AI implementation
AI in Drug Discovery: 10 Cutting-Edge Applications
17 min read
—
May 29, 2023
Let’s explore the use of AI in drug discovery, including its applications, benefits, challenges, and the future of the industry.
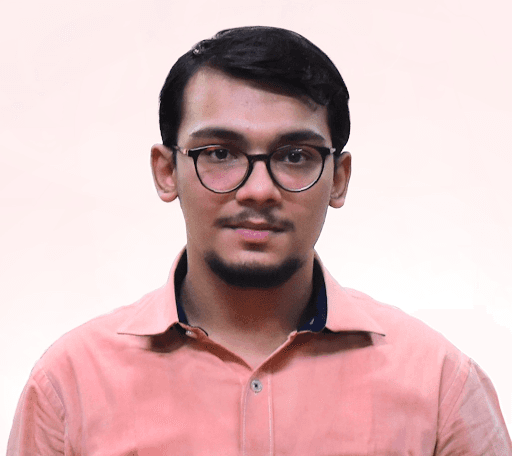
Guest Author
In recent years, Artificial Intelligence has emerged as a key player in developing innovative treatments for various diseases. According to Forbes, pharmaceutical companies can save nearly 70% of their costs by using AI for drug discovery.
The drug discovery process is typically time-consuming and labor-intensive. It spans many steps, from target identification to clinical trials, due to the complexity of the human immune system.
AI has the potential to streamline the drug discovery process, reduce the time and costs involved, and lead to the development of more effective and efficient treatments.
In this blog, we will explore the use of AI in drug discovery, including several of its applications, benefits, and challenges. Whether you are a researcher, a healthcare professional, or simply interested in the role of AI in medicine, this blog will provide an in-depth look at the exciting and rapidly-evolving field of AI in drug discovery.
We’ll cover the following ten applications of AI in drug discovery:
Faster drug development
More effective drugs
Better clinical trial design
Prediction of drugs’ bioactivity
AI in quality assurance
Drug repurposing
Drug combination analysis
Patient stratification
Real-World Evidence
Post-market safety monitoring
Ready? Let’s go!
AI in drug discovery: Overview
The traditional drug discovery process is educated guesswork in the beginning. It is a long (up to 15 years), complex (takes the effort of several experts), and expensive (billions of dollars) process to complete. It involves several stages, each with high rates of failures:
Target identification: The objective of this stage is to identify a biological target that is involved in the disease process. Traditionally, this process involves a combination of biochemical assays, cell-based assays, and animal studies.
Lead discovery: This involves identifying a molecule that has the potential to interact with the target and modulate its activity. The traditional approach involves screening large compound libraries to identify active molecules, which is time-consuming.
Lead optimization: It is the process of optimizing the pharmacological properties of a lead molecule, such as its potency, selectivity, and bioavailability. This process typically involves medicinal chemistry, computational modeling, and in vitro testing.
Preclinical testing: It involves testing the efficacy and safety of the lead molecules in animal models. This process can be time-consuming and expensive.
Clinical trials: Clinical trials involve testing the safety and efficacy of lead molecules in humans. This is the most expensive and time-consuming stage of the drug discovery process, as it involves extensive clinical trials to assess the safety and efficacy of the drug in humans.
AI can significantly improve the drug discovery process by reducing costs, and time and increasing the chances of success at each stage, which we will look at in the following sections of the article.
Faster drug development
With the advent of AI, the target identification process can be accelerated by leveraging machine learning algorithms to analyze large data sets. For example, a machine learning algorithm can help analyze the large-scale genomic data of a disease, identify potential drug targets, and predict the drug's effectiveness. Machine learning algorithms can also analyze scientific literature to identify potential drug targets and assist in identifying genetic biomarkers for disease diagnosis and prognosis.
AI can predict the binding affinity of potential molecules to the target protein, thereby reducing the number of compounds that need to be screened experimentally. AI can also analyze the structure of the target protein and generate virtual libraries of molecules with similar structural features to known inhibitors, which can be used to guide the design of new molecules. All this will significantly accelerate the lead discovery procedure.
AI can also be used to analyze large datasets from existing preclinical studies, such as gene expression data, to predict the efficacy and safety of the lead molecules, thus reducing the time for preclinical trials.
Pro tip: Check out how V7 can aid your life science & biotech AI project—from multispectral and hyperspectral image annotation to model training and deployment.
More effective drugs
AI can significantly improve the lead optimization process by predicting the pharmacological properties of the lead molecules based on their chemical structure. Machine learning algorithms can be used to build predictive models that can estimate the properties of the lead molecules, such as solubility, bioavailability, and toxicity. These predictive models can then guide the design of new molecules with improved pharmacological properties.
AI can help to reduce the number of animal studies required by predicting the efficacy and safety of the lead molecules in-silico. For example, AI algorithms can predict the toxicity of lead molecules based on their chemical structure, reducing the number of compounds that need to be tested experimentally.
AI can also be used to design more selective drugs with fewer side effects. For example, researchers can use AI to identify specific proteins or molecular pathways involved in disease and then design drugs that selectively target these proteins or pathways. This can help reduce the likelihood of off-target effects and improve the safety and efficacy of drugs.
Pro tip: Check how V7 can support medical imaging AI teams
Better clinical trial design
AI can play a significant role in designing better clinical trials by improving the efficiency and accuracy of various aspects of the process. Below are some examples of how AI can aid in designing better clinical trials:
Patient recruitment: One of the biggest challenges in clinical trials is patient recruitment. AI can help improve the efficiency of patient recruitment by analyzing electronic medical records (EMRs) and other sources of patient data to identify potential candidates that meet the inclusion and exclusion criteria of the trial. This can reduce the time and costs associated with patient recruitment and improve the chances of successful trial completion.
Trial design: AI can also aid clinical trial design by providing insights into optimal trial designs. For instance, AI algorithms can analyze patient data to identify subgroups of patients more likely to respond to a particular treatment, enabling researchers to design more targeted trials with a higher chance of success. AI can also help optimize the trial's ample size and statistical power, ensuring that the trial has sufficient statistical power to detect meaningful differences in treatment outcomes.
Real-time monitoring: AI can also help monitor patients in real time during the clinical trial. For instance, wearable devices equipped with AI algorithms can track patient vital signs and other health metrics, providing real-time data that can be used to adjust the trial protocols and improve patient safety. AI algorithms can also analyze patient data in real-time to detect adverse events and other safety issues, enabling researchers to take prompt action to address these issues.
Data analysis: AI can also aid in analyzing clinical trial data. AI algorithms can analyze large volumes of data generated during the trial to identify trends and patterns that human analysts may miss. This can help researchers identify new insights and hypotheses that inform future research and clinical practice.
Prediction of drugs’ bioactivity
AI can aid in predicting drugs' bioactivity by leveraging machine learning algorithms to analyze vast amounts of data on known compounds and their biological activities. These algorithms can learn from this data to predict the bioactivity of new, untested compounds.
One approach to predicting bioactivity is through quantitative structure-activity relationship (QSAR) modeling. QSAR models use mathematical algorithms to analyze the chemical structure of a compound and predict its biological activity based on known data. These models can predict the bioactivity of a compound based on its molecular properties, such as molecular weight, the presence of functional groups, and other structural features.
Another approach to predicting bioactivity is molecular docking. Molecular docking is a computational technique that predicts the binding affinity of a small molecule to a target protein. This technique involves simulating the binding of a small molecule to the protein and evaluating the resulting binding energy. The lower the binding energy, the stronger the interaction between the molecule and the protein, and the higher the predicted bioactivity of the compound.
AI algorithms can also use deep learning techniques to analyze large datasets of compounds and their biological activities. These algorithms can identify patterns and relationships between the chemical structure of the compounds and their biological activity and use these patterns to predict the bioactivity of new, untested compounds.
In addition to predicting bioactivity, AI can also help optimize the chemical structure of compounds to improve their bioactivity. This is known as structure-based drug design. AI algorithms can analyze the structure of a target protein and use this information to design compounds optimized for binding to the protein.
AI in quality assurance
AI can be used in drug quality assurance to improve the accuracy and efficiency of various aspects of the quality control process.
Quality control inspection: AI can help automate the inspection of drugs to ensure that they meet quality standards. For instance, computer vision algorithms can analyze images of drug samples to detect defects such as discoloration, contamination, or improper packaging. This can reduce the time and costs associated with manual inspection and improve the accuracy and consistency of the inspection process.
Predictive maintenance: AI can also aid in the predictive maintenance of manufacturing equipment. By analyzing sensor data from manufacturing equipment, AI algorithms can predict when equipment will likely fail, enabling proactive maintenance and reducing downtime. This can improve the efficiency and reliability of the manufacturing process and reduce the risk of defects and errors in the production of drugs.
Read more: 7 Out-of-the-Box Applications of AI in Manufacturing
Fraud detection: AI can also aid in detecting fraud in the production and distribution of drugs. For instance, AI algorithms can analyze sales and distribution data to detect unusual patterns or trends that may indicate fraudulent activity. This can help to identify and prevent the distribution of counterfeit or substandard drugs, improving patient safety.
Risk management: AI can also aid in risk management by identifying potential risks and hazards in the production and distribution of drugs. For instance, AI algorithms can analyze environmental and supply chain logistics data to identify potential drug quality and safety risks. This can enable proactive measures to mitigate these risks and prevent quality issues.
Drug repurposing
AI can aid in drug repurposing, which is identifying new therapeutic uses for existing drugs. Drug repurposing can significantly reduce the time and costs associated with drug development, as existing drugs have already undergone clinical trials and have established safety profiles.
One approach to drug repurposing is computational drug repositioning. This involves analyzing large datasets of drug and disease information to identify potential new uses for existing drugs. AI algorithms can be used to identify patterns and relationships between drugs and diseases and use these patterns to predict which existing drugs may be effective for treating new diseases.
Another approach to drug repurposing is through network pharmacology. This involves analyzing the complex interactions between drugs, targets, and diseases to identify new therapeutic opportunities. AI algorithms can be used to analyze large networks of molecular interactions and identify potential new drug targets or combinations that may be effective for treating different diseases.
AI can also aid in drug repurposing by predicting the side effects of existing drugs. By analyzing large datasets of drug and patient data, AI algorithms can identify potential side effects of existing drugs that may have therapeutic value for other diseases. For example, an existing drug used to treat a particular condition may have side effects that could be useful in treating a different disease.
Additionally, AI can aid in discovering new drug combinations for repurposing. This involves analyzing large drug and patient data datasets to identify potential drug combinations that may be effective for treating different diseases. AI algorithms can be used to predict the synergistic effects of different drug combinations and identify which combinations may have the most significant therapeutic potential.
Drug combination analysis
Many diseases, such as cancer, are highly complex and challenging to treat with single drugs. Drug combinations can offer a more effective approach by targeting multiple pathways involved in the disease.
AI can aid in drug combination analysis, which is the process of identifying and optimizing drug combinations to treat complex diseases.
AI can help in drug combination analysis by analyzing large drug and patient data datasets to identify potential drug combinations and predict their efficacy.
Below are some ways in which AI can be used in drug combination analysis:
Predicting synergistic effects: AI algorithms can predict the synergistic effects of different drug combinations. This can help to identify drug combinations that may be more effective than single drugs in treating complex diseases.
Identifying optimal dosing: AI algorithms can also aid in identifying the optimal dosing for different drug combinations. This involves analyzing the interactions between different drugs and predicting the optimal dosage for each drug in the combination to achieve the desired therapeutic effect.
Personalized medicine: AI can also help to personalize drug combinations for individual patients based on their genetic and molecular characteristics. This involves analyzing large datasets of patient data to identify biomarkers that may predict the patient's response to different drug combinations.
Drug resistance: AI algorithms can also help to identify drug-resistant pathways and suggest alternative drug combinations that may be more effective. AI can predict which drug combinations may be more effective in patients who have developed resistance to certain drugs.
Pro tip: Check out how to streamline digital pathology AI development with V7
Patient stratification
Patient stratification refers to the process of identifying subgroups of patients who share similar characteristics or disease profiles. This is important in drug development and personalized medicine since patients may respond differently to the same treatment. Identifying patient subgroups can help healthcare providers to personalize treatment and improve outcomes.
AI can aid in patient stratification by analyzing large datasets of patient data and identifying patterns and associations that may not be apparent through traditional analysis methods.
Below are some ways in which AI can be used in patient stratification:
Predictive modeling: AI algorithms can analyze large datasets of patient data and develop predictive models to identify patient subgroups who may be at higher risk for certain diseases or respond differently to specific treatments. This can help healthcare providers to personalize treatment and improve outcomes for different patient subgroups.
Biomarker identification: AI can aid in identifying biomarkers that may predict a patient's response to a drug or disease progression. AI can identify genetic, molecular, or imaging biomarkers associated with specific disease subtypes or treatment responses.
Clinical trial design: AI can also aid in designing clinical trials by identifying patient subgroups who may be more likely to respond to a particular treatment. AI can help to design more efficient and effective clinical trials and improve the success rate of drug development.
Personalized medicine: AI can help to personalize treatment by identifying patient subgroups who may respond differently to a particular treatment. AI can help healthcare providers to tailor treatment to individual patients based on their genetic, molecular, or imaging characteristics.
Real-World Evidence
Real-world Evidence (RWE) refers to data collected from real-world sources, such as electronic health records, claims databases, and patient-generated data. RWE can provide valuable insights into the safety and effectiveness of drugs in real-world settings outside of the controlled environment of clinical trials.
However, it takes human experts a lot of time. AI can aid in interpreting RWE to identify patterns, trends, and associations that may not be apparent through traditional analysis methods.
Below are some ways in which AI can be used in the analysis of RWE:
Predictive modeling: AI algorithms can analyze large datasets of RWE and develop predictive models to identify patients at risk for adverse events associated with a drug. This can help healthcare providers to monitor patients more closely and intervene before serious adverse events occur.
Data integration: AI can aid in integrating disparate data sources, such as electronic health records, claims databases, and patient-generated data, to provide a more comprehensive view of patient outcomes. This can help identify trends and associations that may not be apparent through analyzing individual datasets.
Precision medicine: AI can help to identify subgroups of patients who may respond differently to a drug based on their genetic and molecular characteristics. By analyzing large datasets of RWE and patient data, AI can identify biomarkers that may predict a patient's response to a drug and help healthcare providers to personalize treatment.
Comparative effectiveness: AI can also aid in comparing different drugs and treatment strategies based on real-world outcomes. AI can identify which drugs and treatments are most effective for different patient populations and help healthcare providers to make more informed treatment decisions.
Post-market safety monitoring
AI can help in post-market drug safety monitoring, which is the process of monitoring the safety of drugs after they have been approved and used by patients. This is a critical aspect of drug development, as rare or unexpected side effects may not be detected during clinical trials and may only be identified after the drug is on the market.
Below are some ways in which AI can be used in post-market drug safety monitoring:
Signal detection: AI algorithms can analyze large datasets of patient data and identify signals that may indicate potential adverse events associated with a drug. This can help to detect rare or unexpected side effects that may not have been detected during clinical trials.
Real-time monitoring: AI can provide real-time drug safety monitoring by analyzing data from electronic health records, social media, and other sources. This helps identify potential safety issues as they arise, allowing for faster and more effective interventions.
Risk prediction: AI algorithms can also aid in predicting the risk of adverse events associated with a drug based on patient characteristics and other factors. This can help to identify patients who may be at higher risk of experiencing side effects and allow for more targeted interventions.
Drug-drug interactions: AI can identify patterns and relationships between different drugs and predict which combinations may be more likely to cause side effects.
Challenges of adopting AI in drug discovery
While AI has shown great promise in aiding drug discovery, widespread adoption is still hindered by several crucial challenges.
Data quality and quantity: AI relies on high-quality data to generate accurate predictions and models. However, data in drug discovery is often sparse, heterogeneous, and of varying quality, making it difficult for AI to accurately analyze and model the data.
Data privacy and regulatory compliance: Data privacy and regulatory compliance are major concerns when working with sensitive patient data. The use of AI in drug discovery requires compliance with data protection and privacy regulations, such as HIPAA in the US and the EU's General Data Protection Regulation (GDPR).
Lack of standardization: There needs to be more standardization in data formats, data collection, and data analysis methods in drug discovery. This can make it difficult to compare results across different studies and datasets, limiting the effectiveness of AI in generating accurate predictions and models.
Interpretability and transparency: AI models can be difficult to interpret, making it challenging to understand how they generate their predictions and recommendations. This lack of transparency can lead to mistrust and limit the adoption of AI in drug discovery.
Cost and technical expertise: Implementing AI in drug discovery requires a significant investment in financial and technical resources, including developing and maintaining infrastructure and hiring skilled data scientists and AI experts.
Validation and integration: AI models need to be validated and integrated into the drug discovery process in a reliable, scalable, and efficient way. This requires careful planning and collaboration between stakeholders, including data scientists, biologists, chemists, and regulatory authorities.
Final thoughts
Artificial intelligence has become a game-changer in various industries, drug discovery being no exception. AI has the potential to revolutionize the drug discovery process and offer new opportunities to develop innovative therapies. The ability of AI to handle vast amounts of data and perform complex analyses quickly and accurately makes it an indispensable tool in the modern drug discovery landscape.
However, some challenges must be addressed for AI to reach its full potential in drug discovery. The availability of high-quality data is a critical challenge, as is the complexity of the drug discovery process. Regulatory and ethical considerations must be addressed, such as the approval of AI-based drugs by regulatory bodies and the potential impact of AI-based drugs on patients. Researchers are making continual progress toward addressing these shortcomings, and AI’s role in drug discovery will grow in the coming years.
More resources on artificial intelligence across different industries: