AI implementation
9 Innovative Use Cases of AI in Finance [+Pros & Cons]
16 min read
—
Aug 31, 2022
Artificial intelligence is changing the finance industry. Learn about different applications of AI in finance, from fraud detection to algorithmic trading.
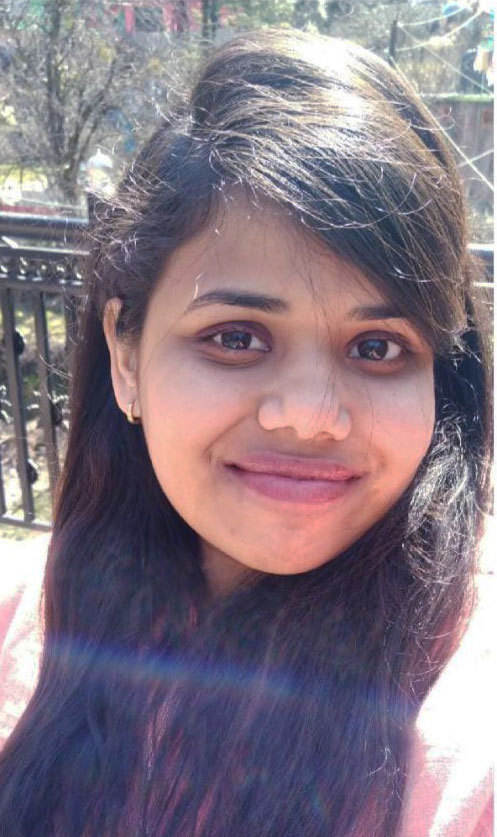
Guest Author and Software Developer
According to a report from Mordor Intelligence, artificial intelligence (AI) in finance is expected to register a compound annual growth rate (CAGR) of over 25% between 2022 and 2027.
What does this mean?
In a nutshell, the sector is going to grow very, very quickly. And if a financial institution hasn’t been dipping its toes in AI waters yet, chances are it’s already lagging behind the competition.
But how exactly can AI help the financial sector? Well, if you can’t think of at least one AI application in finance, you must have been living under a rock.
But don’t worry—this article is here to shed some light.
Here’s what we’ll cover:
Benefits of using AI in banking and finance
Nine innovative use cases of artificial intelligence in finance
Challenges facing AI in finance
Artificial Intelligence in the Financial Industry—Opportunities
The COVID-19 global crisis has accelerated and heightened the digitalization trend, including the application of AI in the finance industry.
Over the 2020–2030 decade, global spending on AI is anticipated to double, rising from USD50 billion in 2020 to more than USD110 billion in 2024. A recent forecast by Business Insider shows that AI in finance can save banks and corporate institutions $447 billion by 2023.
AI usage in finance is expected to grow as it helps financial firms gain a competitive edge in two primary ways:
Efficiency boost. AI helps companies to reduce costs and enhance productivity, which leads to higher profitability.
Higher customer satisfaction. Thanks to AI, new highly-customized product offerings are becoming available to a growing number of consumers.
Check out What Is Machine Learning? [Ultimate Beginner’s Guide]
Use Cases for AI in the Finance Industry
Now let’s dive into some of the most innovative applications for AI in financial services.
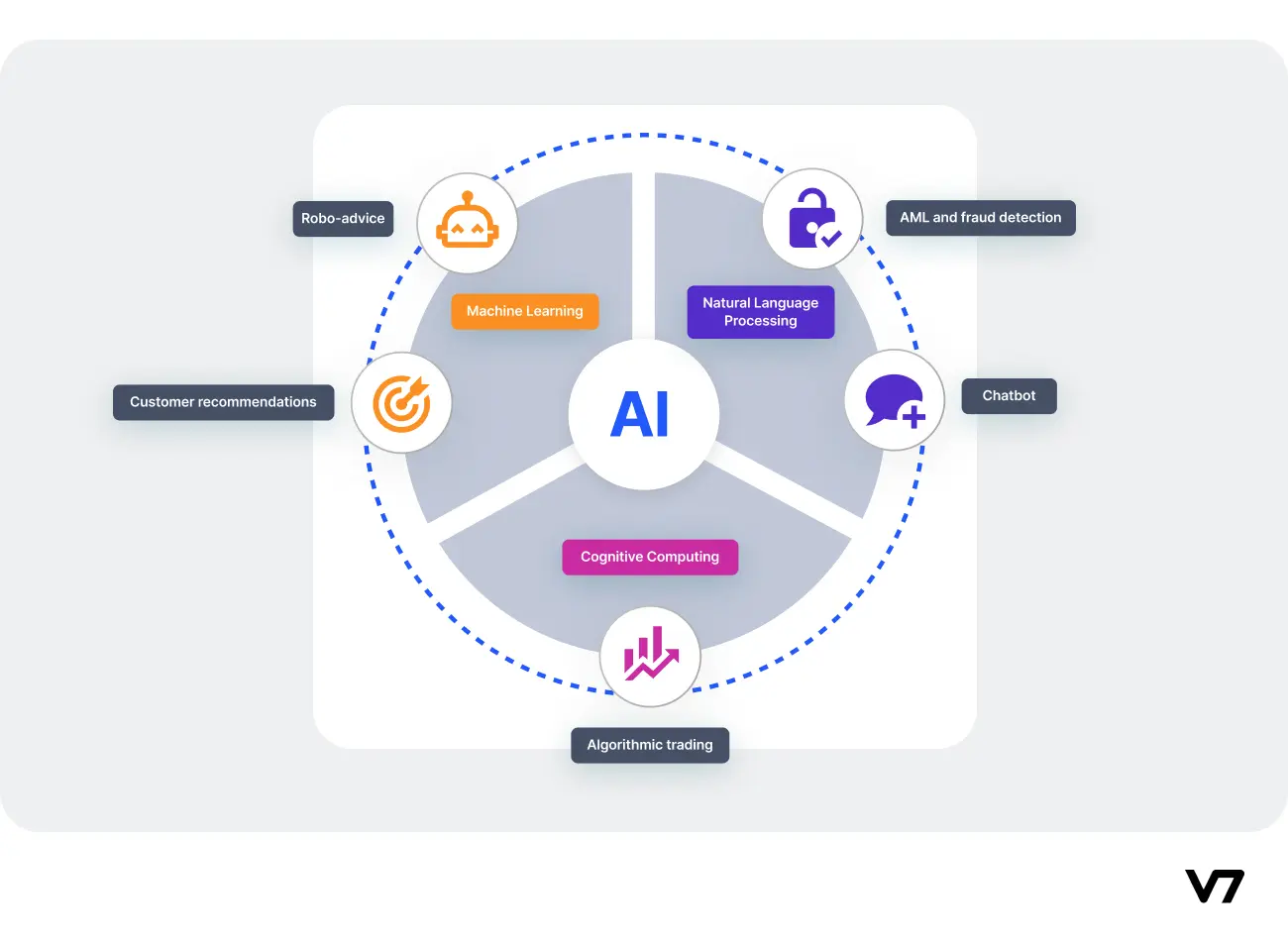
Personalized Banking Experience
Delivering a context-based customer experience is no longer a nice-to-have option. It’s a must-have that all institutions need to deliver in the increasingly competitive world of banking and finance.
The introduction of chatbots and virtual assistants—byproducts of the AI revolution in the finance industry—has minimized wait times and sped up customer service. Customers can easily check their account balance, plan monthly payments, or review their bank account activity.
But the applications of AI in banking go well beyond cutting down on the amount of manual work.
Artificial intelligence has become part and parcel of the authentication process. Customers can now effortlessly log into their banking apps by simply looking at their phones. All this is thanks to advances in machine learning and the development of cutting-edge neural engines that run on mobile phone chips.
Moreover, AI can now analyze user activities and data collected by other non-banking apps and offer customized financial advice. In fact, such banks as DBS or Royal Bank of Canada (RBC) have already embraced such AI-based tools.
RBC has developed a platform called NOMI that helps the bank’s customers automate savings and effectively manage their monthly budgets. The platform has 1.5 million active users, 53% of whom consider it a game-changer for their finances.
One of NOMI’s flagship features is called Find&Save. The bank estimates it has helped its customers save about 1.9 billion dollars by rounding up expenses and automatically transferring small change to savings accounts. The feature is built on an ML algorithm that, for example, rounds up the price of a latte from $3.65 to, say, $3.90 and deposits the extra 25 cents—the amounts saved are all based on a given customer’s financial habits and ability.
See how V7 can help you advance AI in your organization: The Data Engine for Insurance & Finance
Credit Scoring
AI is used in finance to offer a solution that can potentially transform how we allocate credit and risk, resulting in fairer, more inclusive systems.
According to Forbes, 70% of financial firms are using machine learning to predict cash flow events and adjust credit scores.
But AI can’t rely on real-time data for training due to the already introduced bias in the current system. Some recent studies show that predictive systems trained on real people's mortgage data skew automated decision-making in a way that disadvantages low-income and minority groups. The difference in the approval rate is not just due to bias, but also due to the fact that minority and low-income groups have less data in their credit histories.
AI finds application in enabling better credit systems by developing a system where lenders can more correctly determine a borrower's risk with the aid of AI regardless of the social-demographic conditions.
This can be accomplished by looking at real-time indicators that aren't considered in a typical credit score, such as whether the borrower spends their money on necessities or luxuries, their income level, employment opportunities, and potential to earn.
AI may also assist lenders in identifying less visible risk characteristics, such as whether a borrower exploits their available credit.
Leading FinTech companies like JP Morgan have made it clear that the future of customer-centric financial services lies in crunching vast amounts of data drawn from varied sources—often non-traditional. J.P. Morgan has recently summarized critical research in machine learning, big data, and artificial intelligence, highlighting exciting trends that impact the financial community.
Check out An Introductory Guide to Quality Training Data for Machine Learning to learn more.
AI might eventually be able to completely replace current mathematical credit scoring systems that get a lot of flak for being outdated—primarily because of their standardization and lack of sensitivity to individual disparities and nuances.
Fraud Detection and Risk Management
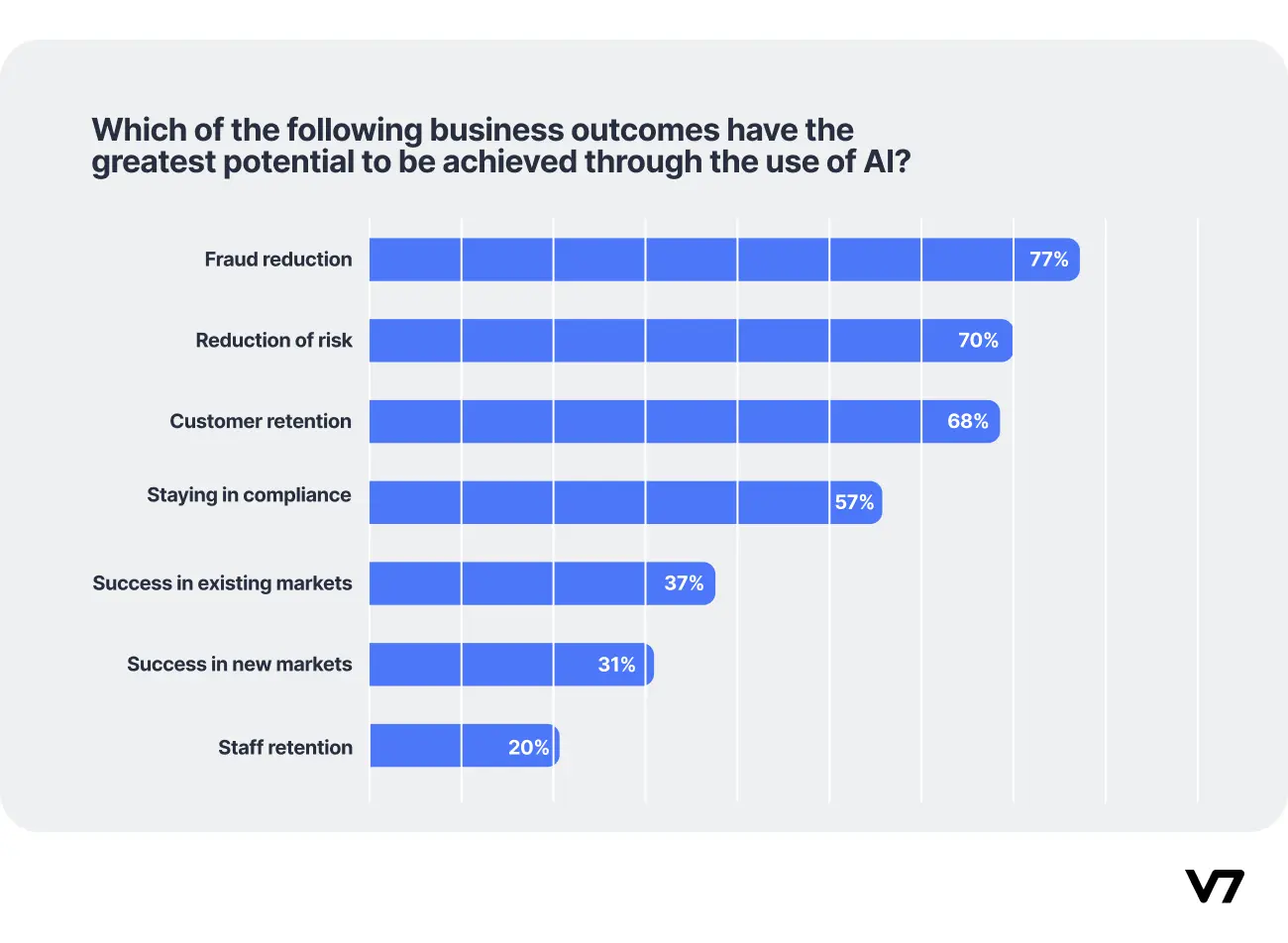
Thanks to their fraud detection capabilities, AI-based systems help consumers minimize the risk and save money from fraudulent activities.
AI applications in the fintech industry range from recognizing abnormal transactions to identifying suspicious and potentially fraudulent activities by analyzing massive amounts of data. AI can quickly gain insights that help protect organizations against losses and increase ROI for their customers.
Additionally, AI and Cognitive ML models can decrease the likelihood of false positives or the rejection of otherwise legitimate transactions (such as a credit card payment that was mistakenly refused), thus increasing customer satisfaction.
Machine learning and automation techniques get better and better at preventing cyber attacks of all kinds.
AI models in the banking domain are trained to reject suspicious transactions or flag them for further investigation. They can also predict the likelihood of fraud, allowing human investigators to focus their efforts on only a few fabricated transactional instances that require human intervention. Machine learning is used in behavioral analytics to analyze and predict behavior at a granular level across all aspects of a transaction.
JP Morgan Chase has set an excellent example of the applications AI has to offer.
The bank previously employed a team of lawyers and loan officers who used to spend 360,000 hours each year tackling mundane tasks and reviewing compliance agreements. But by using an ML-powered program, the bank was able to process 12,000 agreements in just a few seconds.
Industries that are extensively involved in e-commerce have transitioned from rule-based systems to machine learning-based models. AI-based fraud detection technologies can constantly adjust rules and even learn new ones as more and more data is processed.
Unfortunately, these benefits of AI in finance and accounting do not come without risks.
For example, when bank employees give biased advice based on AI recommendations, the entire institution may start systematizing bias into the decision-making process.
Looking for free datasets to kickstart your machine learning project. Check out 65+ Free Datasets offered by V7
Loan Decisions
AI-first banks and investment firms use extensive automation and near-real-time analysis of customer data to produce prompt loan decisions by analyzing loan risks using structured and unstructured data gathered from varied established sources.
AI in banking and finance has expanded to assess the creditworthiness of potential borrowers who do not have a credit history.
Recent studies show that financial institutions will slowly pave their way to expand the availability of affordable credit to so-called "credit invisibles" or "unscorables" by using alternative data such as:
Education
Job function
Property ownership
Address stability
Rent payment history
Internet browser history
Behavioral information
Leading lenders, like Ally, are also automating the process of approving the loan and predicting the maximum amount a customer may borrow and the pricing of the loan using AI and ML models.
Thanks to the development in natural language processing (NLP), AI systems swiftly determine a customer's disposable income and ability to make timely loan payments. For example, by using Optical Character Recognition (OCR), AI can extract and process data from bank accounts, tax returns, or utility invoices.
OCR can automatically recognize and extract data from scanned documents and images in a structured way and helps in reducing processing times for each document.
Process Automation
Automating financial processes relies on artificial intelligence’s ability to gain insights from existing data to optimize credit decisions, risk assessment, and auditing, among others.
Process automation is an interesting option for businesses looking to hire or outsource their financial processes, as well as for professionals who wish to streamline internal processes.
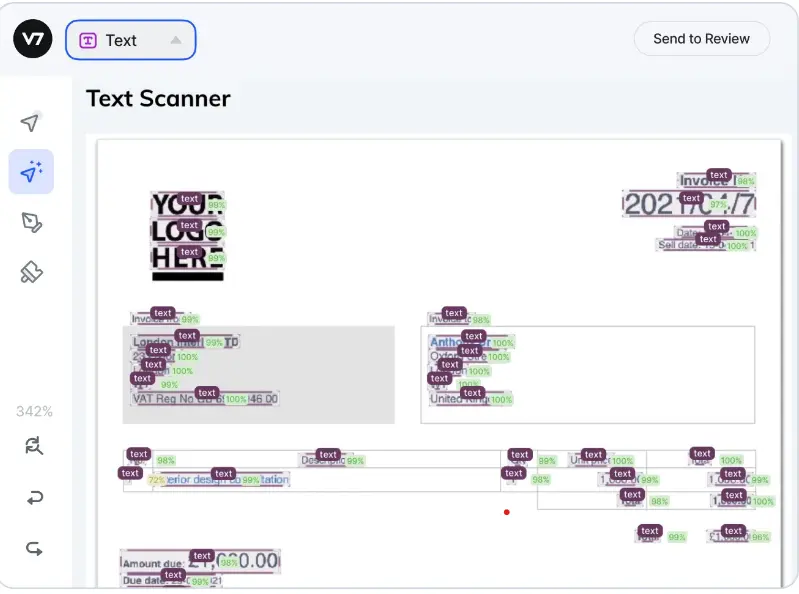
One of the techniques that comes in handy for automation is the already mentioned optical character recognition.
OCR was created by MIT researchers to quickly and accurately read and match the handwritten portions of checks, and effectively changned the perception of using AI in the banking industry.
Since then, OCR has made its way into enterprise resource planning (ERP) and customer relationship management (CRM), going far beyond check processing.
Other technologies are also being tested—
For example, voice-activated programs are used to save time searching for customer information in a database or through piles of documents. What’s more, some banks and investment firms are connecting their technology with Alexa, allowing their customers to check their account balance, make payments, place orders, or ask customer service for help.
Financial automation will undoubtedly affect the responsibilities of many staff members, so managers may have to re-engineer processes and redeploy resources to maximize productivity and output in more sophisticated and strategic areas.
All the major public cloud providers—Google, Amazon Web Services, and Microsoft Azure— equip machine learning platforms with user-friendly developer tools and libraries to scale and speed up the operations.
Algorithmic Trading
All technical analysis is based on statistical data, market behavior, and past correlations.
No wonder that artificial intelligence outperforms human intelligence in market pattern analysis, risk management, and general trading in the market with high volatility.
According to JPMorgan, in 2020 over 60% of trades of over $10M were executed using algorithms.
AI Autotrade is thriving, and it’s developing entirely autonomous trading machines that combine technical analysis with AI self-learning algorithms whose task is to manage deposits for profit. Recent studies show that machine learning algorithms already close approximately 80% of all trading operations on US exchanges.
Some of the benefits of algorithmic trading include:
Increased precision and reduction of human error
Better trading execution by eliminating delays when triggering payments
Automatic and simultaneous examination of multiple market conditions
Top financial institutions like Goldman Sachs have been using AI not only for price prediction but also for decision-making and risk management. The company has an underlying AI model trained on historical market data that predicts the price and underlying risks using a time-series forecasting model like Recurrent Neural Networks.
Do patterns in your data change over time? Does sequential information come into play—like in the case of forecasting stock prices? If so, check out our complete guide to Recurrent Neural Networks.
Financial Robo-Advisory
A robo-advisor is a personal financial management platform that has a background machine learning algorithm running unattended. The advisor trades on an investor’s behalf and manages their account using survey responses which human advisors usually run.
The robo-advisor tends to make investments to maximize returns within an acceptable level of risk through diversification. The general information that the robo-advisor needs includes age, investment timeline, and risk tolerance.
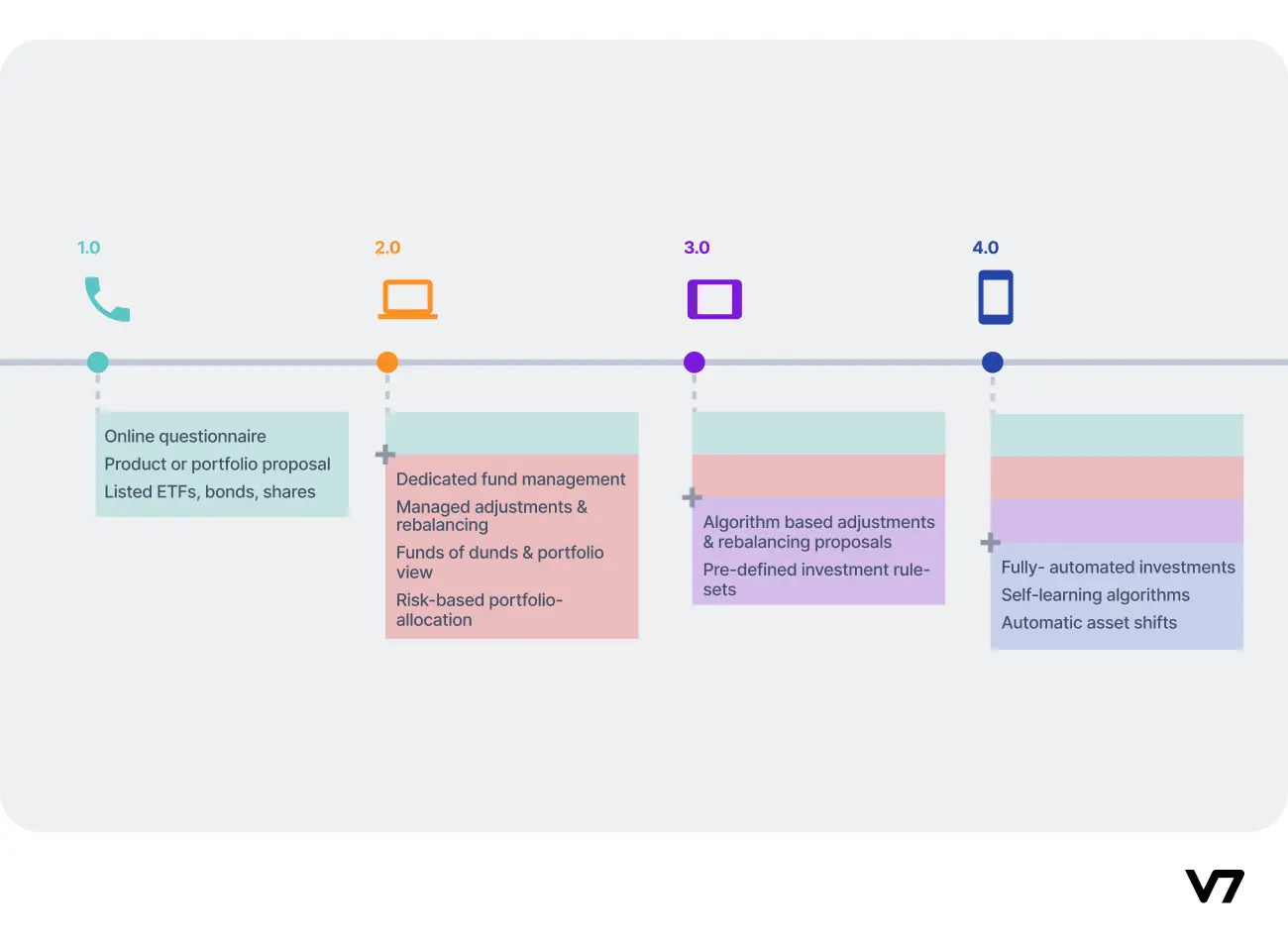
The investor only needs to take care of depositing the money each month, and everything else—from picking the assets to analyzing market conditions to purchasing the assets—is handled for them.
Using robo-advisory is more cost-effective than using a traditional advisor, provides opportunities that traditional analysis may otherwise overlook, and eliminates time-consuming tasks such as rebalancing and checking proper asset allocation.
A recent article from Deolitte introduces a UK-based robo-advisor, Wealthify, which is considered one of the fastest growing robo-advisors in the market today. It’s based on an in-house algorithm that recognizes and anticipates changes in market conditions and automatically proposes shifts in clients’ investment accounts, and sends a push notification to the client.
Enhanced Security in Transactions
Data security in the banking and finance sector is a critical issue as it concerns sensitive customer data.
With so much information publicly available and increased fraudulent activities, organizations are finding it increasingly challenging to keep their usernames, passwords, and security questions safe.
In fact, there may be a drift from passwords, usernames, and security questions in the coming years in favor of more seamless and accurate fraud prevention techniques.
Major FinTech companies are slowly moving away from storing data in traditional database like SQL towards using blockchain that provides better encrypted platform for storing sensitive information.
Machine learning applications in the finance sector are likely to take security to the next level through the use of voice and face recognition, as well as other biometric data.
This would naturally increase customer retention and satisfaction by instilling trust through a secure and seamless authentication process. Some of the companies that have heavily invested in security machine learning and are working extensively towards this shift include Adyen, Payoneer, Paypal, and Stripe.
On the more technical side, banking algorithms rely on NLP to monitor and analyze numerous emails and information from other sources that enter the banking systems. They look for patterns that suggest malicious behavior and scan for any leak of customer-trusted information using supervised machine learning algorithms like SVM, random forest trees, etc.
Data Bias and Quality
Artificial intelligence offers financial institutions a strategic opportunity provided they invest in data quality management.
AI in finance and banking offers exciting possibilities for improving data quality as well as for mining more insightful information.
AI and machine learning techniques can be used for:
Identifying inconsistencies in the client data (for example, discrepancies between the provided addresses and countries)
Improving the quality of data
Flagging any suspicious/erroneous client data
Predicting missing values in transaction data
One of the major risks that come with the applications of AI in banking and finance is the presence of “programmed bias” in the machine learning algorithms used by FinTech companies.
Bias from the baseline data is only one of the ways it can creep into AI and fintech activities. For instance, a publicly available dataset on US FSPs highlighted in this paper indicates that close to 20% of the adult population receive insufficient credit services. An inference derived from this data reveals that women-owned enterprises receive a disproportionately low share of accessible credit, attract smaller loans, and attract harsher penalties for defaulting.
Many open-source toolkits such as IBM AI Fairness 360, Aequitas, and Google What-if assist fintech companies in measuring discrimination in AI models. They recommend mitigation pathways to eliminate bias from data pipeline, and test the overall impact of the biased data on real-world scenarios.
These AI-enabled toolkits look for outliers that demonstrate data bias and remove them from the data flow. It’s also helpful to generate synthetic data by analysing clustered data points to increase the efficiency of the models involved.
To learn more about the importance of data quality, read our introductory guide to quality training data for machine learning.
Challenges of Using AI in Finance
So far, we’ve seen a number of innovative applications of AI in the finance industry. Still, it’s equally vital to understand the potential challenges associated with using AI and machine learning in this industry.
Data Issues
In the world of data science, there is a saying that goes "garbage in, garbage out."
In practice this means that several isolated instances of incorrect or biased data supplied into a trading algorithm can have catastrophic effects on the entire system and result in losing trades and money.
There must be a mechanism to instantly locate anomalies throughout the entire pipeline, pinpoint the problem, and resolve it. That’s exactly why some businesses are built around this idea and offer git-like version control for even their own data.
Another challenge that comes with data is localization. Global financial institutions often need to design models across the multiple market areas they serve. The data must be consistent across different languages, cultures, and demographics to properly customize the customer experience.
Dimensionality reduction
A single transaction can consist of hundreds of data points, which is why financial firms are considered to be sitting on data troves.
For this reason, the entire banking and finance sector has a very low signal-to-noise ratio, which makes the work of data scientists both tough and fascinating.
However, when the number of characteristics skyrockets, many machine learning approaches start to struggle. In that case, the analysts must either carry out some kind of feature selection or attempt to minimize the data's dimensionality. They can employ well-known methods like Principal Components Analysis (PCA) and Linear Discriminant Analysis for the latter (LDA).
Black box
The financial industry is heavily regulated and customer-centric, and all the algorithmic decisions must be fully understood and approved by the institution.
For example, let's consider a person who has a low credit score and has their loan application denied. The individual could then file a claim and request a detailed explanation of all the factors that led to the rejection.
That’s why model explainability is crucial in the financial industry. While the latest state-of-art neural network architecture may be appealing and provide better accuracy, it’s rarely the best tool for the job due to its complex nature. Instead a simpler model is chosen which is better explainable to the layperson.
What are neural networks and how do they work? Have a look at essential guide to neural network architectures.
Security and Compliance
One of the main challenges of AI in financial services is the amount of data collected from the customers, which contains sensitive and confidential information like transaction history, account information, or loan details.
This calls for additional security measures to be in place. The right data partner will provide a range of security options, strong data protection through certifications and regulations, and security standards to ensure the customer data is handled appropriately.
Key Takeaways
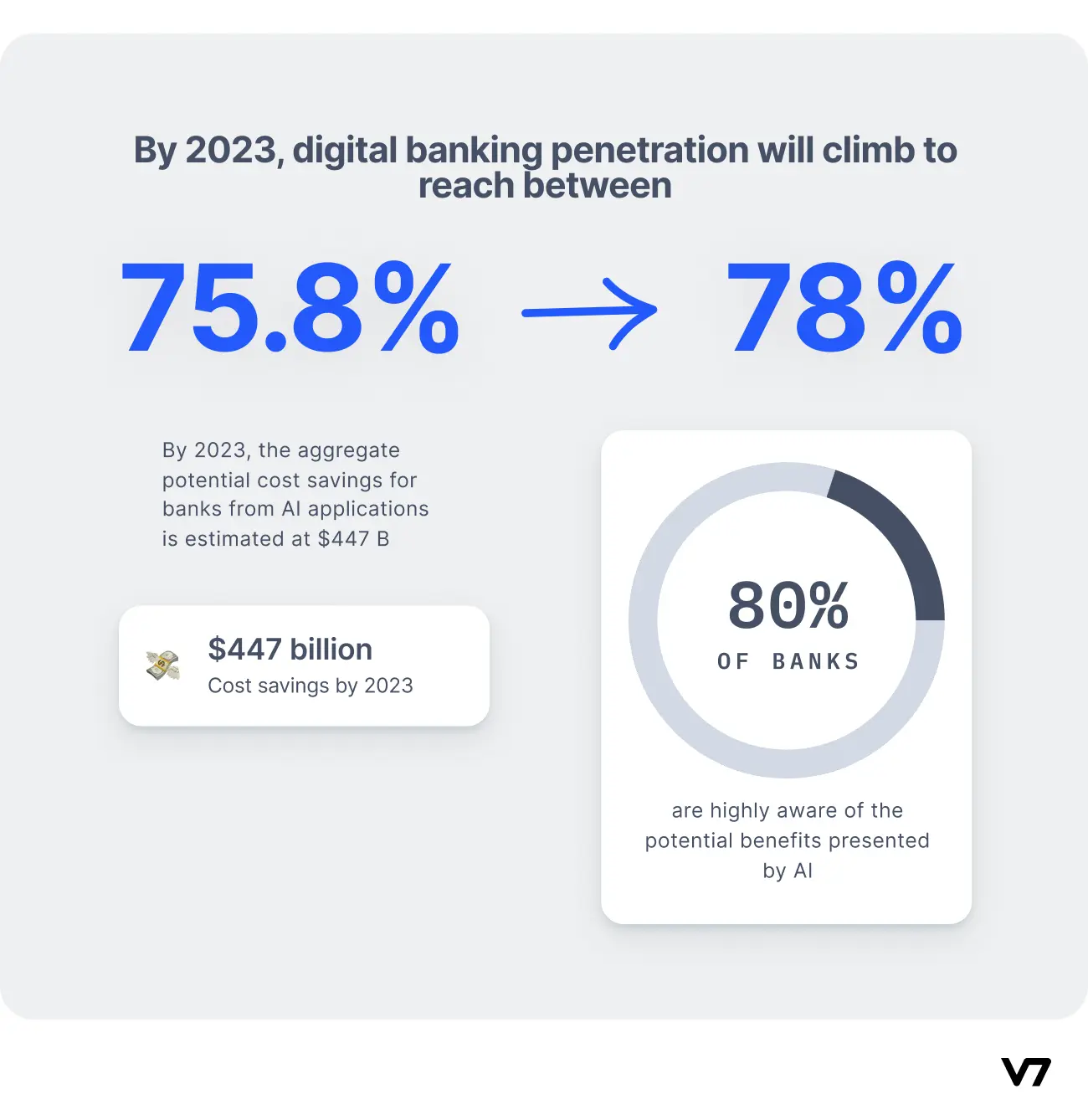
The finance industry has always seen the potential benefits of implementing AI-based solutions. But with the widespread impact of COVID-19, AI has become more of a necessity rather than an option. Most people have embraced the digital experience, and the paradigm shift from traditional banking channels to virtual AI-based services is now more critical than ever.
The areas where the impact of AI in finance is expected to increase in the future include:
Customer acquisition by offering personalized banking experiences
Fully automated services, such as risk assessment
Fighting off cyberattacks
Protecting valuable data from hackers
Making investment decisions based on customer preferences
AI is expected to serve as a vehicle for customer-centric services in the finance industry.
If you’d like to explore AI applications in other industries, have a look at these articles:
Pragati is a software developer at Microsoft, and a deep learning enthusiast. She writes about the fundamental mathematics behind deep neural networks.