Playbooks
Training Data Platform Buying Guide
4 min read
—
Oct 25, 2022
Learn to navigate the process of buying a training data platform. Understand key stakeholders in your organization, evaluation criteria, and the purchasing process.
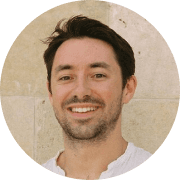
Former Head of Sales
As a fairly new category of software, there is no defined process for purchasing a training data platform. This guide will help you navigate this process, whether that’s through a formal RFP process or through a more informal purchasing cycle. It will cover stakeholders, evaluation criteria, and purchasing processes.
Vision AI & Training Data
Vision AI is a discipline of Machine Learning focussing on unstructured data. It can easily be broken down into two parts:
Model selection and hyperparameter tuning
Training data
Previously, research has been focused on model selection and hyperparameter tuning, but increasingly Google, Tesla, Facebook, and other top AI companies have focused on realizing gains from training data.
Google estimates that 83% of models fail because of poor training data management, but there are also significant performance benefits from experimentation with training data, and great AI companies focus significant time on this experimentation.
What is a training data platform?
A training data platform forms part of the modern MLOps stack. It should enable the team not only to scale their training data but also to run experiments on that data to realize efficiency gains.
The process of annotating data is the core functionality of a training data platform, but good training data platforms allow for rigorous QA processes and provide a dataset management module that can allow users to realize, and explain, gains provided by better training data utilization.
A training data platform should not be expected to provide new raw data or full end-to-end production AI. It is part of a broader machine learning stack, including raw data capture, training environments, hyperparameter tuning modules, and production hardware. As such, good training data platforms should have a flexible, open API to allow for easy integration into broader stacks.
Preparing your organization for a training data platform evaluation
Stakeholders
A rigorous training data platform evaluation should consider the concerns of four key stakeholder groups
Your Annotation workforce
Your Annotator Management team (sometimes can also be part of point 3)
Your data science/computer vision engineering team
Executive stakeholders
Before beginning an evaluation of training data platform providers, consult each of these groups. Find out what they need from a tool, what they want from a tool, and what they can’t do with the current system (or anything in particular they dislike about this).
Typical priorities and roles in the evaluation process for these groups can be broken down in the table on the next page (which is by no means exhaustive).
Benchmarks
For any software purchasing decision, there will be qualitative factors (e.g. quality of support, UI), but anything that can be measured should be, both against the status quo and against other players in the evaluation
A good approach is to pick a limited number of projects to test and to evaluate those against key measurable criteria
Speed of annotation
Accuracy of annotation
Speed of administration
End-to-end project time
These projects should be varied across annotation and data types (i.e. if you’re doing semantic segmentation of MRIs, and classification of x-rays, test both projects on the platform). You should gather the existing benchmarks across these areas.
Table 1: The roles in a training data management platform evaluation
📥 Download: Training Data Management Platform Roles Overview
Table 2: Feature checklist and scoring
Every set of requirements is slightly different, but this should provide a good overall breakdown. A weighted priority score has been suggested, but can be adjusted. Some items, of course, will be breaking.
📥 Download: Features Checklist
Download other charts
| 📥 Data Types and Annotation Types
| 📥 Annotator Speed and Efficiency
| 📥 AI Models
| 📥 API & SDK
Conclusion
As discussed, every team has different requirements, and the tables above should be adjusted according to your own schema. Security should always be an essential requirement, but others can adjusted based on needs or complexity of needs. If you need any further assistance, please reach out to Matt Brown, matt@v7labs.com, who can help with any further clarifications.