Document processing
18 min read
—
May 19, 2025
Dive into how AI is reshaping real estate lease abstraction, enhancing efficiency, accuracy, and cost savings, and what it means for the future of property management.
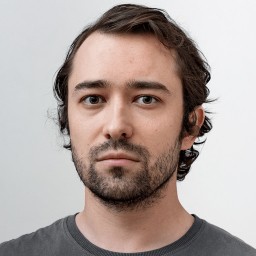
Content Creator
The real estate industry is currently navigating a significant operational shift, driven by the integration of artificial intelligence (AI), especially within the intricate domain of lease abstraction. Traditionally, extracting key data points from lease agreements has been a labor-intensive, time-consuming, and error-prone endeavor, often taking four to eight hours per lease. Industry professionals confirm that even for experienced hands, manually summarizing leases for internal records can easily consume an hour per document. This underscores the pervasive nature of a challenge magnified by commercial leases, which frequently span dozens or even hundreds of pages filled with complex clauses and amendments, rendering purely manual abstraction increasingly unsustainable.
AI, particularly sophisticated platforms like V7 Go, offers a pragmatic solution by automating the extraction, structuring, and analysis of lease data. This not only accelerates processing but also significantly enhances accuracy and reduces the risk of overlooking critical details—a common pitfall in manual reviews. The market for AI in real estate reflects this strong demand, with projections showing substantial growth as businesses seek to harness these efficiencies.
In this article, we'll explore:
The fundamental process of lease abstraction and its traditional limitations.
The burgeoning market for AI in real estate and lease management.
How AI, encompassing machine learning and generative AI, is applied to lease abstraction and the key benefits it offers.
The role of AI-powered lease abstraction in broader knowledge work automation.
Practical considerations, challenges, and the future trajectory of AI in this domain, including insights from industry professionals.
Understanding the nuances of AI application in lease abstraction is crucial for stakeholders aiming to leverage these advancements to streamline operations, achieve considerable cost savings, and maintain a competitive edge in the evolving real estate sector.
What is Lease Abstraction in Real Estate?
Lease abstraction is the critical business function of meticulously extracting pertinent data points from voluminous lease agreements and related documents, then organizing this information into a concise, structured, and easily understandable summary known as a lease abstract. This process involves a careful review of often complex legal documents to highlight key information such as lease terms, financial obligations (base rent, escalations, operating expenses), rights and responsibilities of landlords and tenants, critical dates (commencement, expiration, renewal options, notice periods), and specific clauses (e.g., termination, subletting, co-tenancy, exclusive use). As noted by Ascendix Technologies in their guide to lease abstracts, this summary serves as a quick reference, eliminating the need to repeatedly consult the entire lengthy document.
The importance of accurate lease abstraction spans various stakeholders in commercial real estate. Property managers rely on abstracts for efficient portfolio management and tracking tenant obligations. Landlords use them for revenue optimization and risk mitigation. Investors depend on them during due diligence to assess portfolio performance and inform acquisition strategies. Legal teams utilize abstracts for quick contract review, while accounting and finance teams need them for financial reporting under standards like IFRS 16 and ASC 842, ensuring crucial financial data is accurately captured, as highlighted by Accruent.

AI agents in platforms like V7 Go can orchestrate complex lease abstraction workflows, from document ingestion and OCR to data extraction and validation, streamlining the process for real estate professionals.
The Drudgery and Pitfalls of Traditional Lease Abstraction
The traditional approach to lease abstraction is a deeply manual and painstaking process. Skilled professionals, often lease administrators or legal experts, meticulously pore over each lease agreement, including all amendments, riders, and addenda. This demands a profound understanding of legal and commercial real estate terminology to pinpoint specific data points relevant for lease management and decision-making, a process detailed by RE BackOffice. Information is manually extracted, summarized into standardized templates, and then entered into databases or lease administration systems. Often, a secondary review is implemented for quality control.
This manual method is inherently inefficient and fraught with challenges:
Extremely Time-Consuming: Commercial leases can be monstrously long. Manually summarizing a single lease can take 4 to 8 hours or more. Imagine that labor multiplied across a large portfolio; it’s a significant resource black hole.
Human Error is Inevitable: Let's be frank, humans make mistakes, especially when facing tedious tasks. Manual data extraction is a breeding ground for errors that, as Springbord outlines, can mushroom into costly inaccuracies, legal battles, or missed financial opportunities like renewal options.
Inconsistency and Lack of Standardization: Different people interpret complex lease language differently. This subjective element, also emphasized by Springbord, results in frustrating variations in abstracted data, making reliable comparisons and portfolio-wide analysis a nightmare.
Scalability? Forget About It: Manual processes are notoriously difficult to scale. As lease volumes swell, decision-making grinds to a halt, and responsiveness plummets – a well-documented challenge by industry analysts like Springbord.
The Peril of Overlooked Details: Within the dense jungles of lease legalese, critical clauses or hidden financial implications can easily be missed, potentially leading to unforeseen financial or legal nightmares, a risk highlighted by CREModels.
The sheer variability of leases, a point frequently echoed by property managers in online forums, makes manual standardization a Herculean task. Even when dealing with multiple leases from the same tenant company, almost every document will have unique variations, compounding the difficulty of purely manual approaches.
The AI Intervention: A New Dawn for Lease Abstraction
Recognizing these persistent challenges, the real estate industry has turned, with increasing enthusiasm, to artificial intelligence. AI technologies, particularly Optical Character Recognition (OCR), Natural Language Processing (NLP), Machine Learning (ML), and Generative AI (GenAI), are genuinely advancing lease abstraction:
OCR acts as the initial workhorse, converting scanned lease documents (PDFs, images—you name it) into machine-readable text, making the inaccessible accessible.
NLP algorithms then step in, functioning like a seasoned legal mind to interpret complex legal language, accurately extracting key terms, clauses, and obligations.
Machine Learning models, as highlighted by Trellis AI's blog on automation, are trained on vast datasets of leases. They don't just learn; they continuously improve their ability to identify and extract relevant information with increasing accuracy, even recognizing sophisticated patterns within lease agreements.
Generative AI, the star of the current AI show, excels at summarizing, analyzing, and, as Trullion notes, even interrogating lease contracts to provide insights that go far beyond simple data points.
Platforms like V7 Go embody this AI-led improvement. It can ingest lease documents in a multitude of formats, deploy AI agents to manage the entire abstraction workflow (including OCR, data extraction, and sophisticated validation routines), and present the structured data in an intuitively accessible format. Its AI Citations feature is particularly noteworthy, offering visual grounding by linking every extracted data point directly to its origin in the source document. This ensures an unprecedented level of transparency and dramatically simplifies human review. Furthermore, V7 Go’s Knowledge Hub allows users to upload entire libraries of leases, effectively creating a dynamic, searchable knowledge base that AI can query using Retrieval-Augmented Generation (RAG) to deliver contextually precise answers—turning static documents into active intelligence.
Market Momentum: AI in Real Estate and Lease Management
The global market for AI in real estate is exploding. Valued at USD 2.9 billion in 2024, projections show a surge to USD 41.5 billion by 2033. That’s a compound annual growth rate (CAGR) of 30.5%. Another report, from Maximize Market Research, paints an even more aggressive picture, estimating the market could reach USD 1803.45 billion by 2030 at a 35% CAGR. This isn't speculative hype; it reflects solid investor confidence and rapidly increasing adoption of AI solutions across every segment of the real estate value chain.
Within this AI boom, the lease management software market, a critical arena for AI-powered lease abstraction, is also on a steep upward trajectory. One estimate from Grand View Research places its value at USD 5.65 billion in 2024, expecting it to hit USD 8.13 billion by 2030 (a CAGR of 6.4%).
What’s fueling this fire? According to an MRI Software blog, the primary drivers are threefold: the non-negotiable need for improved efficiency and accuracy in managing increasingly complex lease portfolios; the relentless demand for cost reduction; and the critical necessity to comply with evolving, and often bewildering, accounting standards like IFRS 16 and ASC 842.
AI Lease Abstraction: Key Functionalities and Tangible Benefits
AI-powered lease abstraction solutions offer a suite of functionalities designed to streamline the entire lease management lifecycle. These typically include:
Versatile Document Ingestion: The ability to upload and process lease documents in a multitude of formats—PDFs (both native and scanned), images, and Word files—is fundamental.
Automated and Intelligent Data Extraction: Here, AI truly shines. It doesn't just find data; it understands it. Accruent highlights how AI can identify and capture critical data points such as key dates (lease start/end, rent commencement, option deadlines), intricate financial details (base rent, complex escalation schedules, CAM charges, security deposits), party information (tenant, landlord, guarantors), and highly specific clauses (use restrictions, co-tenancy provisions, subletting rights, detailed maintenance responsibilities).
Systematic Data Structuring: Extracted data is meticulously organized into standardized, easily accessible, and—crucially—usable formats, ready for analysis or integration.
Robust Integration Capabilities: The real power of AI lease abstraction is often unlocked through its ability to connect with the broader real estate tech ecosystem. Many tools offer seamless, out-of-the-box integration with dominant property management systems like Yardi or MRI. More advanced platforms, such as V7 Go, provide comprehensive APIs and Zapier integrations, allowing for deep, custom connections to almost any existing system.
Proactive Risk Assessment & Compliance Monitoring: AI can be a vigilant watchdog, automatically flagging potentially unfavorable clauses, identifying upcoming deadlines or early termination penalties that carry financial implications, and critically assisting in ensuring compliance with complex accounting standards (IFRS 16, ASC 842) and other evolving regulatory requirements.
Advanced Analytics & Strategic Portfolio Management: Beyond simple extraction, the structured data becomes a goldmine for insights. It can be analyzed to identify trends, benchmark property performance, optimize revenue streams, and inform high-level strategic asset management decisions.

AI-powered lease abstraction platforms can intelligently extract and structure critical data from complex lease documents, turning dense legal text into actionable insights with speed and precision.
The adoption of AI in lease abstraction delivers substantial, often game-changing, benefits. Instead of just a feature list, consider the profound shift: traditional methods involve professionals spending 4-8 hours or more on a single lease, battling human error, and producing inconsistent data. AI flips this script. Processing times can drop to minutes per document. Accuracy often exceeds 99%, as noted by Springbord, reducing costly mistakes. This translates to cost savings frequently in the 50-90% range. AI handles vast portfolios with ease, and data becomes a centralized, actionable asset.
Learn more: How to Analyze Virtual Data Rooms with AI: M&A and Beyond
Machine Learning vs. Generative AI in Lease Abstraction: Understanding the Toolkit
When delving into the AI landscape for lease abstraction, it's crucial not to paint with too broad a brush. We're primarily dealing with two distinct, yet often complementary, AI methodologies: Machine Learning (ML) and Generative AI (GenAI). As repeatedly emphasized in operational feedback I've encountered, confusing these is a fast track to misaligned expectations – especially when you expect an LLM to crunch numbers like a dedicated ML forecasting model, or vice-versa.
Machine Learning: The Pattern Detective and Forecaster
Think of Machine Learning algorithms as tireless analysts of historical lease data, as described in an MRI Software blog. By training on vast datasets of past agreements, ML models become adept at identifying patterns, trends, and correlations. In lease abstraction, this translates to capabilities like:
Predicting lease renewals: By analyzing historical renewal rates, tenant behavior, and current market conditions, ML can forecast the likelihood of a tenant renewing their lease.
Identifying market trends: ML can discern prevailing patterns in lease terms, rental rates, and concession packages across a market or portfolio, providing valuable intelligence for negotiations and strategic planning.
Forecasting tenant behavior: Factors like payment history, business stability (if inferable from data), and lease compliance can be fed into ML models to predict potential issues such as the likelihood of default, thus aiding in proactive risk assessment.
Common ML techniques employed here, according to a Ylopo blog post, include regression analysis (excellent for predicting numerical values like future rent prices) and classification models (useful for categorizing leases or tenants based on specific risk criteria or behavioral profiles). ML's strength lies in its ability to find signals in structured or semi-structured numerical data and make data-driven predictions.
Generative AI: The Language Maestro and Summarizer
Generative AI, especially in the form of Large Language Models (LLMs), has dramatically improved how we interact with textual information. These models are trained on colossal amounts of text and code, enabling them to understand and generate human-like language with remarkable sophistication. In lease abstraction, GenAI primarily plays the role of:
Automated Lease Abstract Generation: LLMs can read through lengthy, dense lease documents and generate concise, accurate summaries of key terms, critical dates, and financial obligations. They can identify and extract specific clauses even if the language is non-standard or convoluted.
Natural Language Interfaces and Q&A: LLMs can power conversational interfaces (like chatbots or within platforms like V7 Go's Concierge) that allow users to ask specific questions about lease agreements in plain English (e.g., "What are the tenant's CAM responsibilities for Property X?") and receive instant, contextually relevant answers, often with direct references to the source clause in the document.
While ML is focused on recognizing patterns and making predictions from primarily numerical or categorized data, GenAI is good at understanding the actual meaning, intent, and nuances within complex legal and financial language. However, it's crucial to remember GenAI's limitations: outputs, especially summaries or interpretations, *always* require human review for factual accuracy due to the potential for "hallucinations" (generating plausible but incorrect information). Furthermore, LLMs are not designed for complex mathematical calculations or for primary forecasting based on raw numerical inputs; these tasks are better suited to specialized ML models or traditional analytical tools. A platform like V7 Go recognizes this by allowing the orchestration of multiple AI models and tools, including the integration of Python scripts for custom calculations, bridging the strengths of different technologies.

V7 Go supports a range of sophisticated AI models, like those in the GPT-4.1 family shown here from a changelog entry, which are crucial for powering advanced GenAI lease abstraction tasks that require nuanced language understanding.
AI Lease Abstraction as Knowledge Work Automation
The concept of knowledge work automation revolves around leveraging technology to automate tasks that are predominantly reliant on specialized knowledge, expertise, and intricate analysis. The overarching aim is to boost efficiency, curtail errors, and, importantly, liberate knowledge workers from the morass of repetitive, mundane tasks. This allows them to redirect their cognitive energies toward more strategic, creative, and high-value endeavors. Lease abstraction, a process traditionally demanding a profound grasp of legal terminologies, financial intricacies, and specific real estate practices to accurately distill and summarize vital information from lease agreements, stands as a quintessential example of such knowledge work.
The advent of AI-powered lease abstraction tools marks a significant stride in automating this very specific and demanding knowledge work task. By automating the nuanced processes of extracting, structuring, and analyzing complex lease data, AI significantly streamlines and refines the workflows for real estate professionals. This automation is not merely about saving time; it's about fundamentally enhancing accuracy and mitigating the inherent risk of overlooking critical, often buried, details within lengthy legal documents. This successful application of AI in real estate and lease abstraction powerfully demonstrates its broader potential to similarly uplift other document-intensive and knowledge-based processes throughout the real estate industry, paving the way for substantial gains in operational efficiency and allowing a sharper focus on strategic objectives.
Voices from the Field: Real-World Challenges and User Desires in AI Lease Abstraction
Despite the impressive technological advancements, the practical implementation of AI for lease abstraction isn't always a walk in the park. Feedback from industry professionals, often voiced in online communities and forums, sheds light on the real-world hurdles and unmet needs:
The Specter of Data Security and Privacy: A recurring theme, as seen in discussions on platforms like Reddit, is the significant apprehension around uploading sensitive lease documents—laden with confidential financial and personal data—to third-party AI platforms. Larger organizations, in particular, often have established data governance protocols that make them wary, preferring secure, private deployment options or rigorously vetted in-house solutions. This highlights that trust and control over sensitive data are non-negotiable.
The Quest for Unwavering Accuracy and Reliability: While AI systems boast high accuracy rates, the legal and financial ramifications of errors in lease abstraction mean that human oversight is still universally considered vital. Users report instances where AI output, especially from general-purpose models, requires thorough human review to catch inaccuracies or misinterpretations of complex or ambiguous clauses. The phenomenon of AI "hallucinations" remains a concern, making features like V7 Go's AI Citations crucial for quick verification. Many landlords also express skepticism about AI's reliability for legally binding documents if not properly vetted.
The Legacy Document Labyrinth: A particularly frustrating challenge, frequently cited by users in online real estate forums, is the difficulty AI tools face with older, scanned legacy leases. These documents, often encountered during property acquisitions, may be of poor quality, handwritten in parts, or have inconsistent formatting, severely hampering OCR accuracy and subsequent AI analysis. Robust OCR and image pre-processing capabilities are essential here.
The Demand for Customization and Adaptability: Commercial real estate professionals emphasize that leases are rarely standardized; even within the same portfolio, terms can vary wildly. There's a strong desire for AI tools that offer greater customization, allowing users to define specific data points for extraction based on their unique needs and for the AI to learn from these preferences over time. V7 Go’s Auto Property feature, which uses natural language to help configure extraction, and its agent-cloning capability for creating tailored variations, aim to address this.
The Integration Imperative: For property managers, seamless integration of AI lease abstraction tools with existing property management systems like Yardi and MRI is not just a convenience but a key requirement for efficient workflow, a point strongly made by some industry practitioners online.
Niche Use Cases and Deeper Analysis: Beyond standard abstraction, users envision AI tackling more sophisticated tasks. For example, one user suggested an AI that could review multiple leases and generate a 'Use exclusives / prohibitions chart' to quickly vet potential tenants against existing restrictions. This points to a need for advanced cross-document analysis and logical reasoning capabilities.
Cost, ROI, and Market Saturation: While the benefits are clear, the cost of AI solutions and the time to demonstrate ROI are practical concerns. Some practitioners also noted the increasingly crowded market for AI lease abstraction tools, emphasizing that new solutions need clear differentiators to stand out.
The overarching consensus from these real-world experiences is that a hybrid approach—AI handling the heavy lifting of initial data processing, with human experts providing crucial validation, interpretation of complex scenarios, and strategic oversight—is currently the most effective and trusted model. This makes features that support human-in-the-loop workflows indispensable.
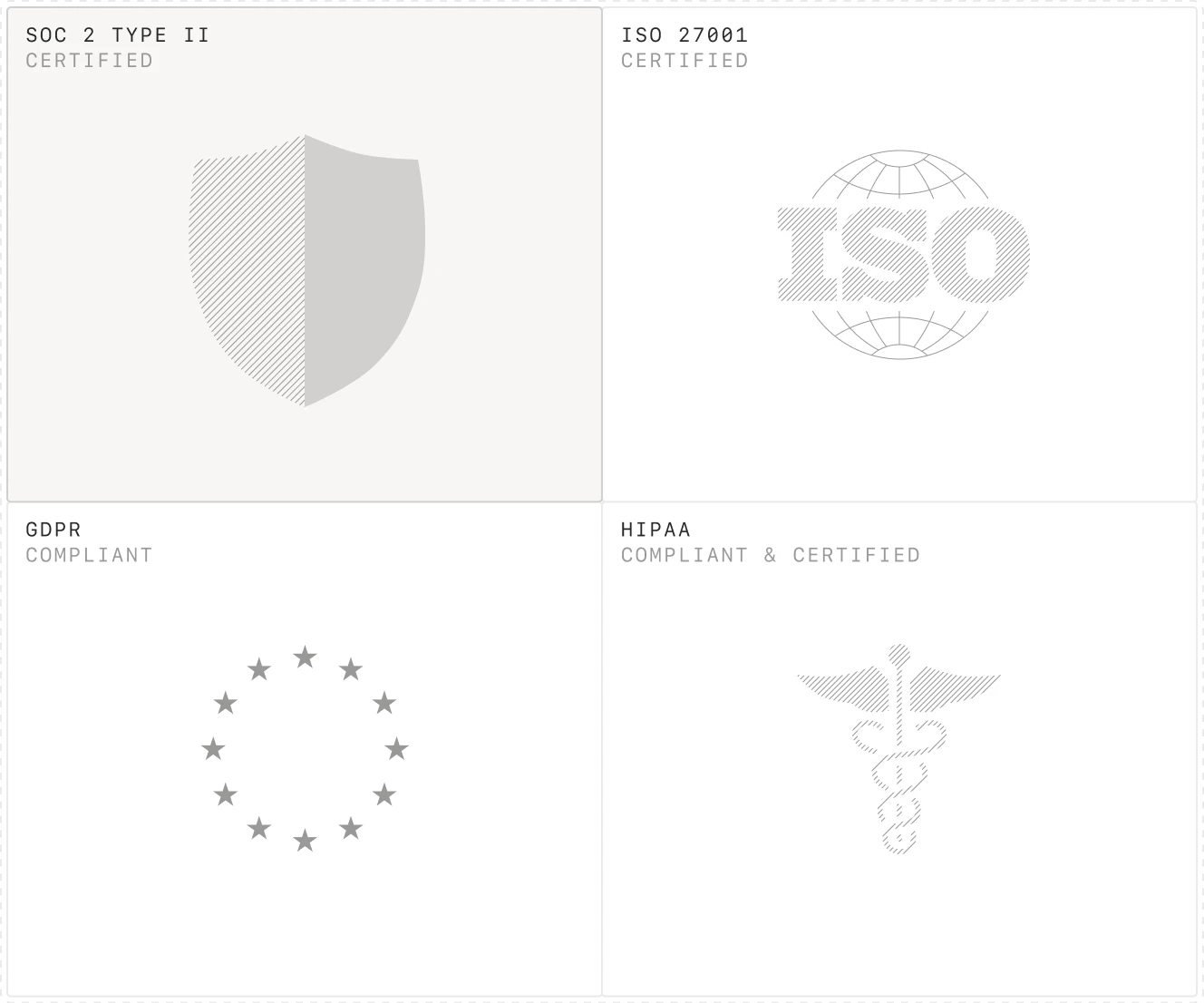
Data security and compliance (SOC 2, ISO 27001, GDPR, HIPAA) are paramount when selecting AI tools for lease abstraction, given the sensitive nature of lease data.
The Future Trajectory: What's Next for AI in Lease Abstraction?
The market for AI in real estate lease abstraction is on an acceleration curve, propelled by the unyielding industry demand for greater efficiency, pinpoint accuracy, and substantial cost savings in managing increasingly complex lease portfolios.
Looking forward, the evolution of AI in this space is likely to be characterized by several key developments:
Hyper-Refined Natural Language Processing (NLP): We can expect AI models to achieve even greater finesse in understanding and interpreting the intricate, often archaic, and highly nuanced language found in legal documents. This means better handling of ambiguities and context-specific terms.
Synergy with the Internet of Things (IoT): The integration of AI lease abstraction with IoT data from smart buildings opens up fascinating possibilities. Imagine AI automatically cross-referencing lease clauses regarding maintenance or utility usage with real-time building operational data, providing actionable alerts on compliance, performance, or even predictive maintenance needs.
Predictive Analytics Reaching New Depths: AI's forecasting capabilities will become more sophisticated, empowering real estate firms with sharper predictions of market trends, tenant behavior, and optimal lease terms, allowing for more proactive and strategic lease portfolio management, according to some industry analyses.
Automation Expanding its Horizons: AI's role will likely extend beyond just abstracting data. We could see increased automation in related routine tasks such as initial compliance checks, tracking critical dates with automated reminders, or even facilitating parts of the rent collection and reconciliation process.
Democratization of Advanced AI: As the technology matures and competition among solution providers increases, sophisticated AI lease abstraction tools are expected to become more accessible and affordable, bringing powerful capabilities within reach of a broader spectrum of real estate professionals and firms.
The Human-AI Symbiosis Perfected: The hybrid model of collaboration will become even more refined. AI will take on the lion's share of initial data processing and routine analysis, while human experts will increasingly focus on strategic oversight, validation of AI outputs in complex or high-stakes scenarios, nuanced interpretation, and leveraging AI-derived insights for decision-making. The focus will be on AI as an intelligent assistant that augments, rather than replaces, human expertise.
For businesses operating in the real estate sector, integrating AI into lease abstraction processes is rapidly shifting from a strategic option to an operational necessity. Early and thoughtful adoption of these technologies can forge a significant competitive advantage. This isn't just about streamlining operations or cutting costs. In the long run, it's about unlocking a higher level of data-driven intelligence that enables better, faster, and more informed decision-making in an ever-more complex and dynamic market environment.
The journey of Centerline, achieving a 35% productivity increase within the first month of using V7 Go for due diligence and data extraction, underscores the tangible benefits AI offers. While their use case focused on broader financial due diligence, the core activities—processing complex documents and extracting critical data—are directly analogous to the challenges in lease abstraction. This success story highlights how platforms like V7 Go, designed for versatile document intelligence, can be effectively applied to streamline lease abstraction and deliver significant operational improvements.
The continued evolution of AI promises to unlock even greater potential. As AI models become more adept at understanding specialized legal and financial language, and as integration with other business systems deepens, the role of AI as a strategic asset in real estate will only grow. The firms that proactively adopt and adapt to these technologies will be best positioned to navigate the future of lease management and the broader real estate industry.
If you are exploring how AI can enhance your lease abstraction processes, consider tools that offer robust document processing, configurable AI agents, and transparent verification features. Booking a demo with V7 Go can provide a practical first step in understanding how these advanced capabilities can be tailored to your specific real estate challenges.