AI implementation
7 Game-Changing AI Applications in the Sports Industry
14 min read
—
Oct 1, 2021
Artificial Intelligence in sports is emerging all over the industry, covering post-game analysis, in-game activity - and even the fan experience. Here are some of the most exciting AI use cases in sports that you should know about. Read on!
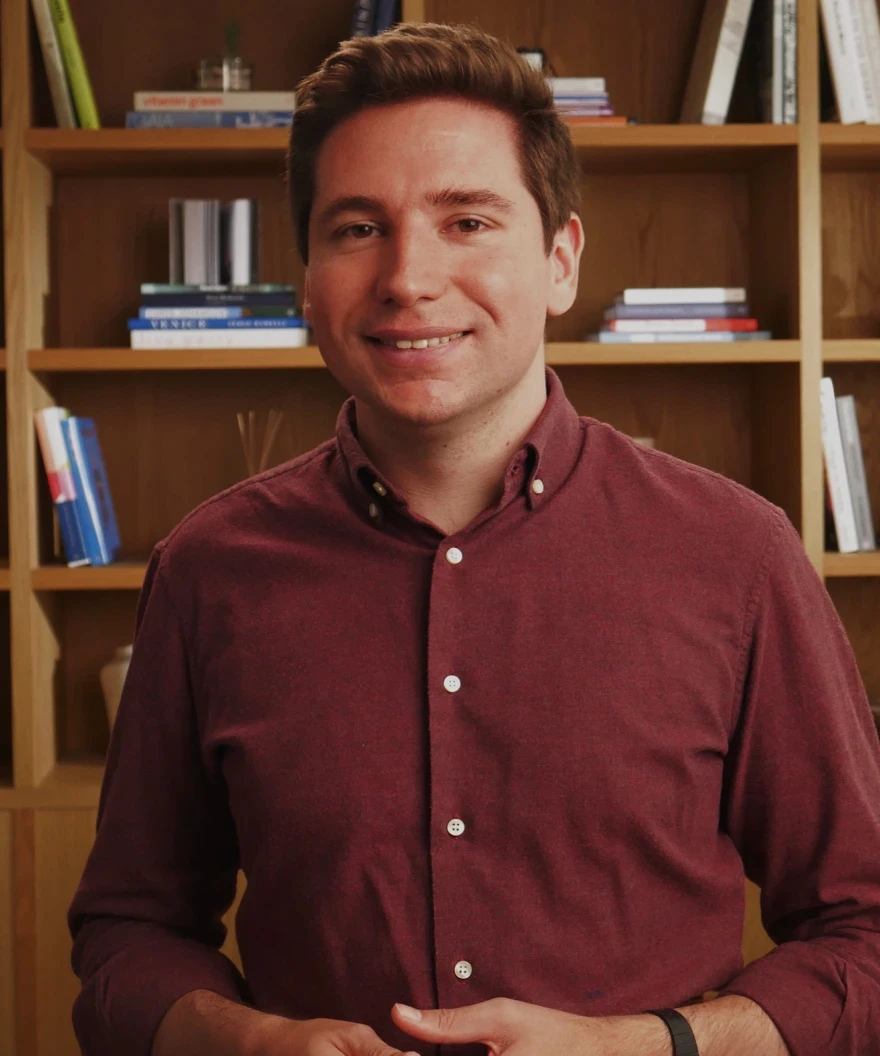
Co-founder & CEO
For the last two decades, coaches have been using data science in sports to help improve the performance of their players.
They’ve been leveraging big data to help them make split second on-the-field decisions, and relying on sports analytics to help them sign the “next big thing”.
Referees, meanwhile, now use Video Assistant Technology (VAR) in football to help them make more precise judgments regarding the big decisions, such as penalties, free kicks, and red cards.
And now that AI, and specifically Deep Learning, has got involved, the sports experience is going to change even more.
Here’s what we’ll cover:
AI Referee
Real-time, personalized training plans
Player performance
Automated scouting and recruitment
Match predictions
Ticketing
Sports journalism
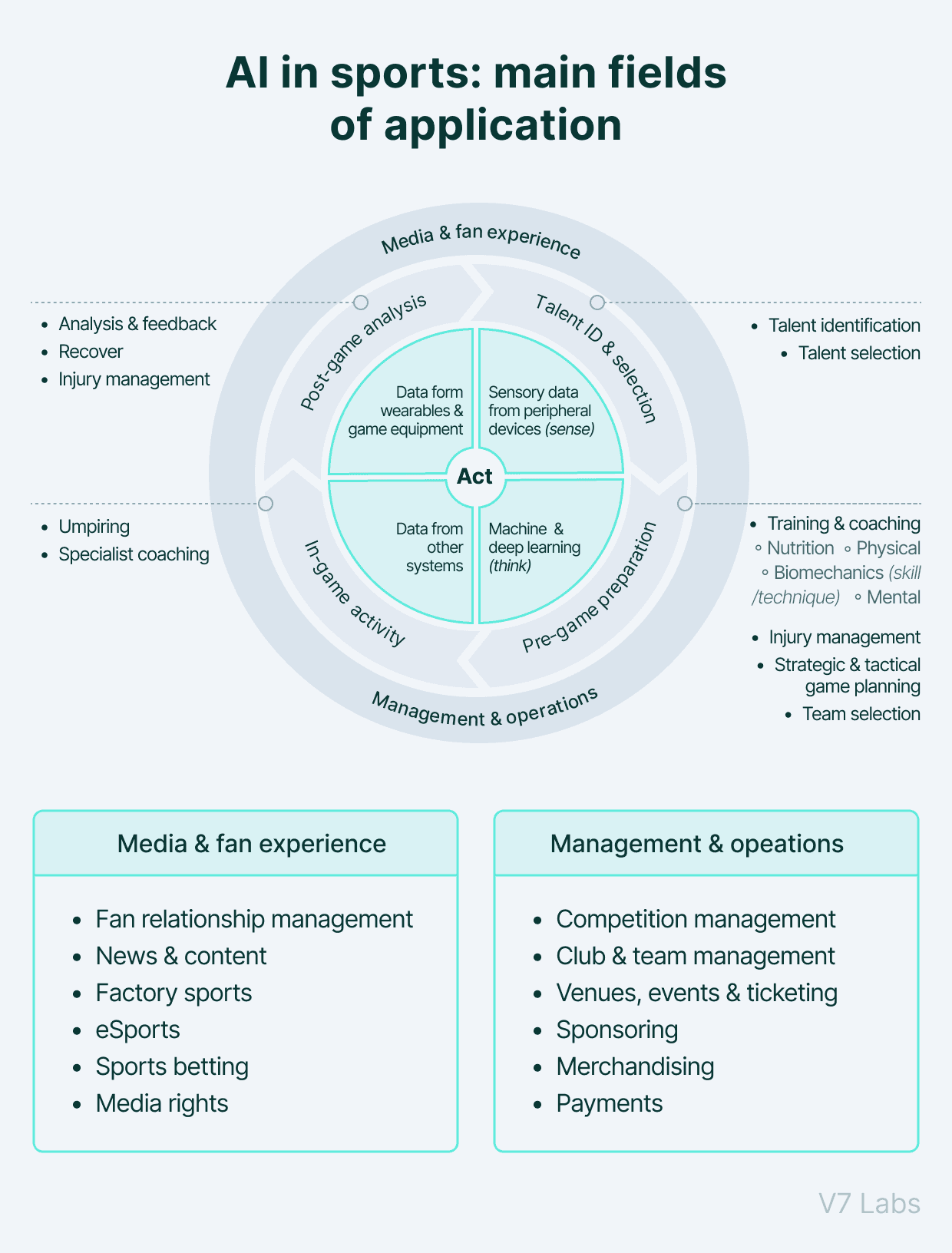
AI Referee
It was the 1986 World Cup.
Argentina was drawing 0-0 with their old enemy, England, in the sweltering Mexican heat when the pint-sized Diego Maradona rose with the England goalkeeper to meet a cross.
The keeper was the favorite to reach the ball—after all, he was able to use his arms.
And yet, somehow the impish Maradona sprung into the air faster, met the ball with his head—and nodded the ball into the net.
Of course, anyone who knows football knows that isn’t exactly what happened.
From the moment Maradona connected with the ball, the England defenders were apoplectic with rage, gesturing to the referee that the Argentinian superstar had scored an illegal goal with his hand.
Today, such a goal would have been chalked off with the use of AI Referees in just a matter of minutes. All big decisions that change the outcomes of games would be judged more accurately, the margin for error would be a lot slimmer—and, arguably, controversies would be hugely reduced if AI in sports was used to help the referees.
You don't have to look far for confirmation—the Euro 2020 game between England and Denmark with the questionable penalty called when Raheem Sterling went down near the goal, is the perfect example. What would AI decide?
The AI Referee verdict, based on the model trained on V7, confirmed that: "any "contact" with Sterling happened 150ms before Denmark would have reached the ball. An AI ref would have still given the penalty to England.
However—
With more camera angles than 2 available for analysis, the outcome may have been different.
Naturally, ever since sports have existed the outcome of a game has often been influenced by the so-called fine margins—line calls in tennis handballs in football, and offensive fouls in the NBA. And for many years, thousands of incidents have been called incorrectly by the referees, leading to anger from coaches, fans, and clubs.
The situation has hardly improved over the years.
In fact, research shows shows that:
Since March 2015 to June 2018, the NBA viewed 26,822 plays from 1,476 games. In those 4,297 minutes of action, the officials have missed or incorrectly called 2,197 plays, or about 8.2% of all calls reviewed. This amounts to 1.49% wrong decisions in the final minutes of each close game.
I know, 1.49% might not sound like a lot—but in sport, it is huge.
It can be the deciding factor between a team winning or losing, especially in a game like NBA where matches often go right down to the wire. Every point matters—and every refereeing call matters.
Sport is at the point now where it’s ready to embrace AI, not to change the outcome of sporting events necessarily, but to improve the decision-making process.
Offside detection from overhead cameras, for example, can assist VAR in football to ensure goals are not correctly or incorrectly given or denied, while on-field tracking systems (such as Hawkeye) can detect tennis balls so that line calls are judged more accurately.
This is especially true in clay tennis, where technology still isn’t able to give a 100% accurate reading of a line call, thanks to the slower movement of the ball.
Computer Vision can also assist in identifying potential penalties across sports so as to reduce mistakes, controversies and prevent games from swaying one way or another due to a poor refereeing decision.
Goal-line technology, meanwhile, was brought into football after England was denied a clear equalizer versus Germany at the 2010 World Cup. As per FIFA’s definition, it is “a technical means of instantly determining whether the whole of the ball has crossed the line.”
The technology uses magnetic fields and cameras to great effect. Interestingly, there are various criteria that GLT needs to meet in order to gain FIFA’s approval, and this includes being able to function accurately “under adverse conditions.”
A typical GLT relies on 14 strategically positioned cameras that are streamed to a cluster of computers, who use advanced image processing algorithms to a) determine the object (ball), b) distinguish it from interferences, such as a player’s boot, and c) confirm whether the whole of the ball has crossed the line.
Personalized training and diet plans
Training and diet plans have improved immeasurably over the years for professional sportsmen and women. Back in 1990s Britain, it wasn’t unusual for a British footballer to hit the pub after training, sink several pints and grab a kebab on the way home. It was normal.
However, the introduction of Artificial Intelligence in sports can help to take the personalization of training and diet plans to the next level. Research has already shown promising results regarding the use of AI in weight training.
The data was based on measurements from 15 rather inexperienced participants performing 3-5 sets of 10-12 repetitions on a leg press machine. The assessment made by professional trainers (using video analysis) showed good performance and prediction outcomes. It indicated the feasibility and potency of AI techniques in assessing performance on weight training equipment automatically and providing sportsmen with prompt advice.
Essentially, an AI diet plan uses machine learning to personalize different plans to different players based on their needs and their current situation (is there a match the next day? Is today a recovery day?)
Apps like FoodVisor are already using deep learning—specifically object recognition—to identify more than 1,200 types of food, estimate the amount, and produce a quick report on the nutritional breakdown.
And that's just the tip of the iceberg!
Let's not forget about the AI-assisted fitness apps that are flooding the market.
Thanks to the computer vision technique called human pose estimation, we can now train algorithms that are able to detect human poses in real-time.
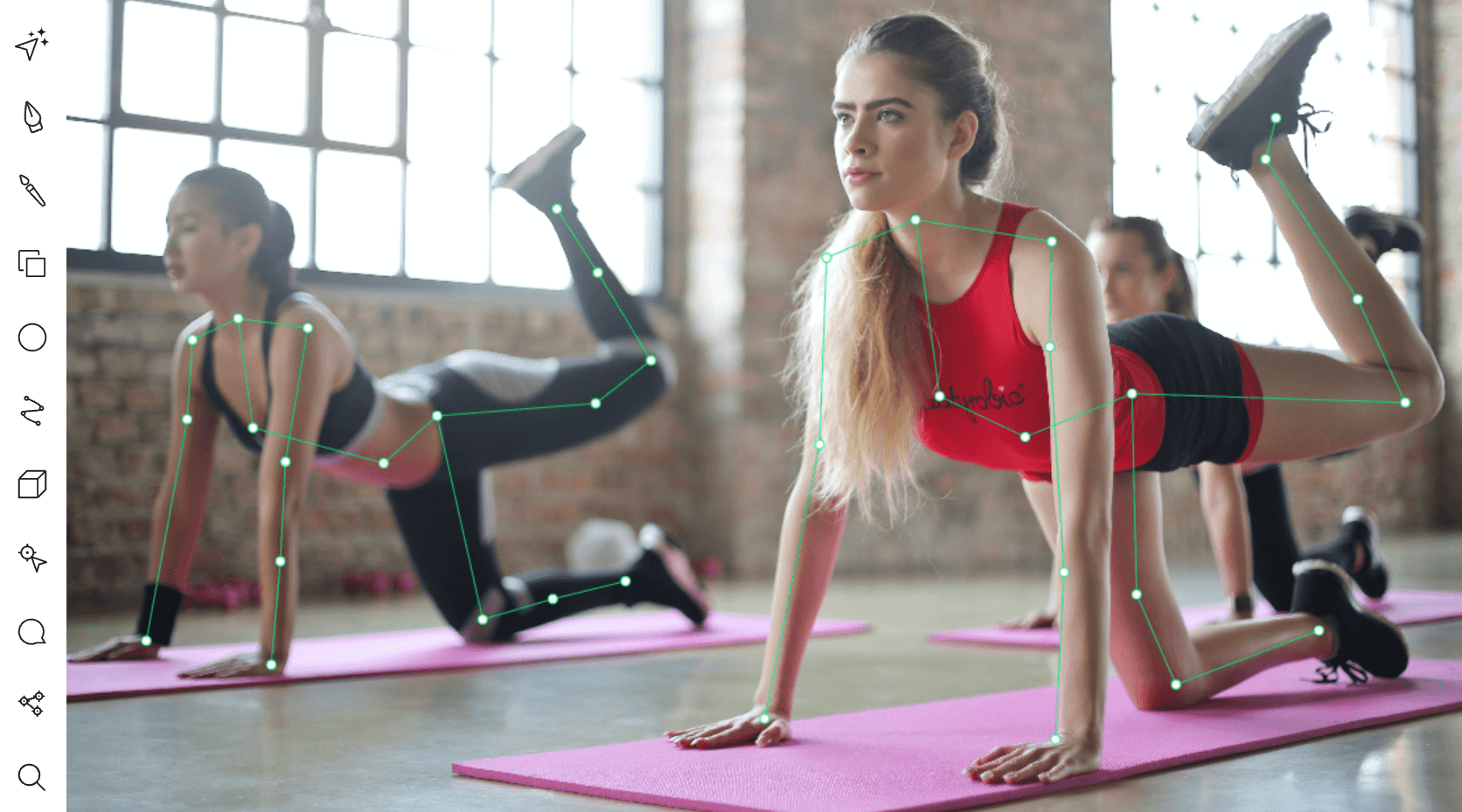
This technology found applications in fields like online yoga and pilates where keypoint skeleton models can be used to identify human joints and provide the user with guidance on how to exercise the right way.
By the way, if you'd like to get hands-on experience annotating data for your Human Pose Estimation projects, make sure to check out the video below.
Player performance
AI is used in sports for boosting performance and health thanks to predictive analysis. With the advent of wearables that gather information about strain and tear levels, athletes can avoid serious injuries. But that’s just the beginning. AI can help teams shape strategies, tactics, and maximize their strengths.
The way player performance is analyzed is now more sophisticated than ever before thanks to the AI. Using data and visuals, coaches are able to gain insights into their teams’ strengths and weaknesses on any current day, allowing them to make changes to tactics and strategy, thereby exploiting any weaknesses of their opponent.
This is true of all sports—from football to tennis, to even handball and swimming.
Computer vision, for example, is used for human motion tracking and analysis. In handball, the development of a computer system for tracking players has caught the attention, with a study describing the process:
The cameras are collecting data and the output result will be spatio-temporal trajectories for all players. These player trajectories provide the sports expert with valuable data of the player capabilities and performance.
Using video sequences, they ran three experiments to identify the best method for the automated player tracking of:
Tracking using just motion detection
Colour tracking
Combination of colour and template tracking
The result: C—The combination of colour and template tracking proved to be the most suitable, thanks to its speed and minimum human operator intervention compared to other methods.
This is how player tracking would look like using bounding boxes for object detection.
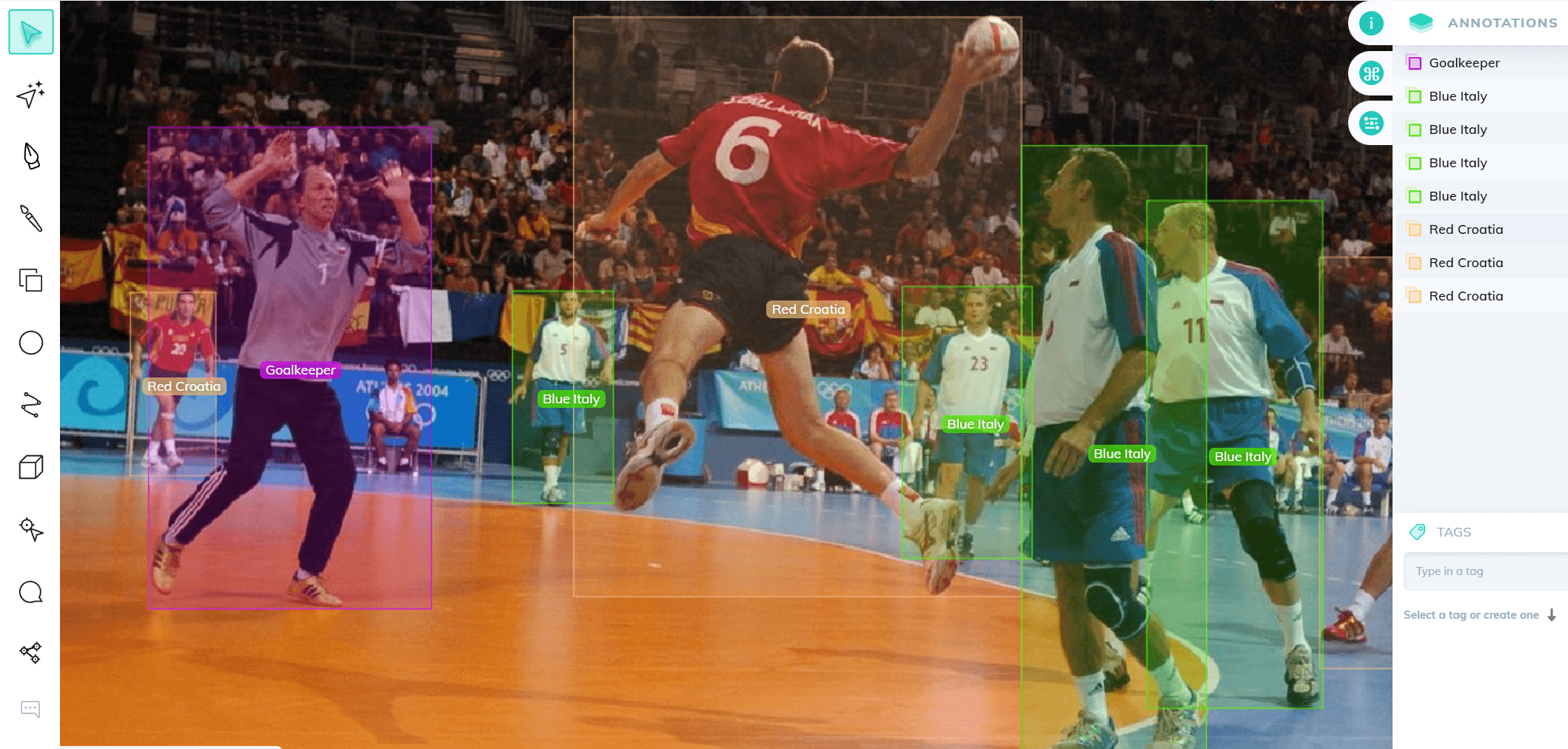
Check out 9 Essential Features for a Bounding Box Annotation Tool if you are looking for one.
In swimming, meanwhile, human pose estimation has been used to analyze swimmers’ performance using single stationary cameras above and below the water filters. This method will eventually take over the quantitive evaluation method, which previously relied on manual annotations of body parts in each video frame (very time-consuming).
The global player tracking market is expected to grow at a CAGR of 24.9% over the next 5 years, with teams in both elite and even minor leagues able to use tracking solutions that will help them understand their players’ movements.
This will not only enable them to make better tactical switches, but it will also highlight who’s putting in the effort (and who isn’t), and which areas of performance—both offensive and defensive—can be improved upon.
4. Scouting and recruitment
These days, teams are taking things further by adding Artificial Intelligence to their scouting and recruitment box of tricks. Everything that happens on the field of play—swings in baseball, runs in football, blocks in basketball—is tracked, with teams amassing terabytes of data.
Indeed, computer vision in sports is used to track players’ movements and the orientation of their bodies during play (object tracking in videos).
Here's how it looks like on an example of soccer players. Labeling data with keypoint skeleton tool and then training the model will allow it to track and then predict players' movements.
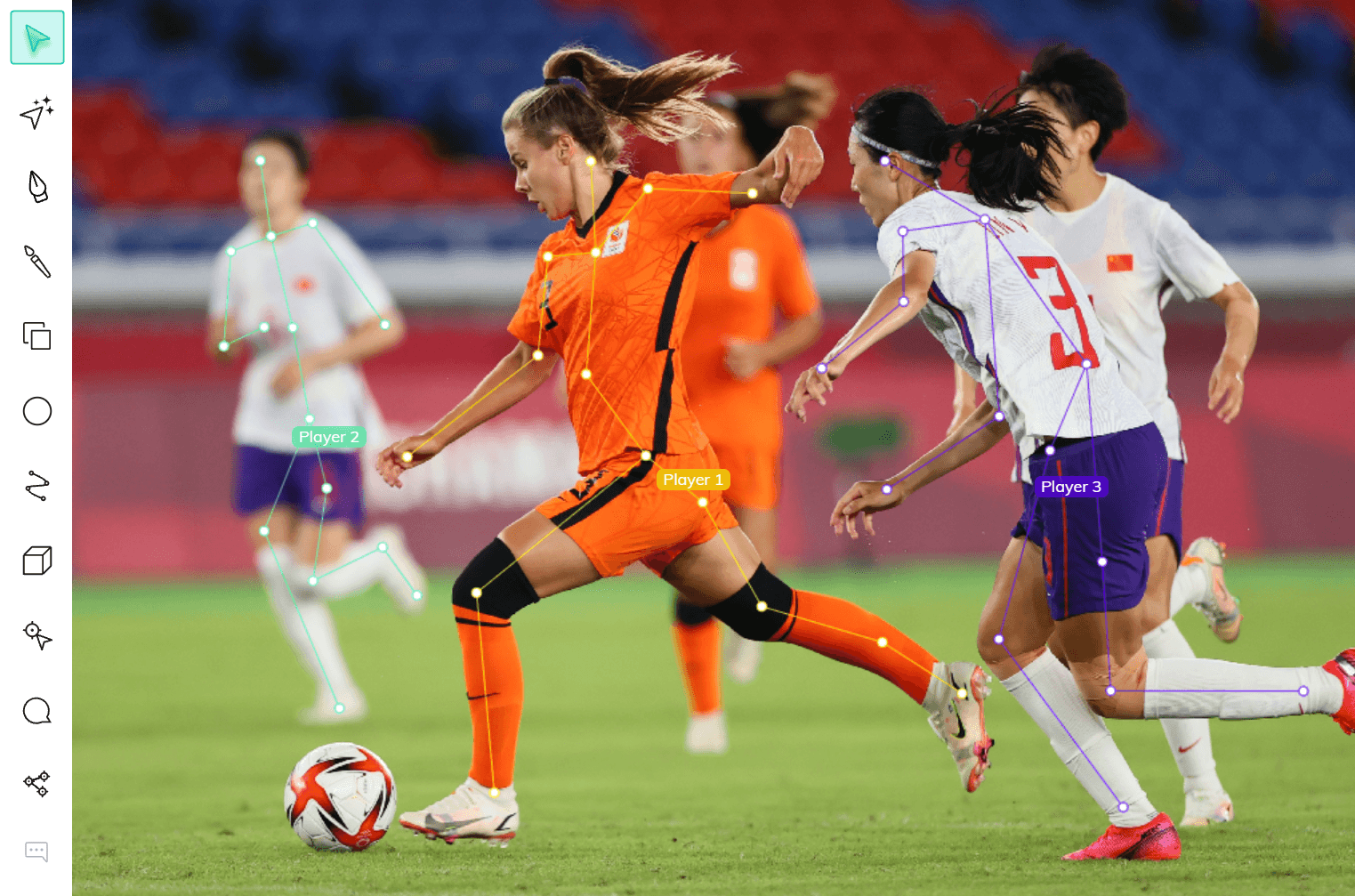
Essentially, Machine Learning algorithms use aggregated data to “evaluate players’ skills and overall potential and rank them in various categories.
For example, offensive forward in the NHL and point guard in the NBA.
Teams can also use computer vision to spot specific attributes—such as corner-taking abilities in soccer—that help determine future performance.
The insights this data gives teams allows them to make better recruitment choices going forward that ensure they build a successful team and buy untapped players for cheap, before developing them and selling them for a profit at a later date.
Brentford did exactly this with striker Ollie Watkins, who was bought for around $2,000,000 and sold for $35,000,000.
Pro Tip: Another use of AI emerging for recruitement practices is contract reviews using GenAI
5. Match predictions
Bettors have for years been trying to process a heap of data in a bid to predict the outcome of future matches and win lots of money. They’ve examined first and second serve percentages in tennis, the number of aces, backhand winners, and so on in an effort at predicting sports outcomes.
Ultimately, though, a human can’t process as much data as an AI-driven football algorithm prediction can, and neither can they accurately predict enough matches so as to become millionaires (ha). They’re always hamstrung by their human limitations—and thus most won’t become millionaires.
AI can’t accurately predict the outcome of every single match either. But it can get much closer than a human can with a predicting algorithm.
For example, computer vision is used to determine ball possession time.
Researchers ran multiple configurations of the pos-N-M model on a manually labeled set of 4,000 frames and achieved an 85.5% accuracy on a test. This was despite several issues, such as the ball being small and not very visible, or the ball at times being covered by a player.
When computer vision is able to be trained properly as a ball possession model, it can help to predict future match results.
Computer vision can also be used to collect and analyze data based on:
Number of passes between teammates
A team’s formation
Number of goals scored
Chances created
Key passes that led to a goalscoring opportunity
Using this data, the model can forecast whether a team will win, lose or draw a future match.
Curious to learn more about computer vision use cases? Check out 27+ Most Popular Computer Vision Applications.
6. Ticketing
Even in 2021, elite sports teams were experiencing entry delays. Southampton FC had to refund their fans after thousands were left stranded outside after the game had begun.
Fans struggling to get inside stadiums on time for the match is nothing new at big sporting events. Indeed, it’s been happening for decades. Nothing has resolved the issue—until now (potentially).
Columbus Crew, for example, is using facial recognition to allow their fans to enter the stadium without having to display their ticket.
Wicket’s facial ticketing platform lets fans upload an image of their faces and the barcode number for their tickets to a game.
This makes stadium entry way more efficient and prevents bottlenecks. Moreover, it will also prove extremely useful at enticing COVID-wary supporters back inside stadiums who don’t want to get stuck in lengthy queues.
Computer vision, meanwhile, is on hand to “monitor crowd density inside the stadium, with staff receiving alerts when certain areas get overcrowded.”
Of course, it’s rare in modern sports that overcrowding happens—especially not on a scale once seen in 1980s European football, where deadly crushes happened more than once. However, with British football clubs set to introduce standing sections for the first time since the early 1990s, the use of computer vision to monitor crowd density will provide an extra layer of safety and security that can prevent crushes.
Predictive analytics can also forecast what attendance is likely to be, as well as when supporters might be expected to turn up. This is helpful for arranging merchandise and food and keeping up with demand.
6. Automated sports journalism
Sports journalism is big business. But in many elite sports—and especially baseball, soccer, and tennis—there is so much to cover.
Some would even say there is too much to cover, especially when it comes to statistics and data in the minor leagues and tournaments that are taking place almost daily all over the world.
Such exhaustive workloads in other sectors are now being eased by AI—and this is also the case in sports journalism.
Wordsmith, for example, is an AI-driven platform that translates hard data from MiLB into narratives, using natural language. Developed by Automated Insights, it has helped AP increase its reporting capacity to cover 13 leagues and 14 MLB-affiliated teams.
Then there’s the small matter of match reports. Each day, there are hundreds of soccer matches taking place—sometimes, there are thousands. To cope with the demand, AI bots are now being used to write accurate match reports that explain the key events, offer statistics and data, and keep the reader engaged by sustaining the required drama.
And while some might argue that “AI is taking all our jobs,” check this out: Dutch regional media group NDC is using AI to help it cover 60,000 soccer matches in one year, which basically amounts to every single local match.
This is an astonishing feat and one that couldn’t reasonably be achieved without AI.
Therefore, with the benefit of AI, local matches are now being covered which means teams, coaches, players, and fans who would normally be absent from news pages are getting their moment in the sun.
Fascinated by AI? Check out AI in Healthcare, AI in Insurance, and AI in Retail learn more.
AI Sports Industry: Key takeaways
Machine learning in sports is growing at a rapid rate, with new developments and applications emerging each year. Everything barring the games themselves is set to be improved via data and technology.
Of course, it’s all about balance.
Implementing AI in sports sounds good in theory, but it can’t come at a cost of the fan experience. For example, while using facial recognition to allow fans into the stadium without them even needing a ticket prevents bottlenecks and gets fans into stadiums faster, but it also removes the turnstile which—for many European football fans, at least—is synonymous with a romantic vision of match day.
The future of sports technology, however, undoubtedly lies with AI. But we’re certainly not at the final frontier just yet—in fact, we’re probably only at the beginning. And that’s exciting.
Read more:
Data Annotation Tutorial: Definition, Tools, Datasets
3 Signs You Are Ready to Annotate Data for Machine Learning
An Introductory Guide to Quality Training Data for Machine Learning
13 Best Image Annotation Tools
And if you are interested to learn more about AI applications across other industries, check out: