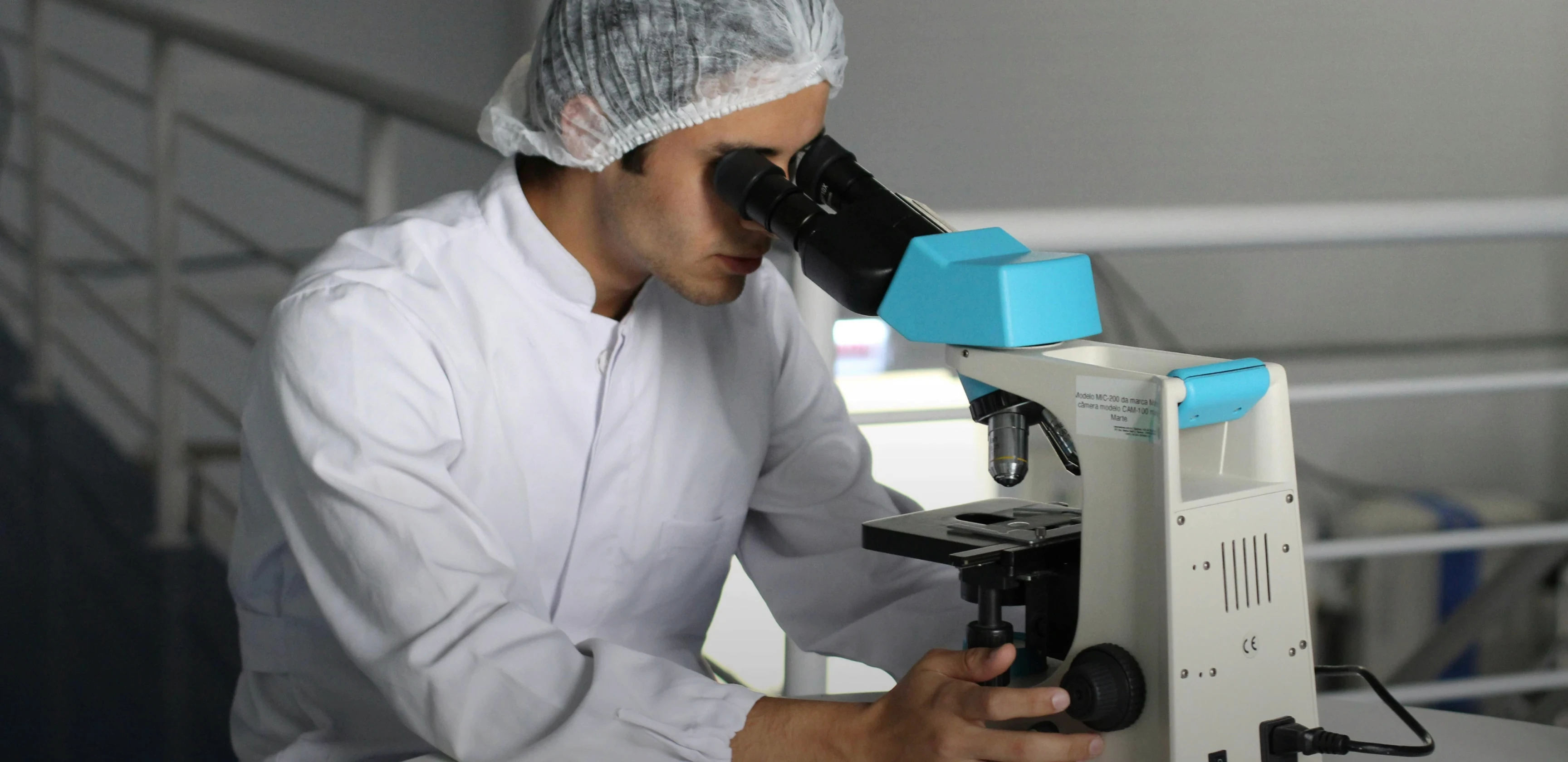
Developing antibody therapeutics for cancer treatments
Industry
Healthcare
Use Case
Cell Segmentation
Company size
1,600+ employees
Headquarters
Copenhagen, Denmark
Data labelling
Discover our solutions
About
Founded in 1999 in Copenhagen, Denmark, Genmab is a leading biotechnology company. It specializes in creating and developing novel antibody therapeutics for cancer treatment.
The team leverages data science and machine learning to extract novel insights regarding indications, targets, investigational products, and potential patient biomarkers. These findings are used for training deep learning models for large-scale image classification that improve cancer diagnosis, prognosis, and treatment selection.
Genmab’s data science team currently focuses on integrating AI/Machine Learning technology across genomic, transcriptomic, proteomic, and digital pathology. The goal is to augment clinical trial data analysis and decision-making that have profound biological, prognostic, and potential therapeutic implications.
The challenge
A biotechnological company, Genmab, uses AI-powered tissue diagnostics to get potentially life-changing insights from histopathology slides. Those insights are used for facilitating cancer therapeutics development that improves patient outcomes.
However, annotating biomedical data requires complex tooling, time, precision, and a robust review system to minimize errors.
Genmab aims to automate the digital and computational pathology workflows and build reliable computer vision models that will help analyze the tumor immune micro-environment and gain insights from clinical studies to inform therapeutics development.
The solution
Genmab uses V7 to label, review, and score digital pathology images faster and create high-quality training and validation data for computer vision.
Thanks to V7, the team has built robust annotation and QA workflows, onboarded a team of experienced pathologists to evaluate pathology images, and annotated more than 5,000 images for sample tissue analysis.
The results
So far, Genmab has annotated over 5,000 images on V7 (and counting).
The team found V7 easy to learn and use, which significantly sped up the onboarding process of new annotators. This, in turn, helped the team to create training data more efficiently and iteratively improve their deep learning models to higher accuracy.
V7 has also enabled Genmab’s team to label multiple tiles simultaneously, filter, review, and score images more easily, as well as fix errors on the go.
Training data needs
Genmab uses V7 to label complex digital pathology images (slides) to aid tumor classification based on tissue characteristics.
The in-house team of board-certified pathologists works closely with machine learning engineers to build deep learning models that understand the distribution of tumor and immune cells.
In their current project with V7, Genmab trains image classifiers and uses V7’s platform to annotate tiled sections of images using tags. The team also occasionally labels their data using polygons to identify other objects present in the images.
Why V7?
Genmab was validating several tools, including commercial platforms and open source solutions before settling on V7.
One of the many reasons the team chose V7 was its robust annotation workflows system. Genmab needed a tool that could help them streamline their annotation and QA process, and facilitate real-time collaboration between the annotators (pathologists) and the machine learning team.
The API, intuitive interface, multi-level quality assurance system, and advanced dataset management features have helped Genmab speed up the labeling process, organize data, and deploy AI faster.