Professional
Custom
Go
Go
Go Pricing
Go Pricing
Go Pricing
Workflow automation powered by AI agents with flexible pricing tailored to your needs.


Formula
Formula
Formula
Transparent pricing built for your workflows
We create a custom pricing package based on three key components:
Your price
Your total annual cost is the sum of:
Platform fee
+
User licenses
+
Data processing charges
Your price
Your total annual cost is the sum of:
Platform fee
+
User licenses
+
Data processing charges
Platform
Foundation access to V7 Go with specialized AI agents for finance, insurance, and legal workflows.

Platform
Foundation access to V7 Go with specialized AI agents for finance, insurance, and legal workflows.

Users
Flexible team access with customizable user roles and permissions.

Users
Flexible team access with customizable user roles and permissions.

Data
Volume-based pricing that scales with your document processing volume. Only pay for what you process, with options to expand as your needs grow.

Data
Volume-based pricing that scales with your document processing volume. Only pay for what you process, with options to expand as your needs grow.

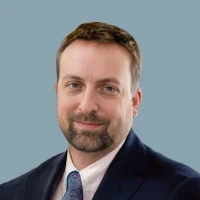
We used V7 Go to automate our diligence process with data extraction and automated analysis. This led to a 35% productivity increase in just the first month of use.
Trey Heath
CEO of Centerline
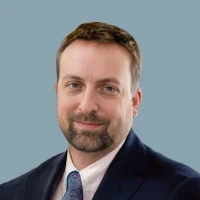
We used V7 Go to automate our diligence process with data extraction and automated analysis. This led to a 35% productivity increase in just the first month of use.
Trey Heath
CEO of Centerline
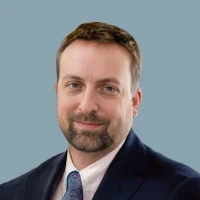
We used V7 Go to automate our diligence process with data extraction and automated analysis. This led to a 35% productivity increase in just the first month of use.
Trey Heath
CEO of Centerline

We use Collections on V7 Go to automate completion of our 20-page safety inspection reports. The system analyzes photos and supporting documentation and returns structured data for each question. It saves us hours on each report.
Ryan Ziegler
CEO of Certainty Software

We use Collections on V7 Go to automate completion of our 20-page safety inspection reports. The system analyzes photos and supporting documentation and returns structured data for each question. It saves us hours on each report.
Ryan Ziegler
CEO of Certainty Software

We use Collections on V7 Go to automate completion of our 20-page safety inspection reports. The system analyzes photos and supporting documentation and returns structured data for each question. It saves us hours on each report.
Ryan Ziegler
CEO of Certainty Software

We often bring complex data challenges to V7 and receive working solutions within hours. That kind of turnaround is game-changing.
Allen Darby
CEO of Alaris Acquisitions

We often bring complex data challenges to V7 and receive working solutions within hours. That kind of turnaround is game-changing.
Allen Darby
CEO of Alaris Acquisitions

We often bring complex data challenges to V7 and receive working solutions within hours. That kind of turnaround is game-changing.
Allen Darby
CEO of Alaris Acquisitions
Trusted by industry leaders
Trusted by fast-growing
companies around the world
Trusted by fast-growing
companies around the world

Get pricing
Let’s build your AI solution
Here’s why we create custom pricing plans:
Every organization uses our platform differently. During a brief 30-minute consultation, we'll assess your platform requirements, number of users, and anticipated data processing volume to provide an exact quote tailored to your needs with transparent pricing and no hidden fees.


Get pricing
Let’s build your AI solution
Here’s why we create custom pricing plans:
Every organization uses our platform differently. During a brief 30-minute consultation, we'll assess your platform requirements, number of users, and anticipated data processing volume to provide an exact quote tailored to your needs with transparent pricing and no hidden fees.

Get pricing
Let’s build your AI solution
Here’s why we create custom pricing plans:
Every organization uses our platform differently. During a brief 30-minute consultation, we'll assess your platform requirements, number of users, and anticipated data processing volume to provide an exact quote tailored to your needs with transparent pricing and no hidden fees.
FAQs
FAQs
FAQs
Have questions?
Find answers.
Why should I use Go instead of calling a model provider directly?
Go is more accurate and robust than calling a model provider directly. By breaking down complex tasks into reasoning steps with Index Knowledge, Go enables LLMs to query your data more accurately than an out of the box API call. Combining this with conditional logic, which can route high sensitivity data to a human review, Go builds robustness into your AI powered workflows.
+
Is V7 Go part of V7 Darwin?
No, they are separate products with different purposes. V7 Darwin is a data labeling platform for annotating images, videos and medical imaging to train your own models. V7 Go specializes in applying foundation models to automate document-intensive workflows through intelligent processing.
+
Does V7 Go support external models?
V7 Go supports a variety of foundation models through our platform, and you can also connect your own API keys to use models of your choice. This flexible approach allows you to select the best AI models for your specific workflows.
+
Can V7 Go recognize printed and handwritten text?
V7 Go is capable of recognizing both printed and handwritten text, leveraging advanced optical character recognition (OCR) technologies, as well as charts, diagrams and logos.
+
Why should I use Go instead of calling a model provider directly?
Go is more accurate and robust than calling a model provider directly. By breaking down complex tasks into reasoning steps with Index Knowledge, Go enables LLMs to query your data more accurately than an out of the box API call. Combining this with conditional logic, which can route high sensitivity data to a human review, Go builds robustness into your AI powered workflows.
+
Is V7 Go part of V7 Darwin?
No, they are separate products with different purposes. V7 Darwin is a data labeling platform for annotating images, videos and medical imaging to train your own models. V7 Go specializes in applying foundation models to automate document-intensive workflows through intelligent processing.
+
Does V7 Go support external models?
V7 Go supports a variety of foundation models through our platform, and you can also connect your own API keys to use models of your choice. This flexible approach allows you to select the best AI models for your specific workflows.
+
Can V7 Go recognize printed and handwritten text?
V7 Go is capable of recognizing both printed and handwritten text, leveraging advanced optical character recognition (OCR) technologies, as well as charts, diagrams and logos.
+
Why should I use Go instead of calling a model provider directly?
Go is more accurate and robust than calling a model provider directly. By breaking down complex tasks into reasoning steps with Index Knowledge, Go enables LLMs to query your data more accurately than an out of the box API call. Combining this with conditional logic, which can route high sensitivity data to a human review, Go builds robustness into your AI powered workflows.
+
Is V7 Go part of V7 Darwin?
No, they are separate products with different purposes. V7 Darwin is a data labeling platform for annotating images, videos and medical imaging to train your own models. V7 Go specializes in applying foundation models to automate document-intensive workflows through intelligent processing.
+
Does V7 Go support external models?
V7 Go supports a variety of foundation models through our platform, and you can also connect your own API keys to use models of your choice. This flexible approach allows you to select the best AI models for your specific workflows.
+
Can V7 Go recognize printed and handwritten text?
V7 Go is capable of recognizing both printed and handwritten text, leveraging advanced optical character recognition (OCR) technologies, as well as charts, diagrams and logos.
+