AI implementation
How to Build AI-Powered Digital Pathology Products
12 min read
—
Jul 3, 2023
AI is fielding phenomenal advances in the digital pathology market, from smart drug delivery to preventative care. We outline the opportunity at hand and explain the pros, pitfalls, and possibilities of AI-powered digital pathology products.
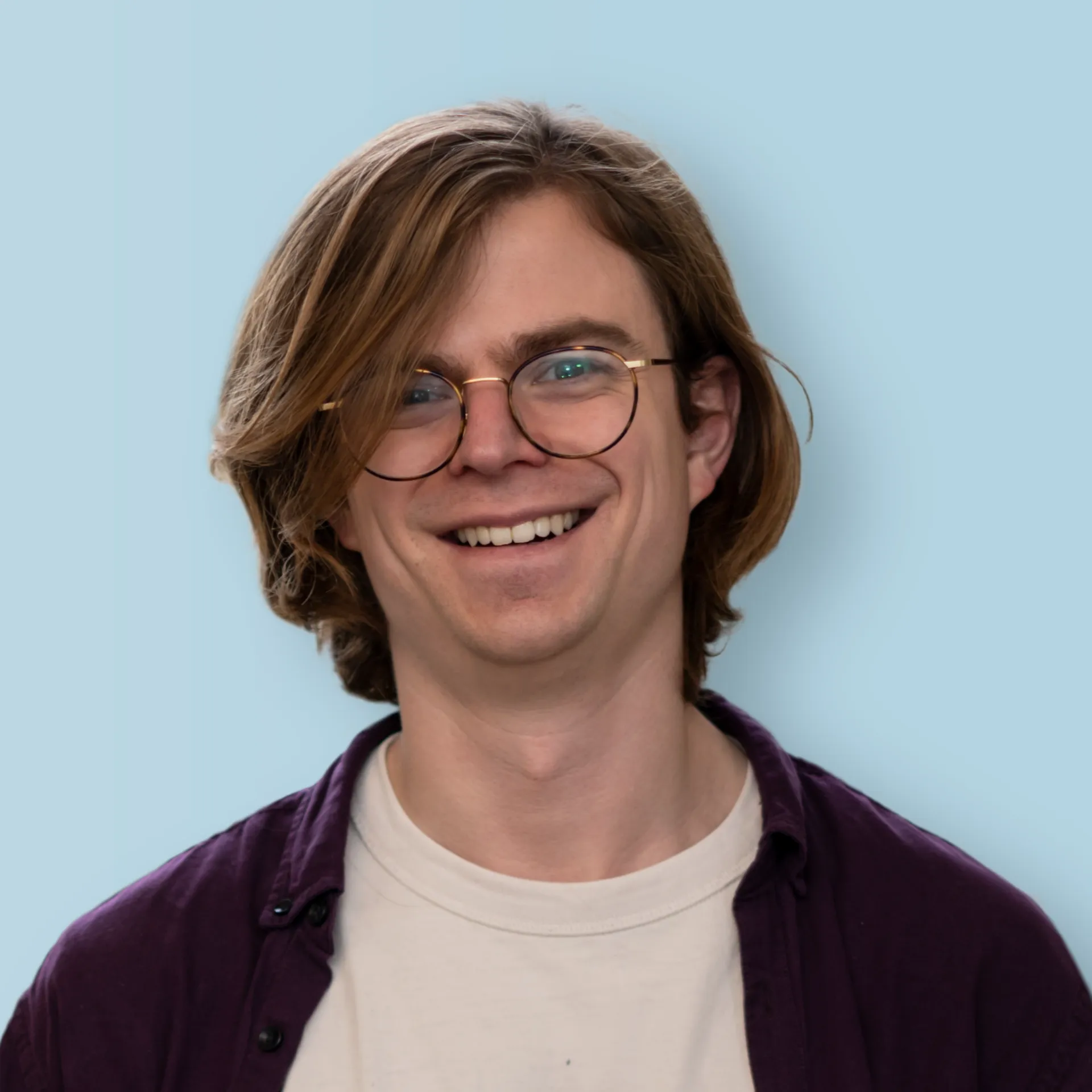
Former Head of Customer Success
As the rate of AI innovation accelerates, game-changing AI products continue to come to the fore. However, while progress is rapid in unregulated fields, for industries like digital pathology, progress is significantly more complex. While many digital pathology companies realize the necessity to innovate with AI, the fact remains - challenges lie in wait.
Handled correctly - digital pathology innovators have a phenomenal opportunity ahead, with cutting-edge products that dramatically advance the medical field. So much so, that the global digital pathology market is expected to expand at a compound annual growth rate of 19.31% until 2030. An impressive feat, given that the market is currently valued at $482.9 million.
However, without the right infrastructure and strategy in place, businesses run the risk of creating pathology products destined to fail. From eye-watering costs to regulatory limitations, healthcare faces complex hurdles to reach the promised land.
So, what’s the solution?
What’s the recipe for AI product success in healthcare?
And how can digital pathology practitioners create fail-safe AI products that appease the appetites of the FDA?
In this article, we explain how to create digital pathology products using AI, with expert insights myself, Principle Customer Success Expert, James Hudson, Deep Learning Lead Jon Almazán, Medical Account Executive Jason Leigh, and Medical Customer Success Expert, Jade Yip. We’ll address…
Digital Pathology and AI: A brief history
The biggest challenges facing digital pathology AI products
The solution for AI products
Digital pathology product success
How to create fail-safe medical products
10 trends in AI healthcare development
A tutorial on accelerating AI model development
Digital Pathology and AI: A brief history
The history of pathology is long and winding. However, seismic industry shifts began in 1999, when Whole-Slide-Imaging (WSI) was introduced. This opened the doors for the digitization of invaluable medical data, and similarly led to the popularisation of the term “digital pathology”. Since then, digital pathology has evolved greatly, shifting to keep pace with an accelerated rate of innovation that has seen sci-fi-esque evolutions within the medical space.
Since the turn of the century, the industry has witnessed innovations across smart drug delivery services, digital pathology-guided robotics surgeries, and AI-enabled wearable interfaces.
In fact, the healthcare industry is heading rapidly into a new and exciting frontier, with AI at its very center. In leveraging advances in artificial intelligence, IoT, robotics, and augmented reality, the healthcare industry now faces a future driven by digital innovation.
The biggest challenges facing digital pathology products
Before we dive into the success stories of digital pathology, it’s worth addressing the hurdles these innovators have had to overcome. Similarly, let's address just some of the ways these challenges can be scaled.
Data, or lack thereof
Digital pathology products necessitate annotated datasets to provide the context from which a model will be trained. However, the process is not always simple. At the heart of any reliable model is a good-quality dataset - however - readily available labeled digital pathology datasets are hard to come by - particularly when medical data often needs to be anonymized.
By its very nature, digital pathology requires specialist knowledge, which in turn means datasets undergo a series of expert reviews which can greatly slow down the process of securing reliably labeled data. Similarly, the process itself can be a time-intensive one, resulting in missed project deadlines, ballooning budgets, and wasted resources as a result of ineffective annotation systems.
Beyond the challenge of annotating data - digital pathology faces a unique hurdle because of the monumental size of WSIs. So much so, most annotation tools struggle to render 1GB+ WSIs - resulting in unsatisfying workarounds that further slow down the process. All in all, without the right infrastructure in place, data annotation can be a prohibitively inefficient process that hinders game-changing projects from their very beginning.
Check out our end-to-end playbook on the digital annotation process.
Cost of annotation and AI ownership
Accurate AI models are reliant on accurate training data, which can be prohibitively expensive within digital pathology. Unlike more simple annotation tasks, like addressing commonplace items in the world, digital pathology is a highly nuanced field - meaning annotation necessitates highly skilled - and expensive - labelers.
Without the right foundations, creating accurate training data can drain resources, whether that’s hard-earned cash, or the valuable time of digital pathology annotators.
Compliance and security
The medical industry is cocooned in regulatory red tape - and rightly so. However, that means that in the context of creating digital pathology AI products, projects can be halted and eternally shelved as a result of lacking compliance. For those that move forward absent of regulatory approval, limited clinical adoption awaits, and with it - limited financial returns.
Any regulatory expert will tell you: start with compliance from the outset. In the context of medical products, this requires compliant tooling, auditing, and secure data storage and processing. For those creating digital pathology products, FDA, HIPAA, SOc2, and GDPR will be top of mind.
V7 is FDA, HIPAA, SOc2, and GDPR compliant. Explore our commitment to FDA compliance here.
What’s the solution?
Faced with regulatory red tape and the overarching challenge of securing high-quality training data delivered quickly at low cost, you may be wondering - what’s the solution?
V7 has made huge commercial investments to back the medical industry, shaping its platform to support game-changing products from GE Healthcare, Bayer, and Boston Scientific. As a result, the V7 platform is uniquely suited to digital pathology products. How exactly, you ask? Well…
Compliance is built-in to our platform, meaning we’re FDA compliant, HIPAA compliant, SOC 2 compliant, ISO 27001 compliant, and GDPR compliant. In other words, our infrastructure effectively sets reliable foundations for your product to secure FDA approval.
Unlike other data annotation tools on the market, V7 delivers reliable WSI support, meaning the process is uninterrupted and backed by easy-to-use UX.
Our platform includes workflows for increased quality and throughput - in addition to model-assisted labeling that drastically reduces the cost of data labeling. And, to ensure best-in-class training data and models, our consensus stage allows teams to resolve disagreements in an easy-to-use workflow.
No man is an island - and the same can be said of the V7 platform. We support direct integrations with AWS S3, GCP, Azure Blob, and Min.lo storage.
Finally, we equip our customers with a global network of medical annotation partners, reducing the time, cost, and effort of sourcing a medical annotation team.
Discover the fit-for-purpose features V7 provides for digital pathology product creators.
Digital pathology product success
The digital pathology industry boasts a host of success stories, backed by rigorous infrastructures that bolster the delivery of successful products. One such product success is Genmab, an international biotech company using V7 to drastically speed up the detection of tumors in digital pathology images. Working closely with machine learning engineers, Genmab’s board-certified pathologists have created deep learning models that can understand the distribution of tumor and immune cells. And, they’ve done so by leveraging the full impact of AI to move the needle within their space.
To learn more about Genmab’s digital pathology success, you can check out our case study on how they did it.
How to create AI-powered medical products
Success for digital pathology products relies on a host of factors, from cost-effective delivery to achieving FDA compliance. Below, we address core actions you can take to ensure the creation of reliable medical products with impact.
Workflows
Ensuring the efficient annotation of data is easier said than done - particularly when confronted with large-scale datasets requiring specialist insight. In V7’s workflow system, annotators can be easily managed, tasks can be distributed, and checks and balances can be securely put in place. Similarly, realtime collaboration is seamless, allowing teams to quickly resolve disagreements.
Below, Jon and I run through a sample workflow that addresses common challenges found within digital pathology, from nuanced annotation requirements to reserving the most complex of tasks for expert annotators.
Validation
Creating successful medical AI products relies on more than annotation precision. It’s all fine and well to create a model that boasts impressive accuracy, but how does it perform in the real world? Is your model an indecipherable black box? Or can you clearly explain its outputs to a human? Similarly, can the model be embedded into a clinical workflow, improve the process, and deliver better outcomes? Or is it producing biases that harm the product overall?
You’ll need to be able to clearly address the explainability of your data, and outline how the AI operates within a workflow, and whether it actually makes actionable sense. Without this, your AI will fail overall. Put simply, validation and explainability will be key to creating a successful medical AI product - meaning you’ll need to embed this into your process from the start.
Data and WSIs
The scale of WSIs requires powerful infrastructure that can adequately process and manage the format. To do so, digital pathology teams require a system that can not only handle large WSIs, but do so in a way that’s intuitive and efficient.
Below, you can see how the V7 platform seamlessly handles a WSI onscreen, in a process that can be likened to the visual structure of Google Maps. Due to the design of V7’s platform, digital pathology annotators can swiftly move through images without facing workaround hurdles that slow the process.
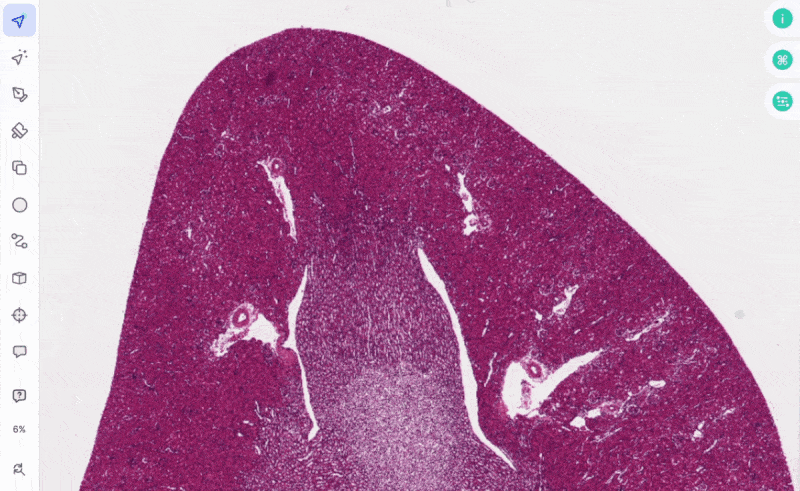
Consensus
Digital pathology is particularly complex, which means disagreements can arise around the accuracy of annotated data. This is particularly true of the healthcare space, where nuanced data is common. To combat this, V7 has an in-built consensus stage that upholds the accuracy of complex data while reducing the time spent on detailed annotation.
A consensus stage will allow you to track the outputs of labelers, assess the quality of annotations, and embed checks that ensure expert reviewers only spend time on the most important tasks.
This also allows you to reduce the cost of medical labeling, a traditionally costly venture, while still delivering highly accurate outputs.
This embeds accountability for training data, while building-in quality-control processes that uphold the integrity of the data and its output.
Model-assisted labelling
We don’t need to tell you that digital pathology labelling can be a time-intensive and costly process. However, there are steps you can take to drastically reduce the time and money spent on labelling data. Let’s say you want to annotate a nucleus within a series of WSIs. Rather than tasking your annotation team with thousands of hours of annotation, you can support the process with a model that has been trained to annotate nuclei at scale. And, when it comes to upholding data quality, you can correct and review the results of the model - continuously training the model to iteratively improve its impact on your budget, and the time spent annotating.
Perhaps you’ve already built a model that’s trained to your specific needs. You can easily import this model into V7 to embed it as part of your labeling process, enabling model-assisted labeling with a fine-tuned model of your own.
Already armed with a model that can support your workflow? Discover how the V7 platform can seamlessly onboard your model, to allow it to work from day one.
Work with regulatory approval in mind
To secure regulatory approval, for example from the FDA, you need to demonstrate that your ground truth is achieved in a clinically verifiable way. V7 houses your project with platform infrastructure that helps you achieve that. While V7’s annotated data can’t be directly used for FDA approval, it does fast-track much of the groundwork needed to create FDA-worthy products.
The future of digital pathology
As healthcare faces a new frontier, emboldened by advancements in AI, robotics, and augmented reality, many await the exciting innovations the industry promises to deliver. And yet, countless products fail to reach their full potential, stifled by prohibitive costs, ineffective tools, insufficient data, and lacking regulatory oversight. For those scaling some of the most pressing challenges, a series of trends have begun to emerge.
5 trends in AI healthcare development
Jason Leigh, V7’s healthcare-focused Commercial Account Executive, put together some of the key changes, tactics, and trends in healthcare AI development.
Foundational models and large language models
Foundational models are having their day in the sun, with the likes of GPT-4, LaMDA, and SAM dominating headlines. Leaders are beginning to explore the practical uses of foundational models, like SAM, on healthcare data. While a world of possibilities awaits, a key consideration will be developing UX that ultimately works for radiologists.
Similarly, innovators are exploring practical applications for FM and LLM, particularly around querying large datasets of non-visual data. In other words, the ability to search specialized medical imaging through text prompts is on the horizon…
Automations
With cost-effectiveness, quality, and repeatability in mind, digital pathology teams are automating workflows and standardizing their training data processes. This saves costly engineering time on Python scripting, removes confusion, and empowers their AI teams to scale.
Model-in-the-loop pipelines and performance
As we’ve already addressed, model-in-the-loop pipelines are a powerful way to automate less complex tasks. By embedding a model in the loop, digital pathology teams can cut down on labeling costs, while reserving expert eyes for more nuanced tasks. This tactic is catching on within the industry at large, boosting productivity and model performance in the process.
When it comes to the performance of models, leaders are similarly identifying edge cases where models underperform, using model-vs-model and model-vs-human workflows. This massively advances the iterative learning approach of their teams.
Real-time reporting
AI healthcare development can be a costly, time-consuming, and complex process. To monitor the performance and viability of projects, leaders are increasingly seeking visibility over their training data operations with real-time reporting. This allows teams to remove bottlenecks, improve performance, and create cost-effective models that are superior in make.
Robust QA
Quality assurance is a guiding principle of AI healthcare development, which often leads to spiraling costs in the pursuit of ground truth. Leaders are now cementing their QA review process by implementing structures that intelligently spread tasks across labelers, models, and industry experts. The result? Accurate ground truth: efficiently achieved.
Create an AI-powered digital pathology product
At V7, our mission is to encode human knowledge into computers, to solve the world’s most crucial tasks and give us longer, happier lives. Few industries exemplify that mission better than the digital pathology space, which works tirelessly to better the quality, longevity, and sustainability of human life. And, backed by the rocket-fuel infrastructure of V7, these companies are empowered to innovate a brighter future into existence.
Preparing to create a digital pathology product? Request a demo to see how V7 can bolster cutting-edge ideas with cutting-edge infrastructure.