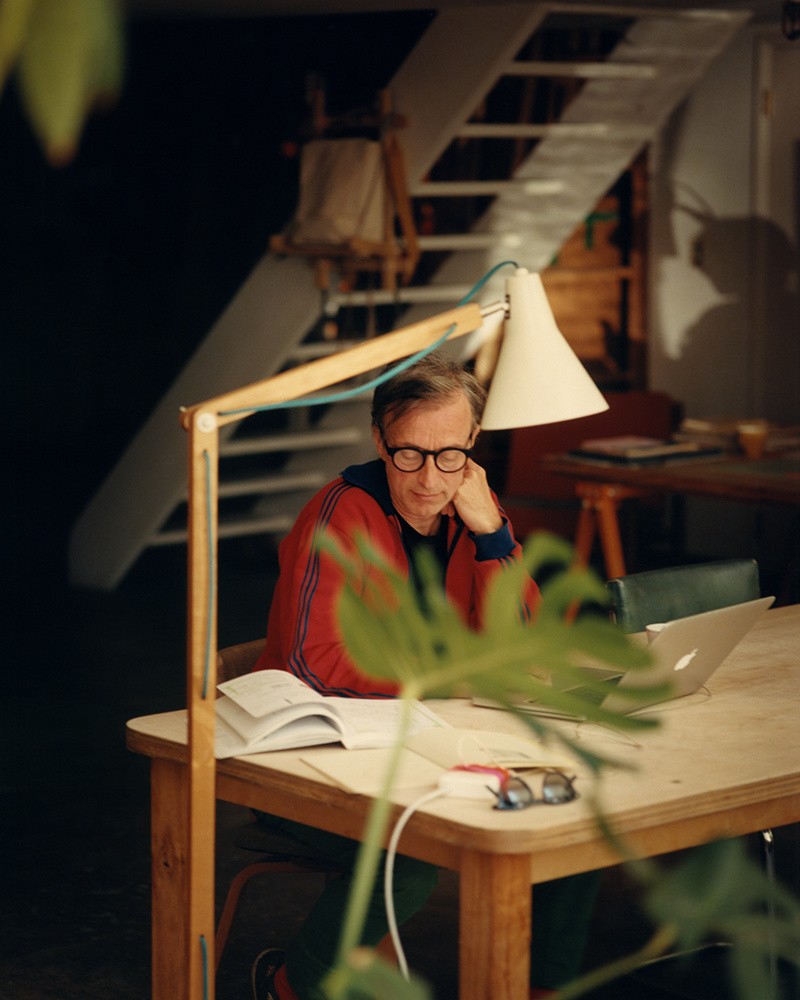
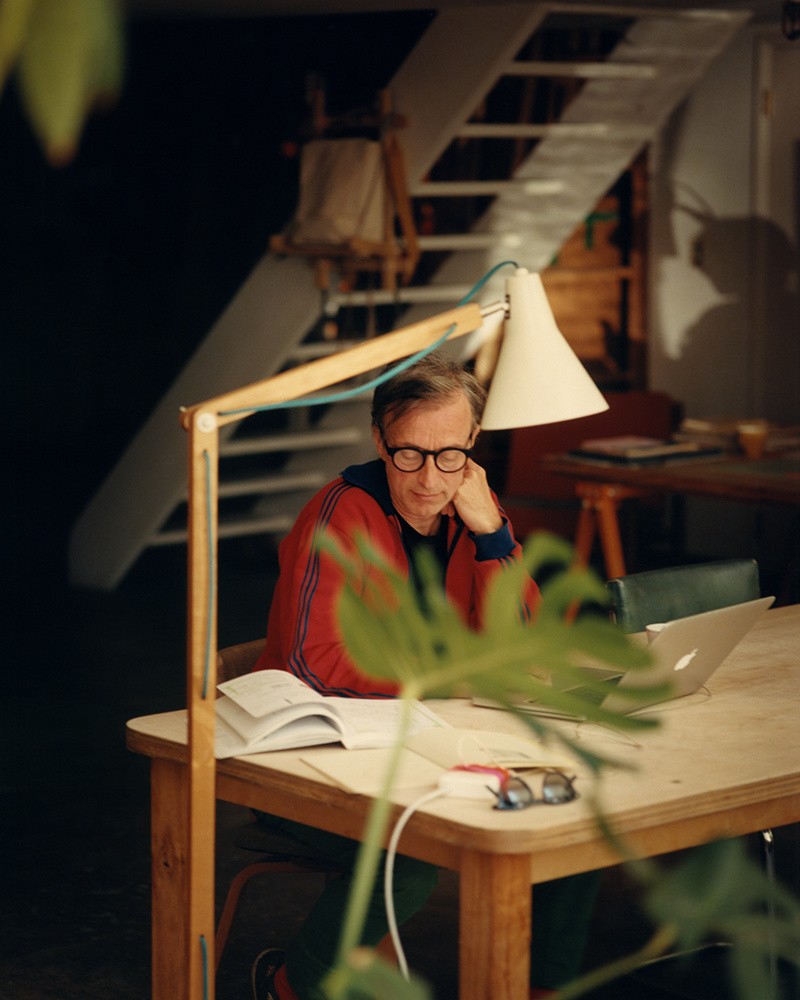
Expert medical annotators
Searching for radiologists, digital pathology experts, or other medical imaging professionals? Our network of expert labelers is skilled in annotating and interpreting medical images. They understand the nuances of various modalities and can provide high-quality, accurate annotations for your specific needs.
Expert medical annotators
Searching for radiologists, digital pathology experts, or other medical imaging professionals? Our network of expert labelers is skilled in annotating and interpreting medical images. They understand the nuances of various modalities and can provide high-quality, accurate annotations for your specific needs.
Expert medical annotators
Searching for radiologists, digital pathology experts, or other medical imaging professionals? Our network of expert labelers is skilled in annotating and interpreting medical images. They understand the nuances of various modalities and can provide high-quality, accurate annotations for your specific needs.
End-to-end project management
We assist in designing custom workflows, implementing quality assurance measures, and overseeing your entire labeling initiative—from scope definition to final delivery. Share your project goals and datasets with us, and we'll help you manage the process, providing regular progress updates.
End-to-end project management
We assist in designing custom workflows, implementing quality assurance measures, and overseeing your entire labeling initiative—from scope definition to final delivery. Share your project goals and datasets with us, and we'll help you manage the process, providing regular progress updates.
End-to-end project management
We assist in designing custom workflows, implementing quality assurance measures, and overseeing your entire labeling initiative—from scope definition to final delivery. Share your project goals and datasets with us, and we'll help you manage the process, providing regular progress updates.
Secure, scalable, cost-effective.
Our platform adheres to SOC 2, ISO 27001, and HIPAA compliance standards for robust data security. We offer flexible team sizing to accommodate projects of various scales, along with competitive pricing models tailored to your specific project requirements.
Secure, scalable, cost-effective.
Our platform adheres to SOC 2, ISO 27001, and HIPAA compliance standards for robust data security. We offer flexible team sizing to accommodate projects of various scales, along with competitive pricing models tailored to your specific project requirements.
Secure, scalable, cost-effective.
Our platform adheres to SOC 2, ISO 27001, and HIPAA compliance standards for robust data security. We offer flexible team sizing to accommodate projects of various scales, along with competitive pricing models tailored to your specific project requirements.
Proof of concept
Send us a subset of your data and tell us about the problem you're trying to solve with AI. Our team will quickly set up a project, demonstrate the tool and workflow, and identify the right annotators for the task. In some cases, our solutions engineers have been able to build and present a proof of concept within hours.
Proof of concept
Send us a subset of your data and tell us about the problem you're trying to solve with AI. Our team will quickly set up a project, demonstrate the tool and workflow, and identify the right annotators for the task. In some cases, our solutions engineers have been able to build and present a proof of concept within hours.
Proof of concept
Send us a subset of your data and tell us about the problem you're trying to solve with AI. Our team will quickly set up a project, demonstrate the tool and workflow, and identify the right annotators for the task. In some cases, our solutions engineers have been able to build and present a proof of concept within hours.
Which programming languages are compatible with the V7 platform?
The V7 platform for medical imaging annotation is compatible with Python. You can use V7's Darwin-py SDK to interact with the platform via the command line interface (CLI) or use it as a Python library. You can find the full documentation for Darwin-py here.
+
What is the pricing for V7's DICOM annotation services?
The pricing for medical image labeling services in V7 can vary depending on factors such as the complexity of the task, the volume of images to be labeled, and the level of accuracy and expertise required. Fill out the Get a Quote form on our website for a more accurate estimate based on your specific needs.
+
Do I need any special hardware to use V7 for DICOM annotation?
There are no special requirements beyond a reasonably modern computer and a stable internet connection. You will need Windows 10, or MacOS Monterrey or above. Also, to avoid performance drops, at least 8GB RAM is needed. V7 supports DICOM natively in 16-bit, which allows you to view images at their original quality. Additionally, V7 offers windowing features that enable you to see beyond what your monitor can typically display.
+
Which formats are used for DICOM annotations in V7?
When exporting annotations, it is recommended to use Darwin JSON 2.0 format or NIfTI format. In Darwin JSON 2.0, annotations from each plane are saved in separate slots, and in NIfTI, exported annotations can be viewed in external 3D NIfTI viewers.
+
What are the best practices for annotating DCM files?
One of the most important parts of successful medical imaging annotation projects is incorporating review and consensus stages in your workflow to validate your annotations. Also, when working with volumetric data, you can leverage orthogonal views for accurate 3D annotation, and use interpolation to create in-between labels, speeding up the process. Lastly, maintaining a well-organized data structure, with separate tags or folders for each modality, body part, and disease, is crucial for an efficient labeling and training process. To find out more, read this guide to data labeling for radiology.
+
Which AI models are available for DICOM annotation in V7?
V7 offers a proprietary auto-annotate model that can automatically segment shapes within a selected area of a DCM file. These shapes can also be interpolated across different slices of a DICOM sequence. You can also use the SAM (Segment Anything Model) enhanced Auto-Annotate feature, which has been improved for accuracy, or contact us to develop a customized and fine-tuned segmentation model for your specific use case.
+
How does V7 handle volumetric DICOM series?
Before uploading a DICOM series to V7, it is recommended to zip the series together outside of V7 and rename the compressed file extension from .zip to .dcm. Once imported to V7, the individual DICOM slices will appear in a series. You can find out more in this guide about annotating DCM files in V7.
+
Which programming languages are compatible with the V7 platform?
The V7 platform for medical imaging annotation is compatible with Python. You can use V7's Darwin-py SDK to interact with the platform via the command line interface (CLI) or use it as a Python library. You can find the full documentation for Darwin-py here.
+
What is the pricing for V7's DICOM annotation services?
The pricing for medical image labeling services in V7 can vary depending on factors such as the complexity of the task, the volume of images to be labeled, and the level of accuracy and expertise required. Fill out the Get a Quote form on our website for a more accurate estimate based on your specific needs.
+
Do I need any special hardware to use V7 for DICOM annotation?
There are no special requirements beyond a reasonably modern computer and a stable internet connection. You will need Windows 10, or MacOS Monterrey or above. Also, to avoid performance drops, at least 8GB RAM is needed. V7 supports DICOM natively in 16-bit, which allows you to view images at their original quality. Additionally, V7 offers windowing features that enable you to see beyond what your monitor can typically display.
+
Which formats are used for DICOM annotations in V7?
When exporting annotations, it is recommended to use Darwin JSON 2.0 format or NIfTI format. In Darwin JSON 2.0, annotations from each plane are saved in separate slots, and in NIfTI, exported annotations can be viewed in external 3D NIfTI viewers.
+
What are the best practices for annotating DCM files?
One of the most important parts of successful medical imaging annotation projects is incorporating review and consensus stages in your workflow to validate your annotations. Also, when working with volumetric data, you can leverage orthogonal views for accurate 3D annotation, and use interpolation to create in-between labels, speeding up the process. Lastly, maintaining a well-organized data structure, with separate tags or folders for each modality, body part, and disease, is crucial for an efficient labeling and training process. To find out more, read this guide to data labeling for radiology.
+
Which AI models are available for DICOM annotation in V7?
V7 offers a proprietary auto-annotate model that can automatically segment shapes within a selected area of a DCM file. These shapes can also be interpolated across different slices of a DICOM sequence. You can also use the SAM (Segment Anything Model) enhanced Auto-Annotate feature, which has been improved for accuracy, or contact us to develop a customized and fine-tuned segmentation model for your specific use case.
+
How does V7 handle volumetric DICOM series?
Before uploading a DICOM series to V7, it is recommended to zip the series together outside of V7 and rename the compressed file extension from .zip to .dcm. Once imported to V7, the individual DICOM slices will appear in a series. You can find out more in this guide about annotating DCM files in V7.
+
Which programming languages are compatible with the V7 platform?
The V7 platform for medical imaging annotation is compatible with Python. You can use V7's Darwin-py SDK to interact with the platform via the command line interface (CLI) or use it as a Python library. You can find the full documentation for Darwin-py here.
+
What is the pricing for V7's DICOM annotation services?
The pricing for medical image labeling services in V7 can vary depending on factors such as the complexity of the task, the volume of images to be labeled, and the level of accuracy and expertise required. Fill out the Get a Quote form on our website for a more accurate estimate based on your specific needs.
+
Do I need any special hardware to use V7 for DICOM annotation?
There are no special requirements beyond a reasonably modern computer and a stable internet connection. You will need Windows 10, or MacOS Monterrey or above. Also, to avoid performance drops, at least 8GB RAM is needed. V7 supports DICOM natively in 16-bit, which allows you to view images at their original quality. Additionally, V7 offers windowing features that enable you to see beyond what your monitor can typically display.
+
Which formats are used for DICOM annotations in V7?
When exporting annotations, it is recommended to use Darwin JSON 2.0 format or NIfTI format. In Darwin JSON 2.0, annotations from each plane are saved in separate slots, and in NIfTI, exported annotations can be viewed in external 3D NIfTI viewers.
+
What are the best practices for annotating DCM files?
One of the most important parts of successful medical imaging annotation projects is incorporating review and consensus stages in your workflow to validate your annotations. Also, when working with volumetric data, you can leverage orthogonal views for accurate 3D annotation, and use interpolation to create in-between labels, speeding up the process. Lastly, maintaining a well-organized data structure, with separate tags or folders for each modality, body part, and disease, is crucial for an efficient labeling and training process. To find out more, read this guide to data labeling for radiology.
+
Which AI models are available for DICOM annotation in V7?
V7 offers a proprietary auto-annotate model that can automatically segment shapes within a selected area of a DCM file. These shapes can also be interpolated across different slices of a DICOM sequence. You can also use the SAM (Segment Anything Model) enhanced Auto-Annotate feature, which has been improved for accuracy, or contact us to develop a customized and fine-tuned segmentation model for your specific use case.
+
How does V7 handle volumetric DICOM series?
Before uploading a DICOM series to V7, it is recommended to zip the series together outside of V7 and rename the compressed file extension from .zip to .dcm. Once imported to V7, the individual DICOM slices will appear in a series. You can find out more in this guide about annotating DCM files in V7.
+
Next steps
Label medical data with V7.
AI for radiology, WSI, and more.
Try our free tier or talk to one of our experts.
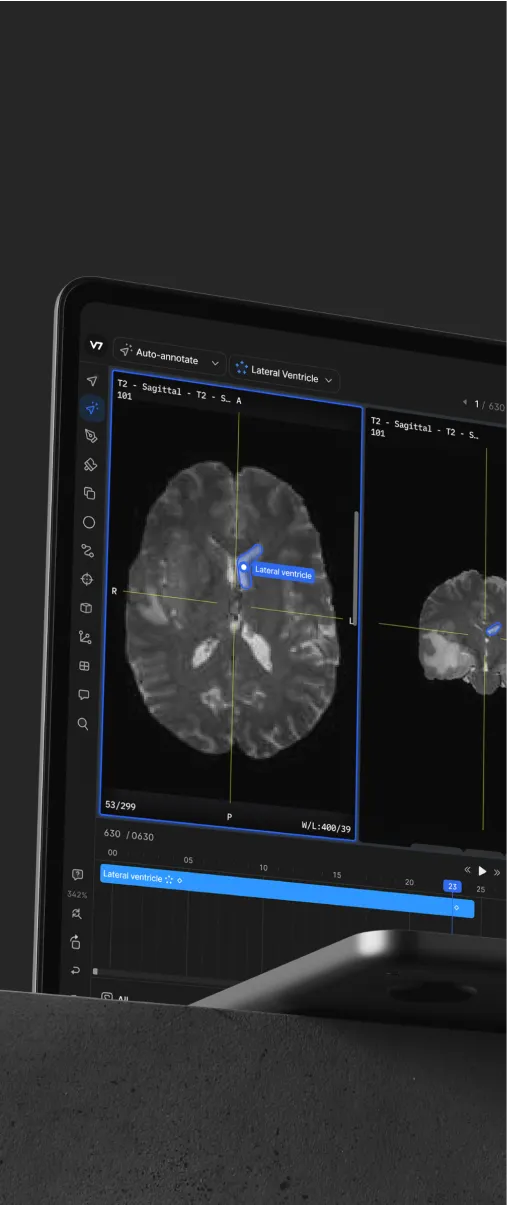
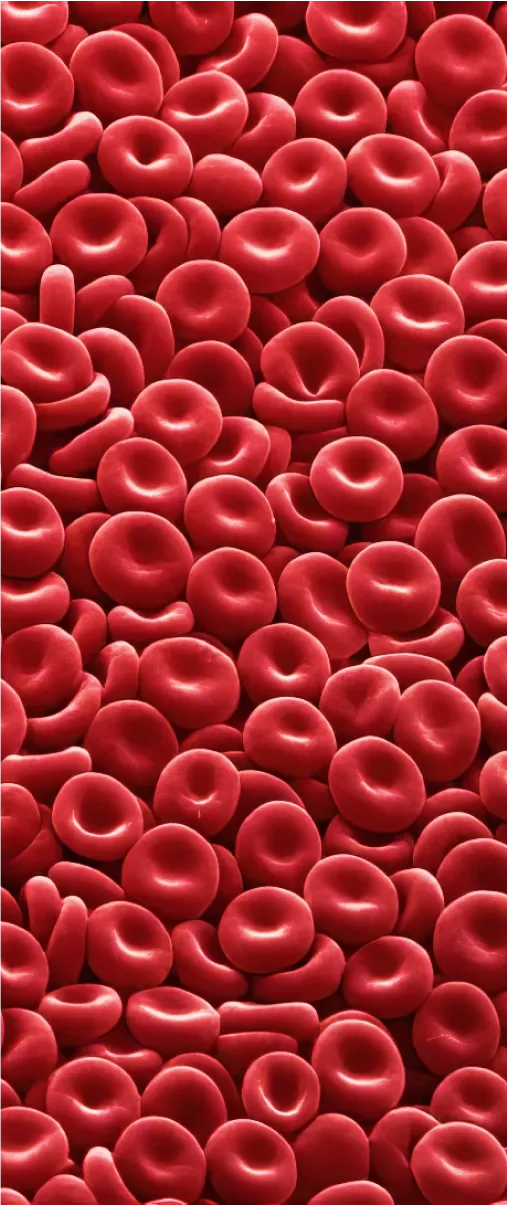
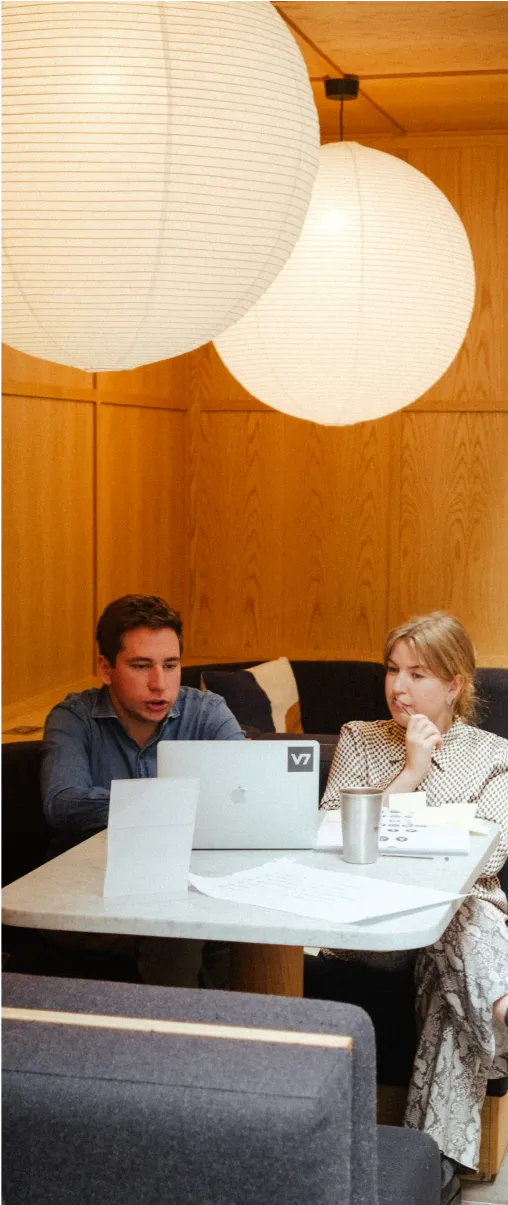
Next steps
Label medical data with V7.
AI for radiology, WSI, and more.
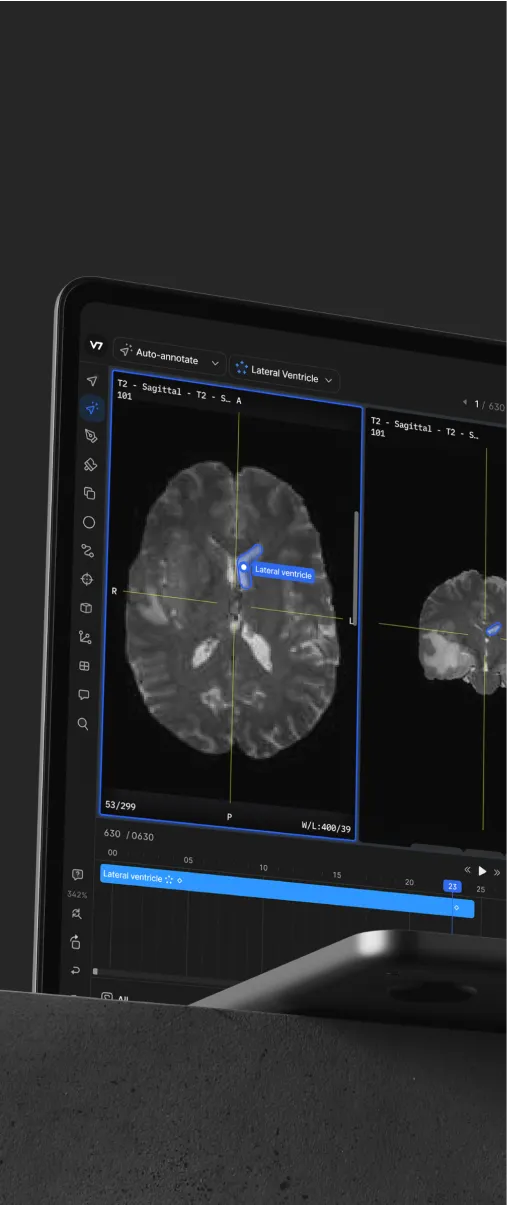
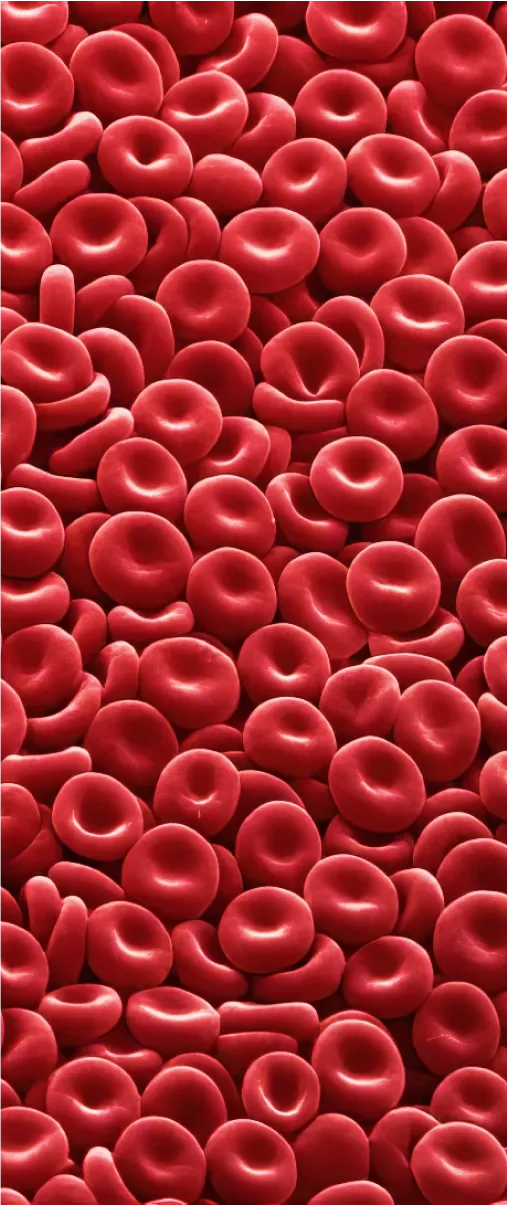
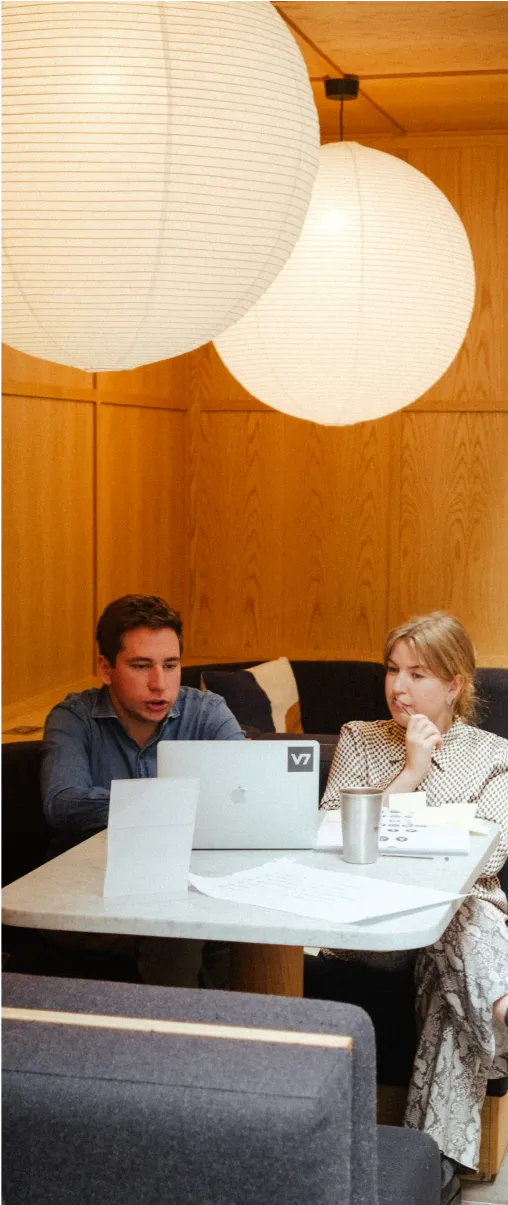