AI implementation
Build Vs. Buy? Tackling Training Data Ops Software
10 min read
—
Aug 29, 2023
Choosing a training data software is a high-stakes decision with many potential pitfalls. This guide will walk you through the selection process of picking the right platform for your use case.
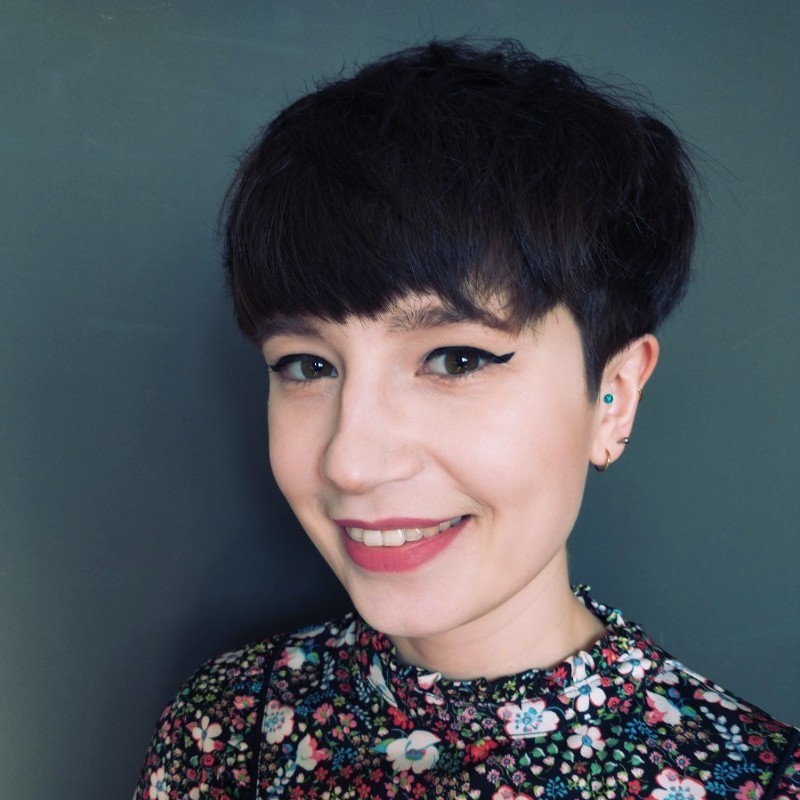
Content Specialist
To guarantee the level of scalability and quality assurance necessary for high-quality products, you need ironclad AIOps software.
However, assembling fit-for-purpose training data infrastructure is easier said than done.
Inevitably, all AI product leaders face an uphill challenge to fuel the quality, speed, and cost-effectiveness of their training data processes. Worse yet, infrastructural decisions must be made quickly—as the pace of the current AI hype cycle has sent many companies on the hunt for success.
To cope with this challenge, many machine learning teams find themselves at the center of the build vs. buy dilemma—as they grapple with either developing dedicated software that meets their needs or investing in a ready-to-go training data platform.
With the vast majority of Fortune 100 companies having moved their operations into third-party platforms, the answer may seem obvious—however, with the life of an AI product at stake, it’s best to carefully examine your options before making the final call.
In this guide, we’ll help you to decide the best approach to training data infrastructure for you and your team.
We’ll go through the pros and cons of both options and go through the ten most important points you must consider when deciding whether to build or buy your training data operations.
Let’s go!
Why do companies decide to buy or build their MLOps tools?
Setting up complex data pipelines demands reliable tooling. A few years ago, many machine learning teams were forced to develop their own in-house solutions, unable to find software that could satisfy their specific data needs.
Fast forward to 2023, and we have a vast landscape of mature training data ops platforms, ready to cater to all sorts of specialized use cases. It’s become possible to build MLOps pipelines with exclusively third-party solutions—relieving data engineers, cutting development time, and minimizing costs in the long term.
Many of the solutions on the market today are so competitive that even companies that already built in-house tools have made the change to external vendors. For many, the growing amount of resources—both financial and man hours—required for upgrades and maintenance turns out to be a bigger strain on the bottom line than making the switch.
Choosing to build or buy your MLOps infrastructure boils down to the number of core pros and cons relevant to your business.
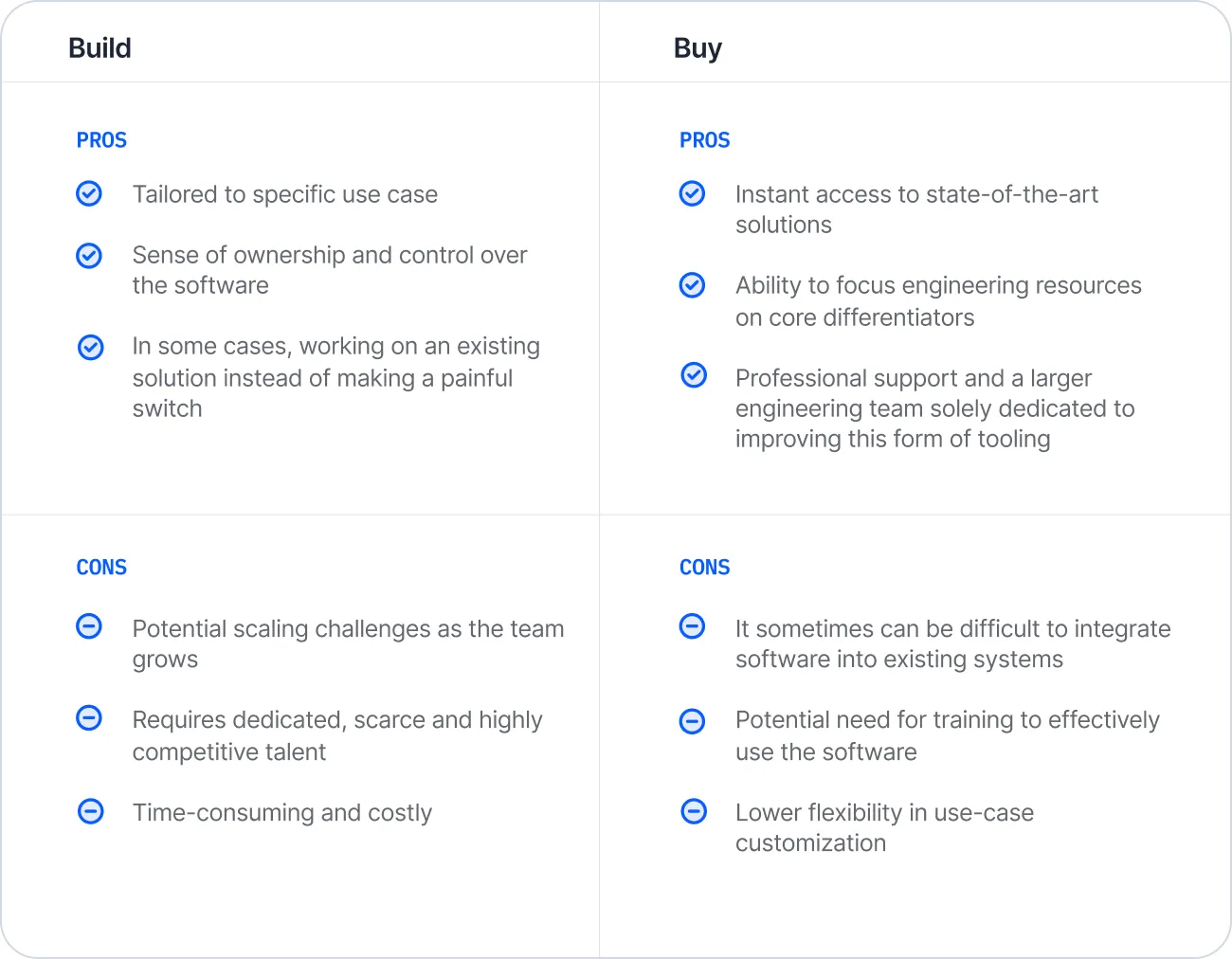
However, before making the final decision, it's essential to closely interrogate your priorities - and whether your solution tackles them. Here are the ten most important factors to consider when deciding whether you should build or buy your training data ops:
Data security: Will your data be kept secure?
Hosting: Should you choose cloud or on-prem implementation?
Effectiveness: How does the software stack up against your current solution?
State-of-the-art technology: What are the latest technologies available in training data management?
Customization: Will the software fit your unique use case?
Integration: Will the software integrate with your existing technology stack?
Compatibility: Should you consider using your cloud provider's default proprietary software?
Cost: What is the total cost, and how do you calculate it?
Expertise: Will you get help and assistance from experts in the field?
Value: Is it worth investing in a training data platform?
To help you make this choice, let’s take a look at each of these points in more detail.
1. Data security
Many companies opt for internal solutions to ensure their data storage aligns with security standards. Historically, this has been the case for many companies dealing with sensitive information, for example, those in the healthcare or life sciences sectors.
However, cloud solutions have made it possible to integrate with external services while storing data internally—ensuring businesses can leverage state-of-the-art MLOps solutions while preserving the security of their data
V7 offers both cloud and object storage integrations. The platform allows users to store data wherever they prefer, while still visualizing it within V7's interface. This approach is in line with HIPAA and FDA guidance, ensuring regulated industries can develop AI with confidence.
Learn more: Accelerating AI Product Development in Digital Pathology with Advanced Workflows
2. Hosting
In the past, companies preferred to keep their technology on-premises—however, the popularity of the cloud-first approach is on the rise. This is particularly true for MLOps, where deployed models “close the loop” with their training data in the cloud for continual learning.
One of the primary advantages of cloud-based training data operations software is the ease with which data can be shared between different stakeholders and collected from various sources, which increases work efficiency and transparency.
Cloud-based solutions also save companies the hassle of worrying about infrastructure when they want to scale up or down, boosting enterprise-wide flexibility.
Additionally, third-party platforms make it easier to create one controlled “data hub” within an organization. Process standardization supports consistent and controlled product development, reducing inefficiencies caused by incompatible asset production and information silos.
What’s more, external hosting reduces the risk of data loss—with maintenance in the hands of external machine learning professionals dedicated to monitoring and introducing the most relevant solutions.
3. Effectiveness
While an in-house tool can have its benefits, the key marker of a successful solution is its ability to deliver better AI products overall.
You need to ensure your platform boasts industry-leading accuracy, performant models and infrastructure designed to fuel impactful development.
To probe whether your in-house solution achieves this, we recommend running tests on internal and external solutions based on a few measurable, quantitative metrics, such as:
Accuracy versus a defined gold standard set
Speed of annotation
Time taken from requesting new data to receiving labeled data
Model accuracy
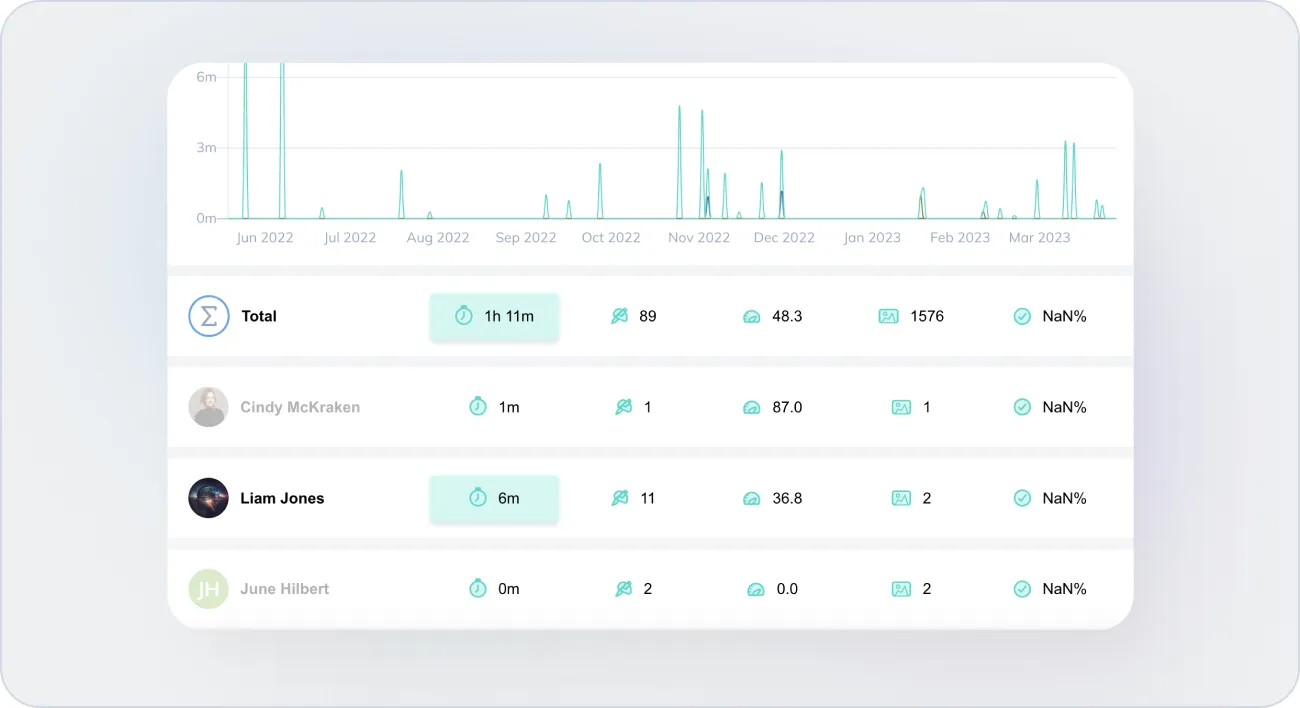
These test results will help you assess the efficiency based on hard data.
Read more: Top Performance Metrics in Machine Learning
4. State-of-the-art technology
Keeping up with the latest advancements in training data preparation directly impacts your ability to stay ahead of the competition and deliver superior products. With how rapidly the market changes, one slip-up can quickly impact your ability to stay at the forefront of your industry.
When it comes to state-of-the-art advancements in AI, here are a few trends that are currently gaining traction:
Integration of Large Language Models (LLMs) with computer vision models
Customizable, easily changeable workflows
Advanced analytics (including acceptable disagreement thresholds)
These rapid evolutions in the technological landscape often make a case for third-party software—designed to stay at the cutting edge of AI. In contrast, constantly introducing new technologies to an in-house tool can be costly and time-consuming, draining already precious resources that could be reserved for development. Worse yet, there are no guarantees that by the time you’ve caught up, your solution won’t have become obsolete.
"On V7’s Customer Success Team, we have the benefit of always being on the other end of the “buy” side of the build-or-buy decision. Computer Vision Teams often tell us that they made the decision to go with V7 over a custom solution for two main reasons: feature richness and the cost of maintaining a custom solution.
I think of Meta’s SAM release as a great case study for the “buy” decision. As soon as SAM was announced, we rallied V7’s Product and Deep Learning Teams to build a user-friendly integration. Our CS Team worked closely with V7’s customers to determine how SAM could be incorporated into their training data pipelines.
For our customers, this meant that engineering cycle time that would have been devoted to experimenting with SAM was freed up for other high-impact projects. We were able to focus on staying ahead of the curve so our customers can do the same."
said James Hudson, Principal Customer Success Manager at V7.
Learn more: What's Next for Large Language Models? [Webinar replay]
5. Customization
Having a platform perfectly fine-tuned to your use case is crucial to guarantee the best possible outcome from your training data process. However, building case-specific solutions from scratch can be extremely time and resource-intensive.
With how robust and competitive the training data platform market has become, most third-party solutions cover 95% of use cases. However, if you have unique features or customization options that your company requires, it's essential to ask potential vendors about their ability to accommodate those specific needs.
Evaluate these platforms based on your specific needs and ensure the investment guarantees a return. Take advantage of demos and free trials to see how a given tool can support all the tasks necessary for your use case.
Learn more: Book a product demo with V7’s team
6. Integrations
Integration is key when it comes to your MLOps platform—without it, you deprive your development of a powerful AI ecosystem, crucial to your product’s success. Similarly, your platform of choice should easily integrate with your tech stack—to maximize the value of existing tech commitments and minimize any potential downtime when making the switch.
Most modern MLOps platforms are designed to be integration-friendly, but it’s important to check if they fit your specific infrastructure.
Additionally, you need to pay attention to flexibility in API options. Many platforms will offer a REST API, and many will also have SDKs (such as a dedicated Python SDK). Ensure that you can easily connect with your cloud provider.
7. Compatibility
It may seem like an easier route to go with your cloud provider’s default proprietary software to ensure maximum compatibility.
However, it’s often a short-term solution. Many cloud providers build ML tools that let their customers get an easy start with their platform and fully expect them to outgrow them—often referring them to third-party vendors as their needs grow.
The training data management space is highly specialized and requires specific features and capabilities. If you need to go beyond basic labeling requirements, it’s better to seek out more specialized solutions from the get-go.
8. Expertise
On the one hand, building your tool gives you full control over the infrastructure of your project and constant access to people who are experts in maintaining it.
On the other hand, your team will become fully responsible for maintenance and addressing issues, which is extremely time-consuming. Additionally, you’ll be forced to keep up with industry trends and standards as they unfold.
In stark contrast, external providers will equip you with a dedicated support team (comprising both software and industry experts), as well as a wealth of educational materials and other resources.
9. Cost
Now, the most crucial point of all:
The true cost of both approaches.
Naturally, the cost cannot be assessed based on face value only—there’s more hidden under the hood of any solution.
You should carefully weigh the hard costs against the opportunity costs. Minimizing spending may seem tempting at first, but it may also diminish your product’s chance of success by limiting its quality and innovative edge, resulting in a delayed time-to-market.
To properly assess the ROI, compare the cost of a SaaS subscription to building and maintaining an internal tool across the following aspects:
Initial costs (subscription vs. development)
Potential gains in their core product's abilities
Ability to generate new areas of R&D and new product lines
Time-to-value
Cost of generating training data
Tool maintenance and update cost
Staffing requirements
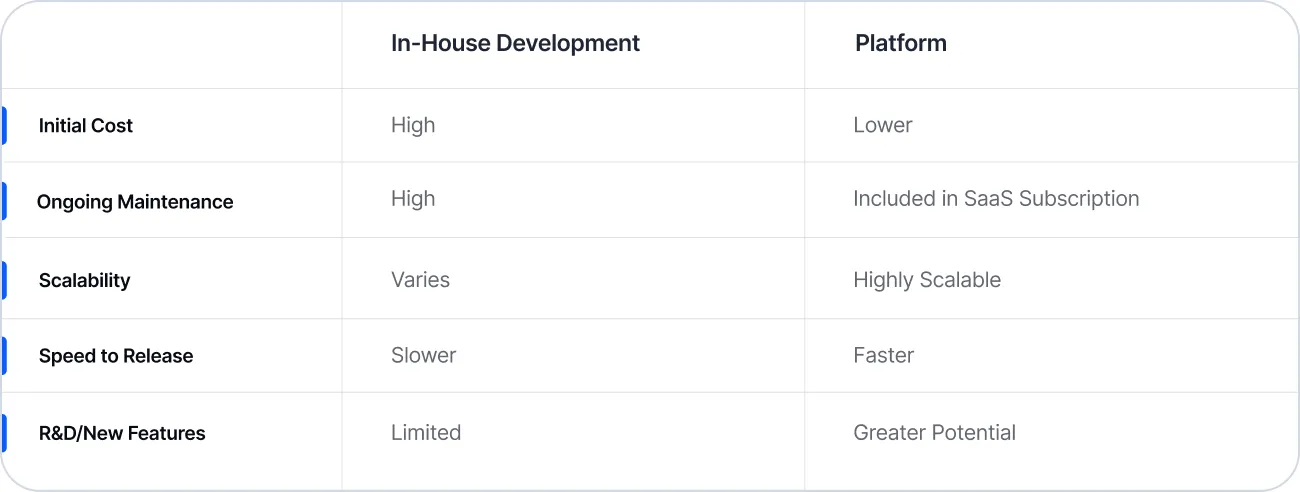
Learn more: Is Now the Right Time for You to Invest in Training Data Software?
10. Value
Now we come to the final question:
Will investing in a training data platform bring extra value to my product?
While this is a question only you can answer, current market trends overwhelmingly point towards the successes of the “buy” approach. Third-party tools have evolved to cater to the most specialized use cases. They also easily integrate with existing infrastructure and guarantee full data security.
At the price of the subscription, you can unlock access to dedicated teams of experts and state-of-the-art technologies that would otherwise be extremely costly to introduce in-house. In addition, MLOps platforms promote standardized processes, which further increase your efficiency, boost product quality, and reduce resource waste.
Still, consult your annotation, engineering, and data operations teams, as well as executive stakeholders, to discover their exact needs and milestones.
And to help you make the final call, here’s a checklist:
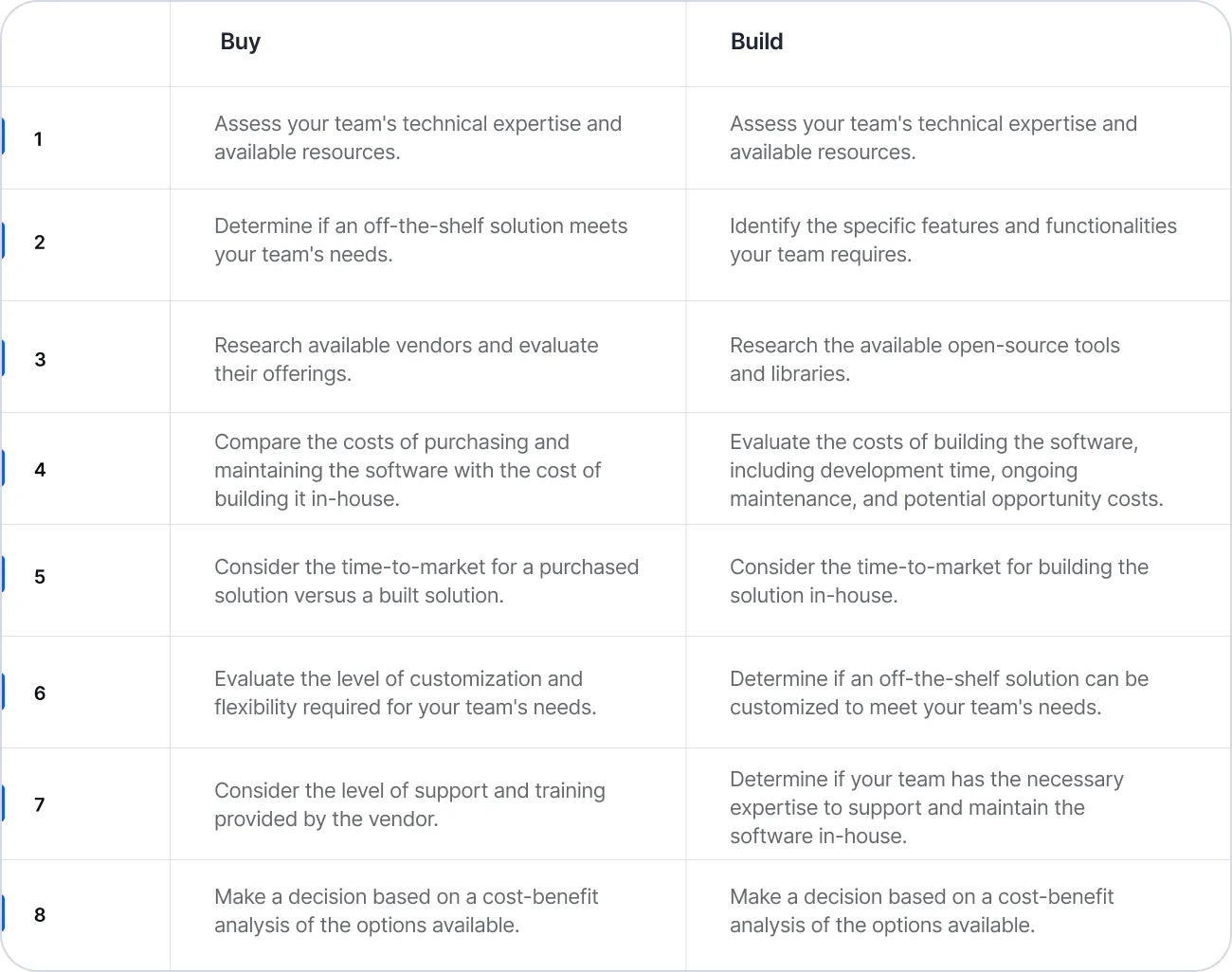
Armed with this knowledge, you’re bound to make the right decision for your project.
Eager to see how V7 stacks against your internal solution? Request a demo now.
As a former Content Specialist in V7, Marta reported on the advancements in the AI landscape and the achievements of the V7 customers & team.