Read why V7 joined iToBoS (Intelligent Total Body Scanner) research project funded by European Union's Horizon 2020 research and innovation program.
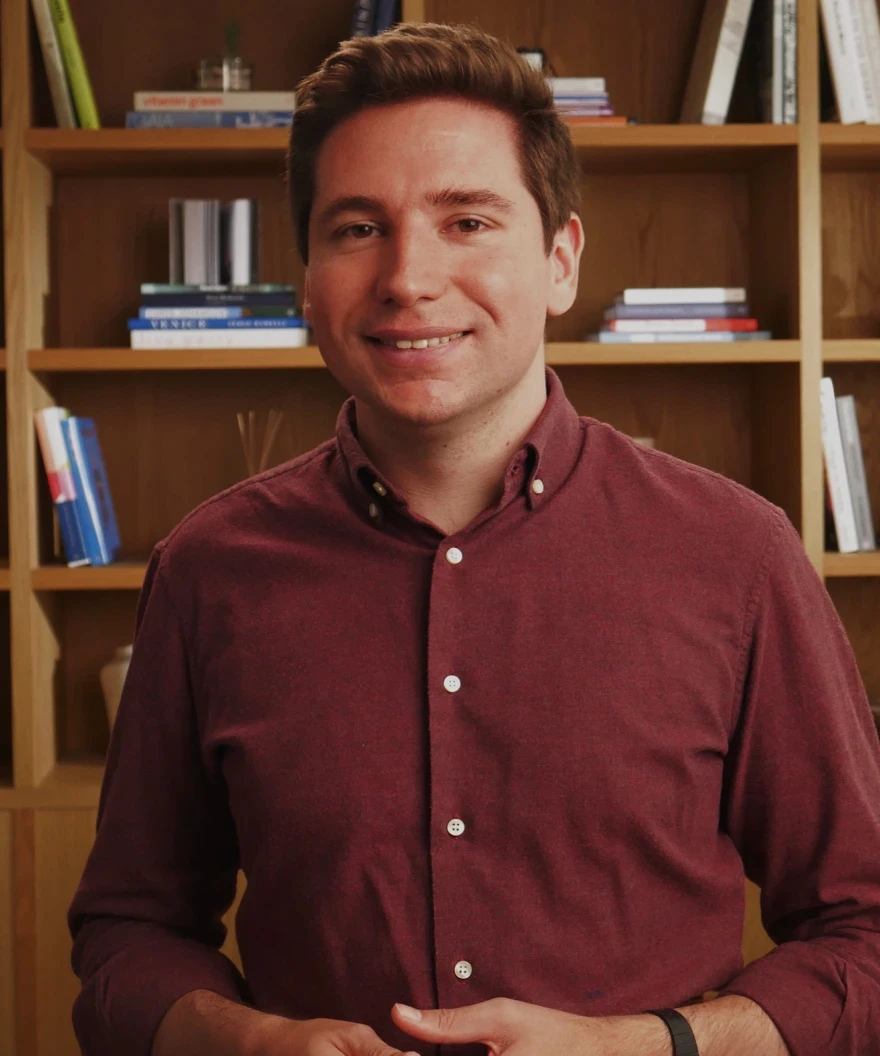
Co-founder & CEO of V7
Fortunately, melanoma may be cured if treated at an early stage.
Mortality increases with the melanoma’s growth into the skin. More than 90% of melanoma patients are still alive after 5 years if treated early. However, if a distant spread of cancer cells occurs (the so-called, metastatic melanoma), the proportion of patients alive after 5 years may be as low as 30%, or even lower.
For these reasons, quick diagnosis is essential to make sure treatment starts before local and metastatic spreading occurs.
About iTobos
iToBoS (short for Intelligent Total Body Scanner) is a research project funded by the European Union's Horizon 2020 research and innovation program.
It aims to build a new diagnostic tool for the early detection of melanoma, utilizing all the available information about the patient. This holistic assessment tool is to understand the individual characteristics of every patient to enable a personalized, early detection of melanoma.
The project lasts for 48 months (April 1, 2021–March 31, 2025) and has a total budget of EUR 12 million. As many as 19 organizations and institutions have partnered up to bring the project to life and they’re led by the University of Girona (Spain).
Here’s a full list of participants:
University of Girona (Spain)
V7 LTD (United Kingdom)
Optotune Switzerland AG (Switzerland)
IBM Israel-Science and technology Ltd (Israel)
Robert Bosch España Fábrica Madrid SA (Spain)
Barco NV (Belgium)
National Technical University of Athens-NTUA (Greece)
Gottfried Wilhelm Leibniz Universitaet Hannover (Germany)
Fundació Clinic per a la Recerca Biomédica (Spain)
Spain IT Services SLU (Spain)
Trilateral Research Limited (Ireland)
Universita degli Studi di Trieste (Italy)
Coronis Computing SL (Spain)
Torus Actions (France)
ISAHIT (France)
The University of Queensland (Australia)
Szamitastechnikai es Automatizalasi Kutatointezet (Hungary)
Fraunhofer Gesellschaft zur Foerderung der Angewandten Forschung E.V. (Germany)
Melanoma Patient Network Europe (Sweden)
What will the iToBoS experience look like?
A patient will enter a room and lay on a bed. The scanner above them will move to acquire images of their body surface, and that’s it! The scanner will look like a series of cameras in an arch that moves.
The iTobos plans to run its first trials with one-half of at-risk patients in order to collect data on a balanced set of healthy and at-risk patients.
The early users will lie comfortably on a bed, switching sides after the first scan is completed within a few minutes.
The patient’s personally identifiable features are excluded from the photography, and a mole-map of the patient is generated with the help of a sole technician.
The Difference
In contrast to previous technologies for mapping the surface of human skin for mole classification, iToBoS will photograph each mole at a higher resolution, and use additional information to determine the level of melanoma risk for each lesion.
The goal is to devise to a machine that captures the same image resolution as a dermatoscope that dermatologists are used to analyzing.
But doing so automatically through the robotic movement of cameras, and utilizing AI to identify and crop each lesion across the body. All this while anonymizing all patient’s identifiable features.
The AI Challenge
Melanoma detection is hard, and spotting it early can save lives.
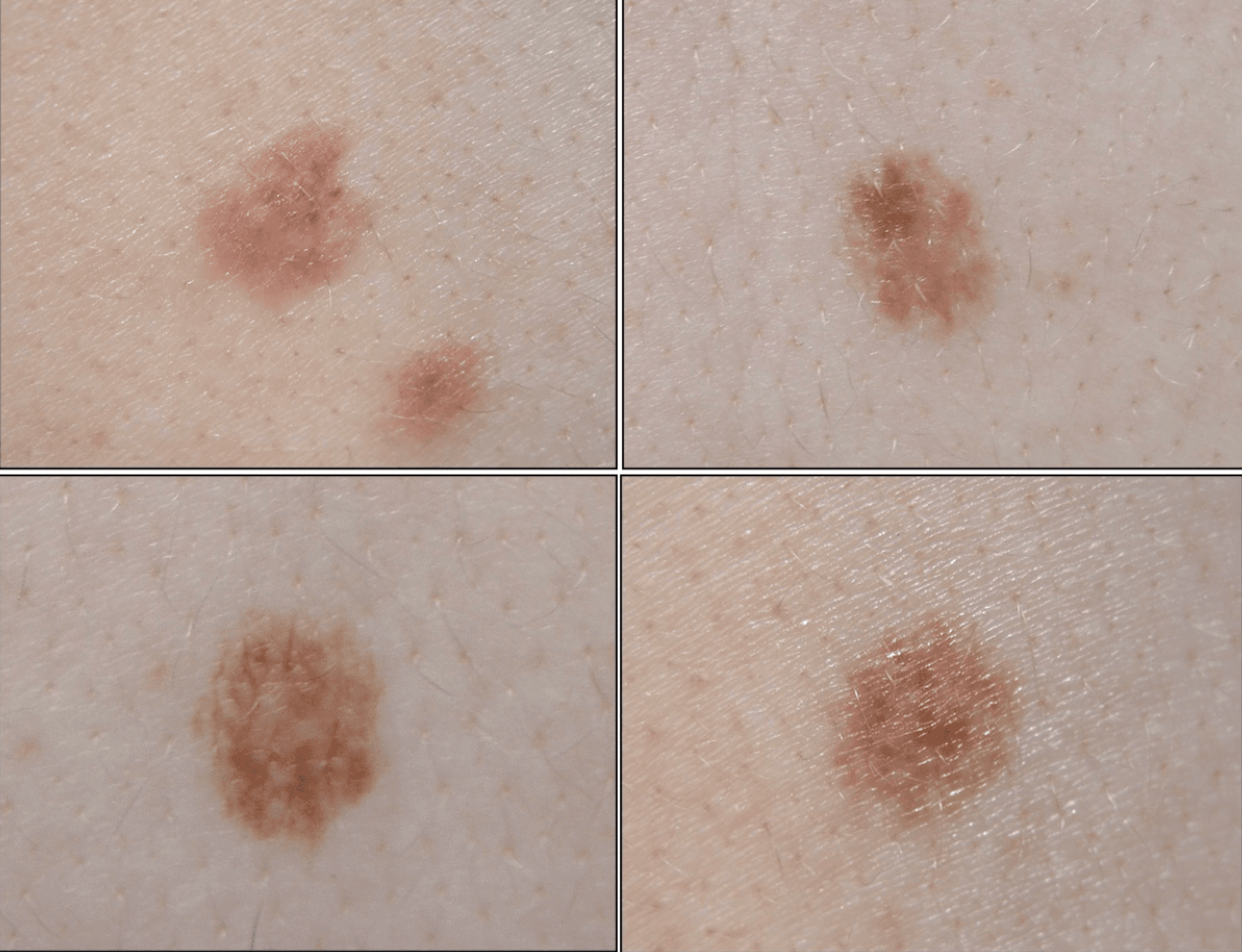
Four moles (melanocytic lesions) with similar appearances. Only the upper-left lesion is malignant melanoma, whilst the other are benign moles. Image credit: Professor Josep Malvehy. Researcher of the Dermatology Department at the Hospital Clinic of Barcelona. iTOBOS consortium.
Consider the image above. The first two moles are merely atypical melanocytic lesions at the dermoscopic level.
But the other two can kill you.
And spotting the difference isn’t that obvious. Even to an expert, the varying appearance of skin lesions across age groups and ethnicities may prove quite challenging. What could look suspicious on an 35-year-old Asian, could look normal on a 50-year-old Southern European.
The advantage AI can bring is that of condensing the world’s dermatological knowledge into a piece of software. This knowledge is referred to as training data, and is composed of annotated images that represent a doctor’s opinion on the mole.
The annotations can take the form of a shape encompassing the boundaries of the mole to train computer vision algorithms, a reference to the histopathology scan, and tags representing its appearance or level of risk.
AI feeds all the information into neural networks. The neural networks can then compare any new case against the training data and see if the new case exhibits similarities to any example the network has seen in the past.
The importance of Accurate AI Training Data
Curating the training data for any medical application is no small feat—
Present too many benign lesions, and the AI will become too biased towards them. Tag your demographics improperly, and the AI may ignore information that it cannot otherwise see or investigate, such as the age or ethnicity of the patient.
V7’s technology is used to apply tags to every patient and lesion to accurately represent both demographics and 16 lesion types.
Populations that go for mole scans are very imbalanced—with people from sunny parts of the world visiting dermatologists more frequently.
This is why it’s crucial to ensure that the technology is trained on representative samples of people.
To avoid other forms of bias, V7’s AI will semi-automatically spot every mole across the 3,000 images captured by iToBoS per patient, generate a cropped version, and human labelers will ensure that the segmentation around each mole is perfect and doesn’t include “distractors” such as items of clothing, tattoos, or jewelry that could bias the AI towards the wrong result (in machine learning this is known as an adversarial example).
An example of the V7 interface for the iTobos project displaying a number of segmented moles.
As AI models within iTobos are trained by data scientists within the consortium, V7’s software will use a special workflow to process every detected mole across multiple neural networks. The scientist observations will be combined with dermatologists’ opinions whenever a mole gets flagged as suspicious. These dermatologists will also have access to the previous version of the mole if available.
This way, skin lesions will be semi-automatically segmented by human expert labelers leveraging AI models. To apply supervised learning to the problem, it’s necessary to see enough balanced data that allows to catch the typical features of a malignant lesion across populations.
The deep learning revolution taught us that having computing power is not enough. What we need are curated datasets to start learning effectively.