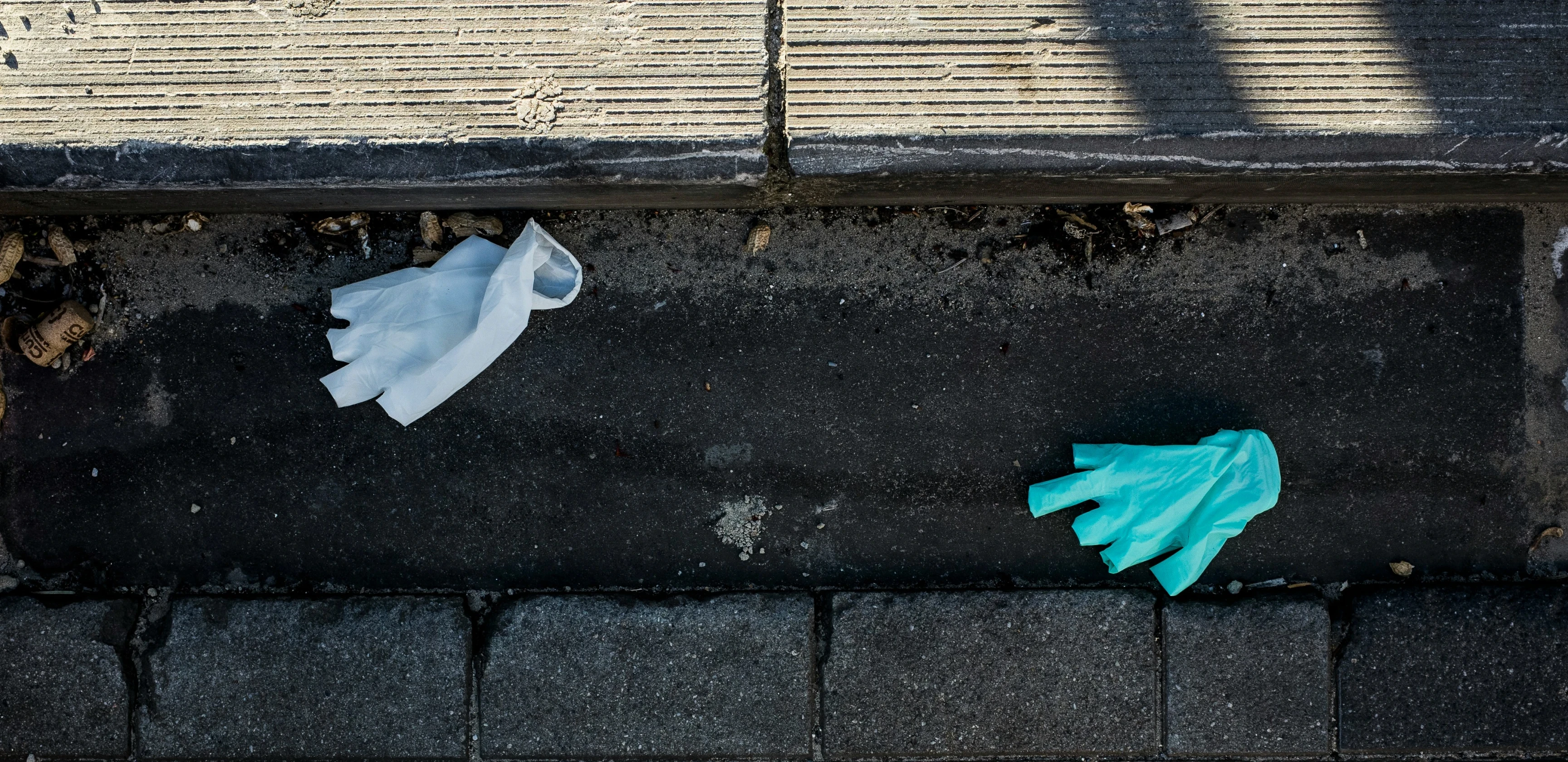
Developing innovative manufacturing processes
Industry
Energy
Use Case
Autonomous Waste Sorting
Company size
800 employees
Headquarters
Coventry, UK
Data labelling
Discover our solutions
About
The Manufacturing Technology Centre (MTC) was established in 2010 as an independent Research & Technology Organisation (RTO) to bridge the gap between academia and the tech industry.
MTC focuses on innovation research—particularly in the manufacturing domain. In 2020, they took part in the ‘Sort and Seg’ innovation competition and partnered up with Atkins,CyanTec (robotics), PSC (nuclear measurements services), and other partners to work with the Nuclear Decommissioning Authority (NDA) on AI solutions for sorting and segregating mixed radioactive wastes at the UK’s oldest nuclear sites.
The challenge
Currently, there’s no reliable solution for sorting stored waste from the decommissioning of nuclear facilities due to the dangers of handling it. MTC teamed up with Atkins and set on the mission to develop a computer vision-powered grasp planning software (robot) for autonomous waste sorting.
The solution
MTC integrated a cloud-based segmentation model using V7 to identify key object types and separate objects of interest in the scene. MTC developed a grasp planning pipeline to merge view and segmentation data and extract grasps for robots for both parallel and vacuum grippers.
The results
Consistency between different labelers was the biggest challenge the team faced—different labels for different objects mean poor results, and manually checking for consistency is tiresome.
However, MTC took advantage of V7’s advanced quality review capabilities and built an efficient data workflow enabling their team to easily spot and correct inconsistencies and errors in their labeling.
Right now, their newly labeled data is added to the dataset so that they can re-train the model and soon test it in the real-scenario environment.
Previously, MTC labeled their data using VGG, but with V7, they could speed up their annotation process by 10x and improve their accuracy thanks to the robust QA process and customizable workflows.
Training data needs
Since the team does not yet have access to the actual site, the project's end goal is to demonstrate how a robot can pick, classify and sort debris using data trained in V7, in a showcase situation which can then be replicated in live environments.
The MTC team began their work by setting up a camera system that captures images of waste distributed between low to intermediate-level radiation levels, with a sample dataset consisting of rubble, pieces of metal, rubber, and batteries—simulating the remnants of decommissioned nuclear facilities.
MTC uses semantic segmentation with polygons to label their data on V7 and train robust models used to then reconstruct 3D scenes from multiple views and target specific objects to be picked up.
The training dataset has less than 600 images. The team collected more than 1000 images, and some of them have up to 12 objects in one image.
Why V7?
MTC found out about V7 through word of mouth and decided to replace their open-source tool with V7 after reviewing more than 10 different providers.
The team is labeling their data in-house, and V7 appealed to them as a very intuitive, easy-to-use platform for non-technical people involved in the project. They were delighted to learn about V7’s customizable workflows, which became their favorite feature as it allowed them to get new images labeled using a pre-trained model.
Additionally, handy tools such as a brush tool and auto-annotate allowed them to label data faster and make necessary changes to achieve pixel-perfect segmentation masks.